Automated Data Governance: How Does It Help You Manage Access, Security & More at Scale?
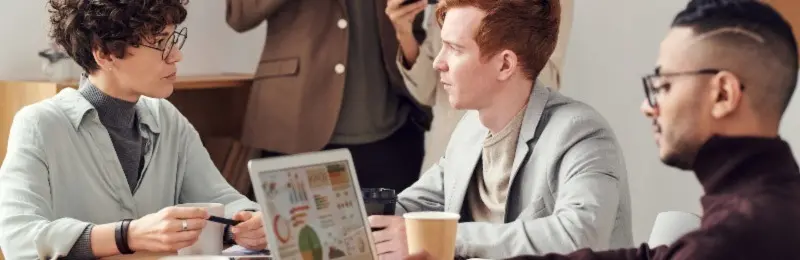
Share this article
Data is exploding: An estimated two quintillion bytes of data are generated each day. Given this scale and speed of data, automated data governance is increasingly necessary to ensure that users can find and use data that is relevant.
Here, we’ve covered the following aspects of automated data governance:
- What is automated data governance?
- Why do we need to automate data governance?
- Examples of automated data governance in play
- Data governance automation: Next steps
Historically, organizations have developed various mechanisms to fulfill the need for well-governed data, often manual processes overseen by a governance team. This has led to a branding problem where governance is seen as a controlling discipline that hinders more strategic work.
To effectively implement collaborative rather than controlling data governance programs - at scale, automation is key. By applying automated data governance, you can codify repetitive governance tasks to ensure they happen in a sustainable and error-free manner. Meanwhile, your data governance committee, data stewards, and departmental representatives are free to work together to build and implement the overall strategy.
What does automated data governance look like? Here, we’ll explore a high-level view of automated data governance and provide some use cases to help you understand how it can benefit your organization.
What is automated data governance? #
Automated data governance codifies the most repetitive governance tasks, replacing error-prone manual approaches with sustainable and reproducible processes. Along with building data lineage and ensuring policy compliance, automation can also be used in tasks like monitoring access to data assets, thus ensuring the right users can leverage data while keeping it secure.
According to a ResearchGate publication by a team of Spanish and Belgian scientists, "[The] large and heterogenous amount of data present in Big Data systems begs for the adoption of an automated data governance protocol, which we believe should include, but might not be limited to, the following elements:
- Data provenance, related to how any piece of data can be tracked to the sources to reproduce its computation for lineage analysis
- Measurement of data quality, providing metrics such as accuracy, completeness, soundness, and timeliness, among others
- Data liveliness, leveraging on conversational metadata which records when data are used and what outcome users experience from it
- Data cleaning, comprising a set of techniques to enhance data quality like standardization, deduplication, error localization or schema matching…”
Why do we need to automate data governance? #
Automation can be used to accomplish many tasks related to data governance. A few motivational factors for the adoption of automation include:
- The increasing volume and velocity of data
- The growing number of unique data sources in enterprises
- A heightened global awareness of cybersecurity, and a corresponding rise in privacy regulations
- The diversity of data producers and consumers
The increasing volume and velocity of data #
The total volume of enterprise data is expected to more than double over 2020-22, from approximately one petabyte to over 2 petabytes (Statista). Data governance needs an approach that can work with this kind of volume at scale.
In particular, tracking, managing, classifying, and enforcing policies through manual intervention is cumbersome and creates bottlenecks for individuals attempting to run analytics and gain data-based insights. All this becomes notably easier with automation in place to manage and streamline the details.
The growing number of unique data sources in growing enterprises #
A survey of North American organizations with over 1,000 employees found that the mean number of data sources per organization is 400 (Matillion and IDG Research). Finding and cataloging data assets resting in this growing number of sources requires data governance automation where possible, so users can locate and access relevant data quickly and efficiently.
A heightened global awareness of cybersecurity — and a corresponding rise in privacy regulations #
The exponential growth of data — particularly sensitive data that requires privacy measures — along with well-publicized data breaches impacting Fortune 500 enterprises and federal agencies, means countries are taking a closer look at privacy rights. An estimated 65% of the world’s population will have its personal data covered under modern privacy regulations by 2023.
Organizations need to ensure each query complies with these regulations, while not impeding workflows — an endeavor that is incredibly difficult to accomplish without the help of automation.
The diversity of data producers and consumers #
The modern data team includes data citizens in all departments and roles, from Arnold in Accounts Payable to Latasha in Legal. They may have questions as they work through data:
- Who owns a dataset?
- Has it been updated recently?
- What would happen if I corrected a piece of erroneous data?
- Has this data been validated by a business domain expert?
For example, a finance team member might have to contact salespeople every quarter to confirm their numbers are finalized. That process could be automated using a quality check that is tagged to the data asset. Automation can also be used to track and share information about data so users can understand the lineage and context associated with it.
Examples of automated data governance in play #
Putting automated data governance into practice requires evaluating specific areas where automation can help. Governance does not exist in a vacuum: instead, it works in tandem with other parts of the modern data stack, such as Snowflake databases, by providing tools that automate the management and use of data. Here are some examples.
Granular column-level access control #
Access controls are key to complying with organizational, industrial, and governmental regulations around privacy. By using granular access controls for users, groups, and teams, you can automatically grant or restrict access to databases, schemas, or even tag-based groups of data assets. This can be used to comply with privacy regulations around sensitive data, for example, by tagging any protected data and ensuring that only authorized users can access it.
Preview of access logs in Atlan
Auto-constructed data lineage #
The ability to trace data lineage is important, especially in tightly regulated industries like finance, where it can be used to demonstrate compliance, but using manual processes to track lineage is inefficient and error-prone. Auto-constructed data lineages can replace manual processes with SQL parsing that automatically understands and creates a visual representation of data lineage.
For example, if a business user wants to update a data set – but is concerned about the impact it may have on dashboards downstream, they can use the auto-constructed data lineage to understand how the data is used, without needing to contact the engineering team.
Visualize data lineage — both upstream and downstream. Source: Atlan
Auto-propagate policies through lineage #
Policies should propagate through lineage to ensure sensitive data can’t be loaded into a column or table with non-matching privileges. It’s important to have a way to automatically classify every table or column derived from a sensitive column so classification tags pass down through the lineage.
For example, a member of the sales department may want to use a column of regional sales data for a dashboard that will be presented externally. If that sales data contains personally identifiable information (PII), the dashboard will be automatically classified to prevent that information from being revealed to the public.
Auto-generated audit logs #
Audit logs are a powerful way to understand which users are accessing sensitive data, who has accessed a particular item, and broader patterns about data usage. Tracking this manually would be a tedious, error-prone effort. Hence, this is an ideal setting for automation which can be used to detect access and build audit logs in the background.
For example, suppose the marketing team builds a set of customer data and wants to understand how useful that set is so they can evaluate whether to continue maintaining it. Using auto-generated audit logs, they can see how many times users have accessed that set and develop a deeper understanding of which departments use it.
Data governance automation: Next steps #
It’s evident that scaling your data governance strategy requires some form of automation. Automated data governance enables you to embed governance activities in the daily workflows of data users. It also flips the narrative around data governance — that it’s about control — and ensures that governance fosters a practitioner-led data program, thus keeping up with the best practices for how data governance should manifest today.
Read this case study to learn about the data governance journey at Southeast Asia’s largest SME digital finance platform, which is advancing its data democratization efforts using automated data governance.
Implementing data governance programs is a monumental undertaking. That’s why a solid plan, impactful goals, relevant and real-time metrics, and an emphasis on constant communication and collaboration are essential data governance best practices to embrace.
Ready to make data governance effortless?
Try Atlan — Auto-construct data lineage and deploy best-in-class data access governance without compromising on data democratization.
Data governance automation: Related reads #
- Data governance and its importance in the modern data stack
- 6 commonly referenced data governance frameworks in 2023
- 8 best practices for a robust data governance program
- The 3 principles of data governance: pillars of a modern data culture
- Data governance policy: Examples, templates & how to write one
Share this article