Data Dictionary: Examples, Templates, Best Practices, and How To Make a Data Dictionary
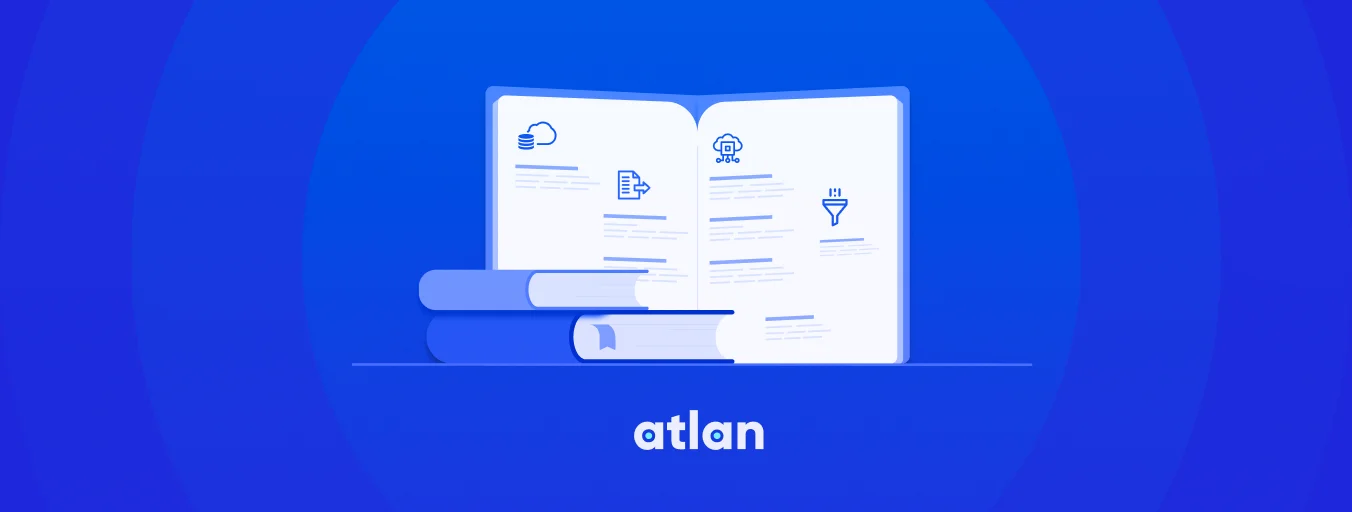
Share this article
Quick answer:
Pressed for time? We’ve got you covered with a 2-minute summary of the highlights of this article:
- A data dictionary includes technical metadata such as data object names and definitions, properties, business rules for validation, reference data, missing data, and more.
- The article looks into the components of a data dictionary, its benefits, setup process, and best practices. We also explore the differences between a data dictionary, a business glossary, and a data catalog.
- Looking for a way to build a modern data dictionary? Make sure to check out Atlan — a modern data catalog that hosts beyond a standard data dictionary. Book a demo or take a guided product tour.
What is a data dictionary? A data dictionary can be defined as a collection of metadata such as object name, data type, size, classification, and relationships with other data assets. A data dictionary acts as a reference guide on a dataset.
80% of a data scientist’s valuable time is spent simply finding, cleaning, and organizing data, leaving only 20% to perform analysis, according to HBR.
That’s where a repository of all data assets — column descriptions, metrics, measurement units, and more — can help. That is the purpose of the data dictionary.
Here, we’ll explore the fundamentals of a data dictionary, its examples, templates, best practices, and an action plan to build it; plus an understanding of tools that can help.
Table of contents #
- What is a data dictionary?
- What is an enterprise data dictionary?
- Business glossary vs. data dictionary
- Data catalog vs. data dictionary
- Components of a data dictionary
- Examples of data dictionary
- 4 Key benefits of a data dictionary
- How to create a data dictionary?
- Best practices for describing variables in a data dictionary
- Data dictionary: Summing up
What is a data dictionary? #
A data dictionary is a collection of metadata such as object name, data type, size, classification, and relationships with other data assets. Think of it as a list along with a description of tables, fields, and columns. The primary goal of a data dictionary is to help data teams understand data assets.
According to IBM’s Computer Terminology Dictionary,
a data dictionary is a “centralized repository of information about data such as meaning, relationships to other data, origin, usage, and format. It assists management, database administrators, system analysts, and application programmers in planning, controlling, and evaluating the collection, storage, and use of data.”
Meanwhile, DAMA UK (Data Management Association, UK chapter) defines
a data dictionary as “software in which metadata is stored, manipulated, and defined.”
What is a data dictionary used for? #
A data dictionary is used by data administrators, analysts, and engineers to understand and trust data assets. It helps in the creation of authentic, transparent, and consistent data throughout the organization.
Where do data dictionaries fit in your stack? #
According to data governance coach Nicola Askham, you can have multiple data dictionaries as it has details of the systems hosting or holding data assets. So, each data source — a warehouse, lake, or lakehouse — will have a data dictionary.
What is an enterprise data dictionary? #
An enterprise data dictionary is a compilation of metadata such as object name, data type, size, classification, and relationships with other data assets. It can also include business metadata such as the definition, associated business terminology, and metrics. The goal of an enterprise data dictionary is to help business teams understand and use a data set easily.
According to a veteran technical business analyst, the enterprise data dictionary is “the key for any company looking to connect the dots for all users.”
Learn more → 6 types of metadata
While exploring the concept of a data dictionary, you’ll come across other terms such as data catalog, data glossary, and business glossary. So, let’s look into the differences between these terms before delving into the components of a data dictionary.
Business glossary vs. data dictionary: What’s the difference? #
A business glossary (also known as a data glossary) covers the business terminology or concepts for an entire organization. The goal is to define a common vocabulary of terms for an enterprise.
The glossary includes a more descriptive name and detailed description of each term, along with possible aliases. In some cases, it also covers specific business rules for defining a term.
Unlike the data dictionary, there can only be one business glossary for an entire organization. Think of it as a common language or a way to talk about the data consistently in an organization.
The business glossary is considered to be a prerequisite for any data governance program and should be available before you start building a data dictionary.
Learn more → Data dictionary vs. business glossary
A business glossary is a centralized repository of business terms, KPIs, metrics, and definitions. Image by Atlan.
Data catalog vs. data dictionary: What’s the difference? #
A data catalog handles the indexing, inventorying, and classification of data assets across multiple data sources in an organization. Modern data catalogs offer rich context on data by crawling data dictionaries and the business glossary for technical, business, and operational metadata.
Crawling all kinds of metadata also helps data catalogs visualize data flow and its lifecycle — the origins, transformations, and upstream and downstream dependencies. Think of it as a platform that tells you the story of each data set.
Additionally, data catalogs also serve as the workspace for collaboration on data.
Both data dictionaries and the business glossary are considered to be integral parts of the modern data catalog.
Learn more → Data catalog vs. data dictionary
A data catalog helps users search, discover, understand, and trust data assets in an organization. Image by Atlan.
A Guide to Building a Business Case for a Data Catalog
Download free ebook
Components of a data dictionary #
According to the USGS (US Geological Survey), a data dictionary can include:
- A listing of data objects (names and definitions)
- Detailed properties of data elements (data type, size, nullability, optionality, indexes)
- Entity-relationship (ER) and other system-level diagrams
- Reference data (classification and descriptive domains)
- Missing data and quality-indicator codes
- Business rules, such as for validation of a schema or data quality
Additionally, the data dictionary should also include information on:
- Data source (data warehouse, data lakes, databases, applications)
- Date and time when the property was created or changed
- Descriptive statistics that go beyond missing values, such as min-max values and histogram distribution
- Owners and editors of data sets that contain these variables
- SQL queries attached to the data asset
- Social metadata associated with each data asset - stored as tags, notes, and chat transcripts
Now, let’s look at some examples of data dictionaries.
Examples of a data dictionary #
The data dictionary can be a simple table maintained using a spreadsheet, PDF, or a full-fledged web application. Let’s look at some data dictionary examples.
1. A simple, tabular data dictionary for human health risk assessment #
A good example of a data dictionary is the one used by ORNL (Oak Ridge National Laboratory).
ORNL maintains this dictionary as a PDF and it resembles a detailed index at the end of a book. The document provides basic information (entry type and description) on each entry, called a variable.
What does a data dictionary include? Example from The Human Health Risk Assessment Data Dictionary. Source: ORNL.
2. A website for cataloging data dictionary terms, along with search capabilities #
The next example is from NASA’s PDS (Planetary Data System). The PDS data dictionary is a web page with a search bar and a listing of all the entries, called attributes. The website allows you to filter your search results in an effort to speed up your research process.
You can click on each attribute to understand it further. The details include technical metadata such as name, data type, the owner (i.e., Registered By), and identifiers for version, registration, authority, etc.
It also contains metrics and data quality indicators such as minimum and maximum values and the unit of measure. Any researcher can look up the terms they need using these dictionaries to make sense of their planetary data.
Example of what constitutes a data dictionary. The PDS Data Dictionary. Source: NASA’s Planetary Data System.
Data Catalog 3.0: The Modern Data Stack, Active Metadata, and DataOps
Download ebook
4 Key benefits of a data dictionary #
A data dictionary documents data assets with relevant context, making it easy to use, analyze, and discuss data across teams. The biggest benefits of using a data dictionary include:
- Detect anomalies quickly and avoid data inconsistencies
- Get more trustworthy data that’s easy to analyze
- Establish consistent processes to collect, document, and use data
- Build transparency and enable self-serve within data teams
1. Detect anomalies quickly and avoid data inconsistencies #
Modern data platforms automatically generate data quality metrics and statistics so that you can understand the quality of your data at a glance.
Since the data dictionary displays descriptive statistics — minimum, maximum, count, frequency, mean, and median — spotting anomalies in data becomes easy. This helps you avoid inaccuracies or inconsistencies in data.
2. Get more trustworthy data that’s easy to analyze #
As mentioned earlier, a data dictionary offers context by documenting metadata as well as data sources/origins, owners, creation dates, and so on. This helps you validate each data set and make sure the information you have is reliable, which makes your decision-making more accurate.
Additionally, modern data platforms such as a data catalog also let you visualize the overall data flow, making it easier to interpret your transformations’ impact on upstream or downstream applications.
Whenever you can’t verify the credibility of a data set, modern data dictionaries let you discuss that data and share it (with just a link or via Slack) with the right people.
Visualize the complete journey of your data asset from source to BI tools. Source: Atlan
3. Establish consistent processes to collect, document, and use data #
If done right, a data dictionary can help you establish certain ground rules for collecting, documenting, and using data. This, in turn, simplifies regulatory compliance.
Since the data dictionary contains all the technical metadata, you can spot which teams or business units aren’t managing their data assets properly and fix those bad data practices.
4. Build transparency & enable self-serve within data teams #
As mentioned earlier, the data dictionary equips everyone in your organization with a common repository for data definitions, standards, metrics, and more.
So, everyone understands what any element within a data set means without having to consult an expert. This reduces dependencies, helps everyone use the data in the same way, and makes onboarding a breeze.
Learn more → The benefits of a data dictionary
How to create a data dictionary? #
The purpose of a dictionary is to help you avoid asking questions such as “what does this variable name mean?” or “what is the ideal value for this field?”
How to create a data dictionary?
That’s why the OSF (Center for Open Science) recommends that your data dictionary should contain:
- Name, definition, and description of each variable
- Synonyms and associated variables
- Measurement units
- A range of accepted values, along with minimum and maximum values
5 questions to ask your data team before building a data dictionary #
To ensure that each variable contains the above information, you can ask your teams the following questions:
- What does each variable/element/field/attribute within a data set mean? What is it describing?
- Who collected your data? Are they still the owners, or is it somebody else?
- Who has interacted with your data, and what are the changes that they made?
- Who oversees the changes made to your data?
- How can you reach out to your data owners, admins, and editors?
- How did you collect each variable? How did you measure it?
- Do you include the raw value for the numeric values, or did you calculate it using a formula?
- What tests or checks did you run to determine whether your data is trustworthy?
Data Dictionary Demo - Atlan in 3 minutes: Future of Data Catalogs 🚀
3 Best practices for describing variables in a data dictionary #
The researchers at Smithsonian adopt the following best practices to define and describe attributes within the data dictionary:
- Start with basic information about the data
- Describe each component of the dataset
- Enable versioning
Their process is a good example of how good data teams document their data. Let’s explore this in detail.
1. Start with basic information about the data #
Each data dictionary should offer basic information about the dataset. This should include:
- Creator or owner of the data dictionary
- Title
- Date of publishing
- The metrics or descriptive statistics
- The purpose of data collection
- Methodologies used or assumptions made while collecting data
The Smithsonian recommends that you follow “the conventions of your discipline when choosing standardized terms or when structuring your data.” This practice also comes in handy with compliance audits.
2. Describe each component of the dataset #
You should provide a complete definition for each component of the dataset. Next, offer a description that contains the following information:
- Component name, which could be the data label, column header, or filename
- A full “human readable” name of the element if the dataset uses codes or abbreviations
- Any units of measure and precision (if applicable)
- The format of the data element (if applicable) — integer, text, date, or time
- If the component is numeric, include what values are valid or allowed
- Any codes, symbols, or abbreviations used in the values themselves
- The source of the data element — sensor, observation, or another application
- Include data validation or quality control processes, wherever applicable
3. Enable versioning #
Versioning the file lets you keep track of changes over time. The versioning is automatic if you use a web-based file system (or wiki) like Git or ArcGIS.
Make sure that your versioning includes descriptions of the changes made — the details of the editors, date and time, elements changed, and so on.
How to choose a data dictionary tool? #
Since several data dictionary tools (both enterprise and open-source) are available, we’ll focus on the capabilities you should look for in a tool.
To begin with, the data dictionary should define all technical terms from data tables or data models — for example, policy_expiration_date, and policy_id. Each of these terms should be linked to tables/dashboards so that data teams can find the information they need faster.
The data dictionary tool should also allow you to set up data definitions and descriptions as mentioned under the best practices listed above.
Additionally, a solid data dictionary for modern data teams should have the ability to:
- Group each element into categories — data sources or domains — to create multi-level hierarchies that mimic the way you work
- Search for each element as per its entry name in the data dictionary, rather than the actual column/table name
- List related elements, definitions, and metrics
- Crowdsource READMEs
- Format the dictionary easily — think of Notion-like commands
- Track all activity, along with proper explanations behind the changes made
- Send notifications/alerts whenever there are major changes to the entries in the dictionary
Data dictionary: Summing up #
Collecting vast amounts of data is only useful if you can interpret or analyze it. A data dictionary is like a README that documents everything you need to know about a dataset in order to use it for further analysis.
If you are looking to build a modern data dictionary, take Atlan for a spin. Atlan is more than a standard data dictionary. It’s a third-generation modern data catalog built on the framework of embedded collaboration, borrowing principles from GitHub, Figma, Slack, Notion, Superhuman, and other modern tools that are commonplace today.
Share this article