AI Data Catalog: Exploring the Possibilities That Artificial Intelligence Brings to Your Metadata Applications & Data Interactions
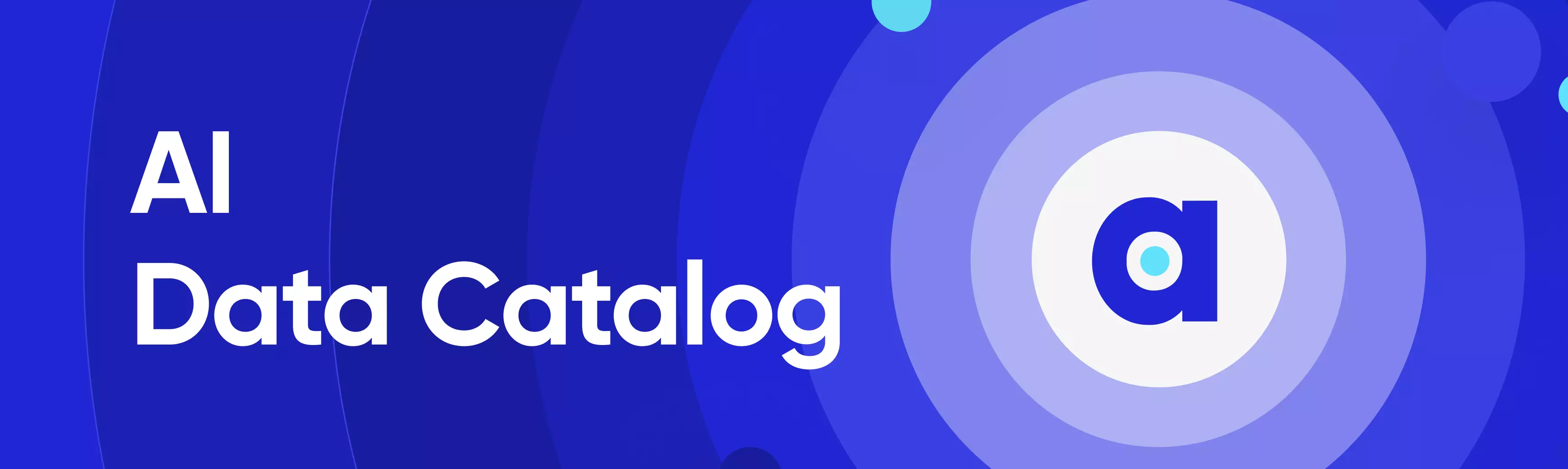
Share this article
An AI data catalog scours your data estate for metadata and then processes it to automate data workflows and offer intelligent recommendations to enrich data discovery, exploration, documentation, and governance.
With an AI data catalog, you can get all the data and context you need in seconds so that you can make better and more insightful decisions. Let’s understand the possibilities of AI in data cataloging, and then explore the business impact of AI data catalogs.
What is an AI data catalog? #
An AI data catalog is a modern data catalog that uses automation and intelligent recommendations to crawl, collect, and process metadata, optimizing data documentation, search, discovery, and exploration. It has the ability to draw context from an asset’s metadata to help data practitioners think more, work less and be more efficient.
According to Gartner, AI data catalogs “automate various tedious tasks involved in data cataloging, including metadata discovery, ingestion, translation, enrichment, and the creation of semantic relationships between metadata.”
For a data catalog to be considered an AI data catalog, it should:
- Offer automatic suggestions for data documentation — business glossaries, data asset descriptions, READMEs
- Suggest questions you can ask about data
- Autocomplete and write SQL queries, enhance existing query scripts, and fix bugs, so that everyone can explore data sets
- Support natural language search across your data estate
- Recommend similar assets when you search for data
- Automatically suggest and update data tags so that you can classify data easily at scale
- Run automated quality checks and alert the right people whenever there’s an issue with an asset or a pipeline
The world of AI is constantly evolving and breakthroughs in innovation are being made every day. For example, Microsoft recently invested $10 billion in OpenAI, GitHub launched a pair programming AI called Copilot, and text and image processing chatbot GPT-4 is now available. These incredible advancements in AI have opened up new possibilities and have shown the potential of AI in transforming and revolutionizing approaches to various workflows.
So, the possibilities of how AI can transform your data cataloging experience are also endless.
Why do you need to care about your data catalog having AI capabilities? #
An AI data catalog can help data practitioners be more productive and efficient with their daily workflows.
How Is AI Impacting The Development of Next-Gen Metadata And What Does It Mean For Data Teams? - Source: Data Cloud Now on Youtube.
According to Gartner, “AI can assist with data preparation, insight generation, and insight explanation. It supports the expert and citizen data scientists by automating many aspects of data science, machine learning, and AI model development, management, and deployment.”
Here’s how Forbes emphasizes the possibilities of AI in cataloging. With AI data catalogs, CMOs can ask questions like “What is the return on our advertising spend in both print and digital, over the last eighteen months for our newest product line?”
Without AI data catalogs, even identifying the data points necessary to answer these questions required the involvement of IT, who —backlogged with other priorities — might get back with the answer in a few weeks. With modern data catalogs powered by AI, users can look for and find the answers to such questions by themselves, within minutes.
Read more on how AI can power data catalog workflows — can save time, improve efficiency, and extract value from data at scale.
What is the business impact of AI data catalogs? #
AI is already changing the way we work, and it’s easy to imagine the possibilities it can bring to our data interactions and experiences. As we get excited, let’s consider how we can use AI data catalogs to drive business outcomes.
- Save costs with faster and more efficient data discovery
- Uncover new opportunities at scale to drive revenue growth
- Work less with automation and intelligent recommendations for data documentation
- Reduce data chaos by ensuring data consistency across all applications
- Reduce time-to-insight with no-code data exploration
- Improve data security, privacy, and regulatory compliance to avoid costly fines and build trust
Let’s see how.
1. Save costs with faster and more efficient data discovery #
AI data catalogs can scour metadata across your entire data estate to increase the accuracy and relevance of your data search and discovery efforts.
Data practitioners may spend hours searching for the data they want — an average employee spends 3.6 hours daily searching for information. IT teams spend half their day (4.2 hours) looking for relevant information to support business user requests.
With an AI data catalog, data practitioners can reduce the effort required to find the right data. They can ask questions about data using a Google-like search interface and get lightning-fast search results.
That’s at least 3 hours saved per day, resulting in significant time and cost savings.
2. Uncover new opportunities at scale to drive revenue growth #
Data practitioners can discover new opportunities at scale as AI data catalogs can analyze and interpret metadata across your data estate.
So, whenever you look for a certain asset, AI will also offer suggestions on similar data assets, so that you can understand data asset relationships better. Think of it along the lines of the “People also ask” and “Searches related to…” sections on Google search.
Without AI, making such connections might have taken days. In some cases, you might completely miss them — “unknown unknowns”, i.e., things you’re not even aware you don’t know.
HBR explains the “unknown unknowns” with this case study:
“GNS Healthcare applies machine-learning software to find overlooked relationships among data in patients’ health records and elsewhere. After identifying a relationship, the software churns out numerous hypotheses to explain it and then suggests which of those are the most likely. So, GNS uncovered a new drug interaction hidden in unstructured patient notes.”
How can AI data catalogs help do something similar?
AI data catalogs can study metadata on customer behavior to make connections and offer insights. So, for instance, if you search for the purchase history of a certain customer, an AI data catalog might recommend reviewing data on the same customer’s service requests or feedback offered.
3. Work less with automation and intelligent recommendations for data documentation #
AI data catalogs can study metadata from related assets to offer automatic suggestions for data descriptions, glossaries, READMEs, and more. Data practitioners can then choose to accept, modify, or reject these suggestions.
This process could take hours and lead to inconsistencies. For example, different teams can interpret “revenue” in different ways:
- The sales team may consider revenue to be the amount of money received from customers
- The marketing team may interpret revenue as the money generated from marketing campaigns
- The finance team’s interpretation could be the amount after deducting cancellations or refunds
- The C-suite may interpret revenue as the overall financial health of their organization
Standardizing such terms and ensuring that all teams are aligned is crucial to make sure that everyone’s on the same page.
AI can crawl metadata from similar data assets to auto-populate data descriptions and definitions to avoid such conundrums altogether. So, data practitioners spend less time and effort manually documenting data and rely on intelligent recommendations to document at scale.
4. Reduce data chaos by ensuring data consistency across all applications #
AI data catalogs, especially the ones powered by active metadata, also enable a two-way flow of metadata. As mentioned earlier, AI can speed up data documentation with intelligent suggestions.
Data practitioners can also perform bulk updates on data descriptions, certifications, owners, classifications, status, and other such metadata across all applications. So, your entire data estate is in sync and everyone has access to consistent and fresh data.
5. Reduce time-to-insight with no-code data exploration #
AI data catalogs can help business users write SQL queries and understand existing scripts with English prompts. AI can also review code, find errors, and offer suggestions on fixing them so that you don’t have to rely on IT to query data.
Without AI, this process involves submitting a request to IT or engineering. They may take hours, even days, to get back.
Even after they get back, the results might not be what you needed as business and IT teams query data with a different lens. While business users try to connect insights from data with project metrics directly affecting the overall business, IT might not have that context.
Forbes summarises this problem on how business and IT teams see data:
“Executives approach business questions through the filter of strategy, sales growth, target markets, competitive threats, the customer experience, and company mission. IT professionals see the world through a vastly different lens.”
AI empowers business users to explore data by themselves, which also frees up the time IT or a central data team has to spend supporting business requests so that they can focus on data quality, security, and availability.
6. Improve data security, privacy, and regulatory compliance to avoid costly fines and build trust #
AI data catalogs can help you automatically tag data and propagate it via lineage, based on the labeling for similar data assets. So, if you’ve identified patient records as PII, then an AI data catalog would compile related assets and recommend tagging them as PII too.
The catalog would also ensure that the right data encryption, masking, and anonymization policies are applied to the PII assets by tracking an asset’s journey through various workflows. This helps you avoid compliance issues and ensure data privacy and integrity.
Just like classification and encryption, an AI data catalog can also propagate access control policies via lineage. Moreover, it can offer suggestions on people who can own or modify data assets by studying metadata from similar assets. This helps with monitoring data access and security.
With AI data catalogs, you can reduce the costs associated with data errors, compliance issues, and inefficient workflows.
Moreover, since data is properly classified, labeled, and tracked throughout its lifecycle, you know where the data came from, how it has been processed, and who has had access to it. This builds trust in the reliability and quality of data.
Here’s a peek into what an AI data catalog can do
Snippet from the demo of AI capabilities of Atlan data catalog. Video by: Atlan
Summing up #
AI in data cataloging is akin to the iPhone moment for the telecom industry. From data discovery to exploration, AI can power numerous use cases, automate recurring tasks, and take data teams a step closer to 100% self-serve analytics.
Think of an AI data catalog as an always-on, intelligent workspace that acts as the context, control, collaboration, and action plane for your data estate.
AI data catalogs can free up data practitioners to spend less time doing grunt work and more time-solving problems that can drive revenue growth, discover business opportunities and improve efficiency.
Ready to get your hands on AI data catalogs? Sign up to Learn More.
Share this article