Data Governance Model: Creating a Sustainable Framework for Effective Data Management
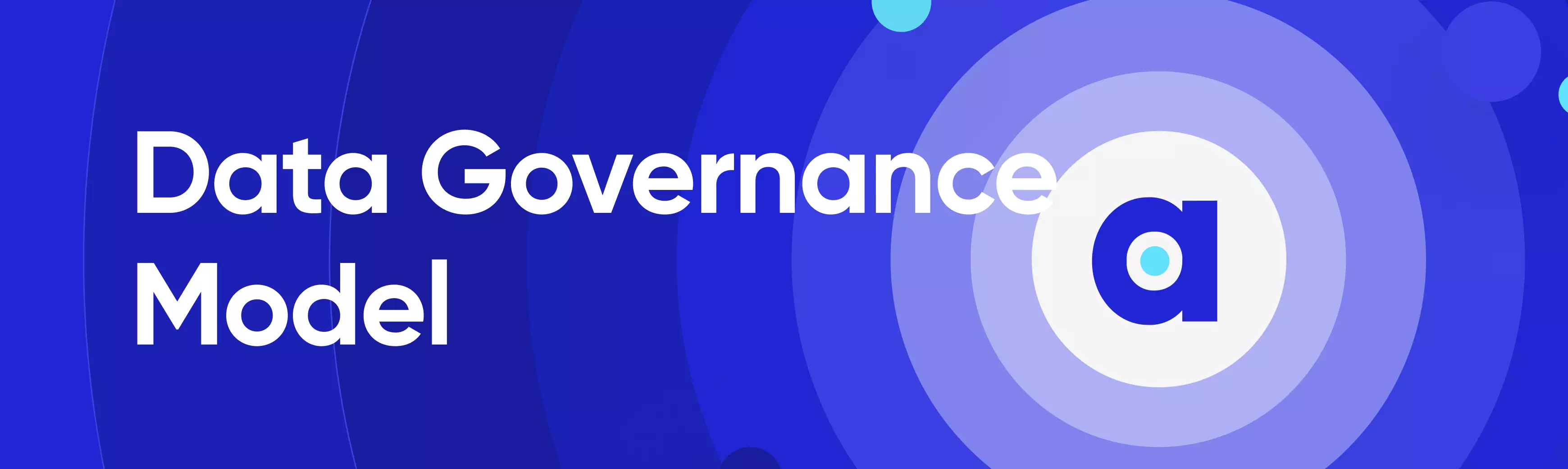
Share this article
A data governance model provides a framework for the policies and procedures that an organization uses to manage its data.
Here’s why your organization needs a strong data governance model - and how to define a model that can scale to meet your company’s needs.
Table of contents #
- Why do you need a data governance model?
- What are the four pillars of a data governance model?
- How to create and implement a data governance model?
- Brainly: People and processes over tools
- Data governance model: Related reads
Why do you need a data governance model? #
A data governance model is, first and foremost, about deciding how to decide.
Without a data governance model, you have no guidelines for identifying the data your organization manages or regulating access to it. You remain stuck in a top-down, centralized model of data management that doesn’t scale.
With a solid data governance model in place, you can rapidly improve your company’s data maturity and reap several tangible benefits, such as:
- Democratizing access to data
- Improved data quality
- Better decision-making
- Better compliance and regulatory adherence
- Increased efficiency
- Reduced risk
Democratizing access to data #
By defining an overarching set of policies, practices, and security measures governing data, you enable employees and business units to manage their own data in a safe, secure, and reliable manner. Centralized tooling, such as a data catalog, enables everyone to discover and verify the veracity of data across the company.
A data governance model enables a new decentralized, democratized approach to data management. New data projects don’t need to wait on permission or resources from IT or the data engineering team. Data storage and analysis become a bottom-up, practitioner-led practice.
Improved data quality #
Do you know what data your company has? Where it came from? When it was last updated? Who has access to it?
Without answers to these questions, you can’t be sure that the data you have is the right data.
A data governance model helps ensure that the data used across an organization is accurate, complete, and consistent. This, in turn, can lead to better-quality products and services, improved customer satisfaction, and increased operational efficiency.
Better decision-making #
McKinsey says that organizations without a strong data governance model spend as much as 30% of their time on non-value-added tasks. That’s because employees must spend time finding data of high enough quality to make decisions.
A data governance model helps ensure that the data used to inform decisions is accurate, reliable, and consistent. With a well-defined data governance model, decision-makers can have confidence in the data they use to make critical business decisions.
Better compliance and regulatory adherence #
Adhering to standards like the European Union’s General Data Protection Regulation (GDPR) is impossible without a data governance model.
A strong model helps organizations comply with various regulatory requirements and standards, such as GDPR, HIPAA, and PCI DSS. Compliance with these regulations is critical, as non-compliance can result in significant financial and reputational damage.
Increased efficiency #
A data governance model creates a framework for managing data assets and streamlining data-related processes. This fosters increased efficiency, as data management processes are standardized, streamlined, and optimized.
This is especially true for organizations that automate key portions of their data governance model. Automation can ensure that security and compliance policies are implemented uniformly and automatically. Automation can also improve data quality through data cleaning and establishing data lineage.
Reduced risk #
Without a data governance model and a consistent framework for regulating access to data, you can’t define who should have access to what. That leads to some employees having overprivileged access, and some data even being left wide open.
Such lapses are costly. IBM estimates that data breaches cost companies USD $4.24 million per incident.
With a data governance model, you can identify and manage data-related risks, such as data breaches, data loss, and data corruption. By implementing a robust data governance model, you can reduce the likelihood of data-related risks and minimize the impact if they do occur.
What are the four pillars of a data governance model? #
Here are the four indispensable pillars on which any data governance model should focus.
- Data quality
- Data security
- Data privacy
- Data stewardship
In the next section, we’ll dive into how to put these into practice.
Data quality #
- Ensure the data your organization uses is accurate, complete, and consistent.
- Establish data standards, processes for data validation, and measures to monitor data quality.
Data security #
- Protect data from unauthorized access, modification, or destruction.
- Define access controls, encryption standards, and security protocols to ensure that data is protected at all times.
Data privacy #
- Ensure personal and sensitive data is handled in compliance with applicable regulations and privacy laws.
- Define data privacy policies, obtain consent for data use, and implement measures to safeguard data privacy.
Data stewardship #
- Define clear roles and responsibilities for data ownership, management, and usage within an organization.
- Establish a framework for data governance, assign data stewardship roles, and implement data management processes to ensure that data is used in a responsible and accountable manner.
How to create and implement a data governance model #
Putting a data governance model into practice requires more than just buying and implementing a set of tools. First, you need a clear vision as well as organizational buy-in.
Follow these steps to build your data governance model:
- Align your organization
- Define your goals
- Rank your current data governance maturity
- Define your data governance model
- Invest in the necessary infrastructure
Align your organization #
Data governance is the responsibility of the entire company. Before establishing a governance model, you need to incorporate key groups and decision-makers from across your organization. These include:
- The Chief Data Officer (CDO) or another C-suite executive with approval, funding, and staffing authority.
- Data governance program management team. This team owns the day-to-day responsibility of gathering requirements, coordinating policy approvals, standing up tools, and educating users. It establishes the KPIs that determine success and collects metrics to measure progress toward these goals.
- Data governance committee. A cross-company, cross-discipline group that owns establishing data governance model policies and principles. While the program management team is responsible for implementing and driving success, the process itself requires buy-in from across the company if it’s going to be successful.
- Data stewards. Stewards oversee individual data sets. They are typically subject matter or line of business experts and are responsible for overseeing data quality, access, sharing, and classification.
Define your data governance goals #
The ultimate end goal of a data governance model is to foster a human-centric approach to data. Typical goals of a data governance model include:
- Share knowledge seamlessly across your organization
- Increase trust in data
- Drive value through increased collaboration
- Make security and compliance a part of everything you do
Define which of these goals are top of mind for your organization. Then, come to an agreement on how you’ll measure and track progress toward each goal.
Rank your organization on the data governance maturity model #
You can’t know where you want to go if you don’t know where you are. By placing your organization on the data governance maturity model, you gain a clear perspective on what steps you need to take next to make your data governance model a success.
Data governance maturity model. Source: Atlan.
For example, if you’re at Level 0, you know your primary task is socializing the need for data governance and driving both team-level and C-suite buy-in. If you’re on Level 2 and already have a data governance project deployment, you know your next step is to evaluate the data you’ve gathered and use it to improve subsequent deployments and drive further adoption.
Define the data governance model your business requires #
Historically, data governance models have used one of the multiple approaches:
- A centralized, top-down approach where all data is managed in a single set of repositories with a set of uniform policies
- A decentralized, bottoms-up approach where teams take ownership and responsibility for vetting and security of their own data
- A hybrid approach where a centralized team defines core services (such as security) while individual teams own and control their own data
Centralized approaches and completely decentralized approaches tend to create slow processes and encourage data silos. By contrast, a hybrid solution fosters a more human-centric data approach while ensuring security and regulatory compliance are implemented uniformly throughout the company. It’s up to your organization to define how to balance these two priorities.
Invest in the necessary infrastructure #
A human-centric, self-service approach to managing data requires the right tooling.
The first step for many organizations is investing in a data catalog. If your teams are unsure of what data to use, can’t find the right data, and don’t know what metrics related to data even mean, a data catalog should be your first investment.
Data catalogs do more than just provide a central repository and break down data silos. They are also key in tracking data lineage, an indispensable part of verifying the authenticity and timeliness of data.
Iterate repeatedly over your data governance model #
A data governance model isn’t something you roll out to an entire company overnight. Rather, identify a single team and bring their data set under the governance of a data catalog. Then, use the feedback and learnings from the first experiment to expand the model to other teams.
With each new deployment, your organization will learn more about the best way to balance centralized governance with a democratized data model. Even when you’re near 100% saturation, you can use your metrics to identify issues with data cleanliness or security and continue to improve your implementation.
Brainly: People and processes over tools #
When tackling a large problem such as a data governance model, it’s tempting to jump right into buying tools and crafting a solution. But tools aren’t effective without an effective process.
Brainly’s experience demonstrates the importance of a data governance process. After deploying a data catalog, Brainly quickly realized how critical people were to the process. As a result, it implemented clear data ownership policies and created incentives to document data assets across the company.
Conclusion #
A data governance model is critical for taking your organization from data chaos to a mature, centralized, and democratized data governance practice that ensures accuracy, timeliness, compliance, and security.
Data governance model: Related reads #
- What is Data Governance? Its Importance, Principles & How to Get Started?
- How AI in Data Governance Shows Potential To Help You Scale Data Security, Integrity, Privacy, and Compliance
- Data Governance and Compliance: Act of Checks & Balances
- Data Catalog: Does Your Business Really Need One?
- Enterprise Data Governance — Basics, Strategy, Key Challenges, Benefits & Best Practices
- Automated Data Governance: How Does It Help You Manage Access, Security & More at Scale?
- Data Governance Policy — Examples, Templates & How to Write One
Share this article