15 Essential Features of Data Catalogs To Look For in 2024
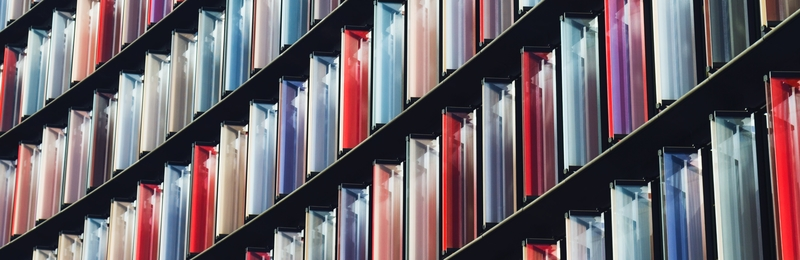
Share this article
Data catalogs should have some essential features that facilitate seamless data discovery, governance, lineage, collaboration, and automation across your organization’s data landscape.
15 Essential Data Catalog Features #
Here are 15 essential features of data catalogs that you should consider when evaluating solution providers:
- Out-of-the-box (OOTB) connectors to import metadata natively
- Automated lineage creation by mining lineage information using OOTB connectors
- Extensibility using APIs
- Programmatic lineage creation to ingest lineage from any tool using APIs
- SQL syntax and natural language search
- Browse and filter to customize data search and discovery
- 360° data profiles to build GitHub-like repositories for each data asset
- An active business glossary for better context
- Querying data without SQL knowledge
- Embedded collaboration to eliminate switching across apps
- Active data lineage to act on pipeline issues directly from the catalog
- Column-level lineage for end-to-end visibility
- Persona-based policies for personalized, granular access control
- Active metadata to set up an always-on, intelligent data ecosystem
- Intelligent automation that can be customized to perform various aspects of data management
Essential data catalog features - Image by Atlan
In addition, the data catalog platform should be customizable, self-service (even for business users), intelligent, and open to support all current and future data and analytics use cases.
Table of contents #
- 15 Essential data catalog features
- 1- Out-of-the-box (OOTB) connectors to import data natively
- 2- Automated lineage creation by mining lineage information using OOTB connectors
- 3- Extensibility using APIs
- 4- Programmatic lineage creation to ingest lineage from any tool using APIs
- 5- SQL syntax and natural language search
- 6- Browse and filter to customize data search and discovery
- 7- 360° data profiles to build GitHub-like repositories for each data asset
- 8- Active business glossary for better context
- 9- Querying data without SQL knowledge
- 10- Embedded collaboration to eliminate switching across apps
- 11- Active data lineage to act on pipeline issues directly from the catalog
- 12- Column-level lineage for end-to-end visibility
- 13- Persona-based policies for personalized, granular access control
- 14- Active metadata to set up an always-on, intelligent data ecosystem
- 15- Intelligent automation that can be customized to perform various aspects of data management
- Summing up on data catalog features
- Data catalog features: Related reads
So, let’s explore each data catalog feature to wrap our heads around its significance to modern cataloging and data management.
1- Out-of-the-box (OOTB) connectors to import data natively #
Data is diverse, granular, and dynamic, and pours in through numerous applications in your tech stack. A top challenge for most data teams is bringing together all that data and making it ready to use at scale.
That’s why it’s important for data catalogs to offer integrations and support smooth interoperability with data tools. These integrations can either be out-of-the-box (OOTB) connectors or APIs.
Out-of-the-box (OOTB) connectors in data catalogs offer native integration with:
- Data sources such as Amazon Redshift, Google BigQuery, MySQL, Salesforce, and Snowflake
- BI tools such as Looker, Microsoft Power BI, and Tableau
- Data movement tools such as dbt Cloud and Fivetran
With native integrations, simply connect the source, authenticate the connection, specify what the cataloging platform should scan, and set up a crawl frequency to collect metadata.
2- Automated lineage creation by mining lineage information using OOTB connectors #
When the data catalog supports (OOTB) connectors, the lineage is also automated, down to a column level. For instance, if your data catalog connects natively with Snowflake, it will generate the lineage mapping as soon as you authenticate the connection and crawl Snowflake.
So, you can set up cross-system lineage by connecting everything from data warehouses to BI tools, and the data catalog would import lineage information automatically.
Automated lineage creation in data catalogs using OOTB connectors. Screenshot from Atlan
3- Extensibility using APIs #
As mentioned above, another way to ensure seamless integration with the rest of your modern data stack is via open APIs.
With open APIs, you can bring in metadata from any data product you want, from any source. The open API architecture lets you integrate lineage from your own home-grown tools, data sources like DynamoDB or S3 buckets, and orchestration suites like Apache Airflow or Dagster.
4- Programmatic lineage creation to ingest lineage from any tool using APIs #
Another issue that data teams suffer from is a lack of visibility of their data assets. According to Forrester, end-to-end visibility can help data engineers visualize existing and future data sources and integrations to support impact analysis, root cause analysis, bug fixes, and data policy compliance
That’s where an open API setup can help. With open APIs, you can generate lineage by bringing in any data product you want. For instance, you can build a workflow that connects HubSpot to Salesforce and integrates them with the data catalog to create a visual lineage mapping.
Programmatic lineage creation in data catalogs using APIs. Screenshot from Atlan
5- SQL syntax and natural language search #
A key data catalog feature is a keyword-based search. So. the data catalog should be equipped with a Google-like search engine that lets you search all data assets, look up SQL syntax, discover assets linked to business metrics, and more.
Just like Google, the search should also display related search results — synonyms, antonyms, connected reports, and more.
The data catalog interface should act like Google for your data universe. Screenshot from Atlan
This is crucial for finding the right data from the entire data landscape — a one-stop shop for data search and discovery.
6- Browse and filter to customize data search and discovery #
The ability to search for the right data significantly cuts down the time spent looking for data.
However, the ability to customize the search with metadata properties can help you filter through the results and zero in on the most relevant data quickly. These filters can include metadata, such as:
- Asset type
- Owner
- Classification type
- Last run status
- Certifications (Verified, Draft, Deprecated, or No certificate)
- Glossary terms
- Asset properties (Title, Description, Has Lineage, Created, or Last Updated)
- Usage
How metadata filters in a data catalog can create a personalized data shopping experience. Screenshot from Atlan
7- 360° data profiles to build GitHub-like repositories for each data asset #
360° data profiles act akin to a GitHub-like repository or a LinkedIn profile for each data asset. A data catalog should offer a masked preview of all data while hashing or redacting sensitive data.
Each profile should include:
- A comprehensive table summary
- README
- Query history
- Metrics
- Dashboards
- Activity log
- Native embeds with tools like Slack, Google Drive, Confluence docs, Jira, Looker, and GitHub
- Notion-like commands to format your content quickly
What 360° visibility would look like in a data catalog. Image by Atlan
You should also be able to share these profiles with GitHub-like URLs for each data asset, or via communication tools like Slack.
As a result, you can use one interface to get all the context about data — who owns a data set, where it comes from, how has it changed, and how to use it.
8- Active business glossary for better context #
A business glossary is your organization’s second brain that mimics your business domains and highlights how your data, definitions, and domains are connected. So, it would offer context on KPIs, metrics, business taxonomies, and more.
A key data catalog feature is an active business glossary. Screenshot from Atlan
An active business glossary goes a step further by linking related definitions, metrics, and assets to create a connected organization. You should also be able to compile all the tribal knowledge in your organization by adding details, such as owners, READMEs, documentation, and certification.
The glossary should also offer a glimpse into the journey of each term by also displaying version history, activity log, and announcements.
9- Querying data without SQL knowledge #
Querying data is essential to find the answers you need by merging different tables, adding or removing irrelevant data, or building catalog views for frequently searched data assets.
Traditionally, performing these actions required you to be familiar with SQL. However, modern data catalogs can help even non-technical (business) users run SQL queries with a low-code/no-code interface and intelligent automation.
That’s why a vital feature that any modern data catalog should offer is the ability to:
- Query data assets directly from the platform, either by writing your own SQL or by using the no-code, visual SQL builder
- Auto-suggest relevant columns/tables when writing a query
- Add custom variables in SQL
- Autocomplete queries with rich metadata context
- Create a collection of queries and schedule them to run at specific intervals
- Save queries and curate them in folders/collections
- Automatically link saved queries to tables and columns used in the query
- Switch the whole querying functionality on and off
10- Embedded collaboration to eliminate switching across apps #
An essential feature of data catalogs is embedded collaboration, which borrows principles from the modern tools that teams already use and love. It enables micro-flows powering the two-way movement of data — think of it as reverse ETL, but for data assets.
A data catalog with embedded collaboration doesn’t merely exist as a standalone tool. Instead, it weaves into the daily workflows of data teams seamlessly. So, you can discuss data assets, raise support tickets, link Slack threads, tag others, and share data assets with a link.
With a feature like embedded collaboration, you can stop switching between apps and instead use the data catalog to implement use cases such as:
- Requesting and getting access to data assets via a link
- Approving or rejecting access requests using your favorite collaboration tool
- Configuring data quality alerts on Slack so that your team can ask questions about a data asset and get context directly in Slack
- Triggering support requests on Jira without leaving the screen where you’re investigating a data asset
Democratize data analytics with collaboration. Screenshot from Atlan
11- Active data lineage to act on pipeline issues directly from the catalog #
In a platform that supports embedded collaboration, you also get active data lineage. That means you can use in-line actions — alerting downstream owners, raising support tickets for broken assets, or downloading all downstream tables for impact analysis — within the catalog.
Raising Jira tickets from the data catalog, thanks to active data lineage. Screenshot from Atlan
12- Column-level lineage for end-to-end visibility #
Data lineage captures how data flows across your data landscape and:
- Traces an asset’s origins to help with root cause analysis
- Traces an asset’s destinations to help with impact analysis
- Automatically propagates metadata to derived assets
- Enables end-to-end visibility to illustrate column-level relationships
End-to-end visibility of data asset relationships at a column level. Screenshot from Atlan
13- Persona-based policies for personalized, granular access control #
The humans of data are diverse — data analysts, engineers, consultants, and team managers. Each persona requires access to different assets with varying levels of permissions. For instance, an engineer would want to find assets with failed pipelines to fix a broken dashboard. Meanwhile, a consultant might want to check when the data on a certain dashboard was last updated.
That’s why one of the data catalog features must be persona-based access policies to cater to the preferences and needs of each user role.
Persona-based access policies in a data catalog. Image by Atlan
You should be able to customize the homepage of each user, display relevant metadata, and curate the right data assets. This helps in automatically applying personalized, granular policies to govern access and reduce the overall time-to-value.
14- Active metadata to set up an always-on, intelligent data ecosystem #
Active metadata helps you continuously analyze data, plus everything that happens to it or is done to it. A data catalog powered by active metadata would help you drive use cases, such as:
- Making data consumers aware of potential problems in advance by alerting them in real-time
- Removing duplicates and purging stale assets to cut costs and maintain a clean data landscape
- Automating quality control of data pipelines
- Continuously validating metric calculations to spot problems right away
- Creating custom relevance score to increase the reuse of popular data assets
- Supporting security and compliance reporting with the help of interrelated metadata
15- Intelligent automation that can be customized to perform various aspects of data management #
As the volume, veracity, and velocity of data grow, automating several aspects of finding, compiling, and inventorying data (irrespective of the type, format, or source) is vital to extract value from data quickly.
That’s where intelligent automation can help. For instance, an AI assistantcould offer intelligent recommendations to populate context—data asset descriptions, READMEs, and insights.
The AI assistant could also help you write SQL queries to run automated no-code transformations by writing the necessary prompts — no coding involved.
Some of the possibilities of intelligent automation include:
- Creating rules and filters to speed up documentation with auto-suggestions for asset descriptions, asset owners, linked terms, etc.
- Build rule-based playbooks to drive actions, such as automatically spotting unused assets, flagging risky or bad data and outliers, and propagating classifications across sensitive data assets
- Automatically assigning data owners to data sources
Here’s how Nasdaq accelerated key business users’ ability to access data by ramping up a modern data stack, which included deploying a data catalog tool
Summing up on data catalog features #
The Forrester Wave™ for DataOps, Q2 2022 highlights the importance of metadata and the role it plays in modern data catalogs by outlining three fundamental capabilities. A modern enterprise data catalog should:
- Address the diversity, granularity, and dynamic nature of data and metadata
- Generate deep transparency of the nature and path of data flow and delivery
- Deliver a UI/UX that reinforces modern DataOps and engineering best practices
Such a platform with the 15 features that we’ve covered above and the three fundamental capabilities outlined by Forrester will finally check all the right boxes:
- Facilitate data search and discovery
- Enable open knowledge-sharing and collaboration
- Build trust in data
- Ensure governance and regulatory compliance
- Enable data democratization without compromising data security and privacy
Interested in knowing how to find and implement such a catalog and drive business value? Take Atlan for a spin. Atlan is a third-generation modern data catalog built on the framework of embedded collaboration that is key in today’s modern workplace, borrowing principles from GitHub, Figma, Slack, Notion, Superhuman, and other modern tools that are commonplace today.
Data catalog features: Related reads #
- Data catalog benefits: 5 key reasons why you need one
- Modern data catalogs: 5 essential features and evaluation guide
- The ultimate guide to evaluating a data catalog
- Top data catalog use cases intrinsic to data-led enterprises
- What Is a Data Catalog? & Do You Need One?
- Best Alation Alternative: 5 Reasons Why Customers Choose Atlan
Share this article