Data Fabric Use Cases: Understanding Its Suitability & Applicability for Your Business
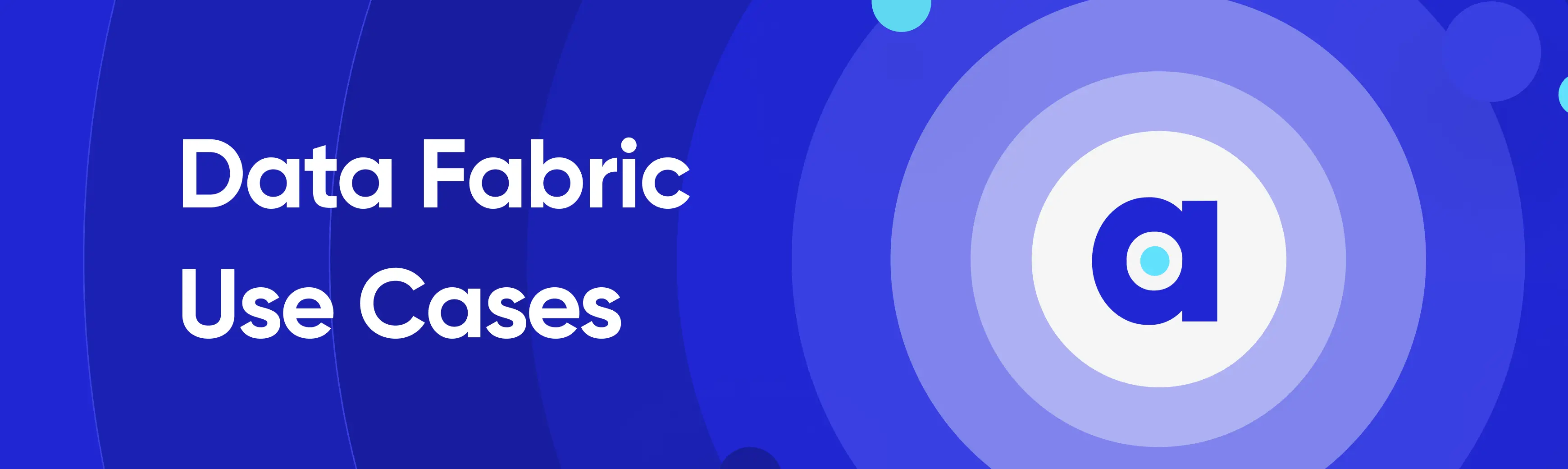
Share this article
A data fabric is used to create a unified, integrated layer of data across various sources and locations, making it easier for users to access, manage, and analyze data. It simplifies data management, improves data accessibility, and enables better data insights for decision-making.
A data fabric is a composable, flexible, and scalable way to maximize the value of data in an organization. It enables data management across cloud and on-premises environments, including data discovery, integration, orchestration, security, governance, and cataloging.
The key use-cases for data fabric typically involve simplifying and accelerating the process of data management and analysis. In this article, we will explore data fabric uses cases in greater detail.
Let’s dive in!
Table of contents #
- The top 5 data fabric use cases
- What is data fabric used for: Taking a closer look with case studies/examples
- When is a data fabric not ideal for you?
- Where to learn more about data fabric use cases?
- Rounding it all up
- Related reads
The top 5 data fabric use cases #
A data fabric addresses the challenges of managing and analyzing data that’s distributed across multiple systems and locations. It offers a unified, integrated data environment that simplifies data access, improves data quality, and accelerates data-driven insights.
Let us begin by looking at the biggest problems that data fabric solves with the help of a few use cases:
- Data discovery and governance
- Data integration and orchestration
- Data security and privacy
- Data quality
- Enabling real-time insights
Let us look at these data fabric use cases in brief:
1. Data discovery and governance #
Data fabric can help in the process of data discovery, metadata management, and maintaining data lineage, which is crucial for governance, compliance, and data understanding.
2. Data integration and orchestration #
Data fabric helps integrate data from different data sources, regardless of their format or location. It also allows for the orchestration of data pipelines across different systems and technologies.
3. Data security and privacy #
Data fabric provides a centralized place for managing data security and privacy. It can help apply consistent data masking, encryption, and anonymization policies across all data sources.
4. Data quality #
Data fabric can help ensure data quality through data profiling, standardization, matching, and consolidation.
5. Enabling real-time insights #
Data fabric can help enable real-time analytics and decision-making by facilitating real-time data ingestion, processing, and analysis.
Why data fabric could be an excellent approach to consider? #
Here’s why many organizations adopt a data fabric:
- Reduces load on OLTP database
You can keep your operational OLTP database intact, but you can offload analytical queries to the data fabric, which can connect to and integrate data from the OLTP database as well as other data sources. This will help reduce the load on the OLTP database and mitigate the security risk of running analytical queries directly on it.
- Provides a unified view of data
The ability of data fabric to provide a unified view of data across disparate sources will be invaluable for your business organized across multiple categories. It will enable each category team to access relevant data and insights easily.
- Provides real-time insights
The data fabric approach can also support real-time or near-real-time insights, which would be beneficial for teams like the website team that need to run experiments and gain timely insights.
Despite its benefits, remember that implementing a data fabric requires a significant investment of time and resources. You’d also need to encourage a change in mindset and culture, as you move from a more siloed approach to data management to a more integrated, collaborative one.
So, while it could potentially solve your problem and provide significant benefits, it’s crucial to consider these factors and plan accordingly.
What is data fabric used for: Taking a closer look with case studies & examples #
Now, let us examine a few data fabric use cases along with their implementation across industries.
1. Data discovery and governance #
This refers to the process of identifying the data available in an organization and managing it in a way that ensures compliance, quality, and security.
For example, a healthcare organization might use data fabric to track all instances of patient data across its systems. The data fabric can help them understand where the data came from (lineage), who has access to it, and how it’s being used (metadata management). This is crucial for compliance with regulations like HIPAA.
With data fabric, healthcare companies can integrate their numerous data repositories, enabling centralized data governance, monitoring, and policy enforcement.
This assures that patient data remains private and compliant, reducing the risk of penalties while also enabling efficient patient care via unified data access.
2. Data integration and orchestration #
Data integration is about combining data from different sources and providing users with a unified view of this data.
For instance, a retail company may have data in a cloud-based sales system, an on-premises inventory system, and a third-party e-commerce platform. Data fabric can help integrate data from these disparate sources into a consistent format that’s ready for analysis.
An automobile manufacturer can implement data fabric to bring together production data, supply chain information, sales data, and customer feedback. This results in more efficient production planning, improved quality control, and the ability to quickly adapt to market trends.
A telecom company can deploy data fabric to consolidate data from its various systems related to customer data, network data, billing, and support. This enables them to provide better customer service, optimize network performance, and develop targeted pricing strategies.
Orchestration refers to automating the workflows of data transformation, enrichment, and validation. It allows for the seamless execution of complex data processes.
3. Data security and privacy #
Today, data breaches are common. So, data security and privacy have become paramount.
For example, a financial institution might use a data fabric to apply consistent security measures, such as masking and encryption, across their data estate. This helps protect sensitive information like credit card numbers or social security numbers from being accessed by unauthorized individuals.
Moreover, in case of data anonymization requirements (for instance, to comply with GDPR), data fabric can help ensure these policies are consistently applied.
4. Real-time fraud detection #
A bank may face issues with delayed fraud detection due to siloed transactional data and lacked real-time processing capabilities. Implementing data fabric will enable the bank to stream transactional data in real-time across systems and integrate it with analytics tools.
As a result, the bank can detect suspicious activities immediately as they occur, thereby reducing financial losses and enhancing customer trust.
5. Data quality #
Data fabric can help to ensure data quality by checking for errors, inconsistencies, and inaccuracies.
For example, a logistics company might use data fabric to ensure that the addresses in their delivery database are correctly formatted and standardized. This approach reduces delivery errors. Other data quality processes like deduplication, validation, or enrichment can also be performed.
6. Enabling real-time insights #
Many businesses require up-to-date, real-time data for decision-making.
For instance, a news media company might use data fabric to ingest real-time social media feeds, process the data immediately, and analyze the sentiment toward certain news items. This can inform their reporting and content strategy on the fly.
Or an investment management firm can deploy data fabric to connect real-time market data, historical data, and data from multiple trading platforms. This integrated data environment facilitates faster and more accurate trading decisions and risk assessments.
7. Integrating analytics #
An automotive manufacturer might want to use the data from sensors in its vehicles to predict maintenance needs and enhance the driving experience. Using data fabric, the manufacturer can collect and process data at the edge (in the cars themselves) and integrated it with centralized systems for deeper analytics.
This allows for real-time alerts to drivers about potential issues, predictive maintenance schedules, and the development of new features based on driving behavior analytics.
8. Content cataloging and discovery #
A media streaming company with a vast content library might find it challenging to offer personalized content recommendations due to disparate data sources about viewer preferences and content metadata. Data fabric enables the integration of user behavior analytics, content metadata, and third-party data sources.
As a result, the company can offer highly personalized content recommendations, increasing viewer engagement and satisfaction.
Remember that these are generalized examples of how data fabric can be applied across different use cases. The specific use cases and benefits for a particular organization will depend on your business, data infrastructure, and data needs.
When is a data fabric not ideal for you? #
While data fabric provides a comprehensive solution for managing and analyzing data across disparate sources, it may not be suitable for all scenarios. Here are some situations where data fabric might not be the best fit:
- Small-scale data environments
- Organizations with low data complexity and integration needs
- Highly regulated environments
- Where immediate ROI is expected
- Lack of skilled resources
Now, let us look in brief at each of the above environments where a data fabric may not be ideal:
1. Small-scale data environments #
For startups or small businesses with simple data architecture, limited data sources, and manageable data volumes, the implementation of data fabric could be overkill. The cost and complexity of deploying a data fabric might outweigh the benefits for these organizations.
2. Organizations with low data complexity and integration needs #
If an organization’s data sources are largely homogeneous and already well-integrated, or if there’s no need for real-time insights, there is no need for a data fabric.
3. Highly regulated environments #
While data fabric can support data governance and compliance, in some highly regulated environments, the extensive data access it enables might be seen as a risk.
For example, in very sensitive sectors like defense, where data compartmentalization is crucial, using a data fabric might be inappropriate.
4. Where immediate ROI is expected #
Implementing a data fabric is a strategic, long-term decision. It requires significant upfront investment in terms of time, resources, and change management. If an organization is looking for quick wins or immediate return on investment, data fabric might not be the best solution.
5. Lack of skilled resources #
Deploying and managing a data fabric requires specific expertise in data architecture, data engineering, data governance, and more. If an organization doesn’t have access to these skills and isn’t ready to invest in upskilling or hiring, a data fabric implementation could fail.
While a data fabric may be unsuitable in the above scenarios, there may still be exceptions. The decision to implement a data fabric depends on a thorough understanding of your business’s specific context, needs, and capabilities.
Where to learn more about data fabric use cases? #
Here are a few resources that will help you understand the context in which the concept of data fabric has emerged and evolved:
For recent and specific resources on data fabric, your best bet would be to look at the research and reports by leading IT research firms:
- Gartner: Gartner has been covering the concept of data fabric for several years. Their reports on the subject provide an authoritative source of information on the evolution and current state of the data fabric. While some of their content is behind a paywall, there are also free resources and summaries available.
- Forrester: Like Gartner, Forrester has been tracking the development of data fabric. Their research reports offer valuable insights into the trends driving the adoption of the data fabric.
Books #
“Data Architecture: A Primer for the Data Scientist” by W.H. Inmon, Daniel Linstedt, and Mary Levins.
This book is an excellent resource on data architecture. While it doesn’t cover data fabric per se, it provides a comprehensive view of how data should be managed and structured, setting the groundwork for understanding the rationale behind data fabric.
Rounding it all up #
In this blog, we examined the role of a data fabric in maximizing the value of data in organizations. We explored key use cases, including data discovery, integration, security, quality, and real-time insights. We also delved into the benefits of data fabric adoption and potential scenarios where it may not be the best fit.
A data fabric should be used when an organization requires a centralized platform to access, manage and govern all data. The first step is to design a framework that makes sense for your organization. The next step is implementation, which involves deploying a platform that:
- Consolidates all metadata, with context, in a single repository
- Enables active metadata management
- Ensures granular access control and governance
- Empowers open collaboration and sharing of data
That’s where a modern data catalog and governance platform like Atlan can help.
Data fabric use cases: Related reads #
- What is Data Fabric: Components, Use Cases & More
- Data Fabric Architecture: Components, Tooling, and Deployment
- Data Catalog for Data Fabric: 5 Essential Features to Consider
- Data Fabric: Can it Future-Proof Your Architecture, Unify Your Data, and Save Costs?
- Data Fabric vs. Data Virtualization: Overview, Comparison, and Differences
- Data Mesh vs. Data Fabric: How do you choose the best approach for your business needs?
- Implementing a Data Fabric: A Scalable and Secure Solution for Maximizing the Value of Your Data
Share this article