Information Science vs Data Science: 5 Key Differences!
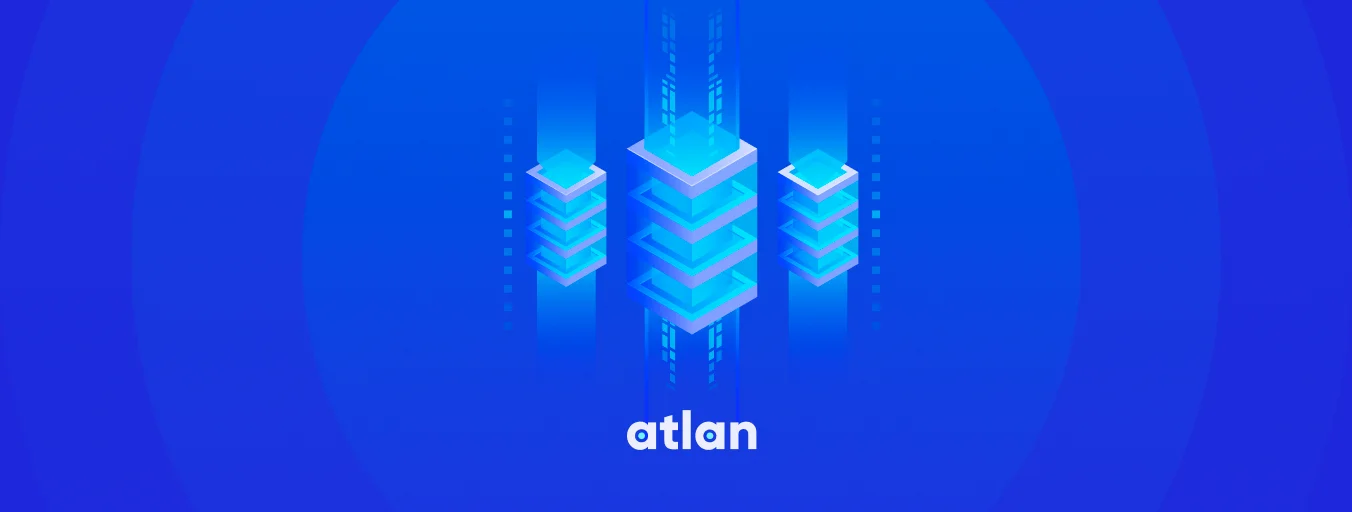
Share this article
Information science focuses on the effective organization, retrieval, and utilization of information, while data science involves extracting insights and knowledge from structured and unstructured data through statistical, mathematical, and computational methods.
Modern data problems require modern solutions - Try Atlan, the data catalog of choice for forward-looking data teams! 👉 Book your demo today
In this article, we will understand their definitions, key differences, and their interrelationships. Whether you’re an experienced leader or a newcomer, this guide will clear up the confusion between information science and data science.
So, let’s kick things off with the fundamentals.
Table of contents #
- What is information science?
- What is data science?
- Information science vs data science: 5 Key differences
- Overlap and intersections between information science vs. data science
- To conclude
- Related reads
What is information science? #
Information science is a field that delves into how we collect, organize, store, and use information.
Think of it as the science behind how we manage all the information available to us, especially in this digital age where data comes from countless sources every day.
For business owners and decision makers, understanding the basics of information science can be invaluable. It gives insight into how information flows, how it can be managed effectively, and how it can be used to make informed decisions.
For business owners and decision makers, it’s crucial to understand some of its primary concepts. The core concepts of information science are as follows:
- Information theory
- Information retrieval and organization
- Information behavior and users
- Knowledge representation
- Digital libraries and archives
What is data science? #
Data science is a field that has captured the attention of businesses around the world, and for good reason. But what exactly is it? Let’s break it down in simple terms.
In today’s data-driven era, businesses benefit immensely from understanding the fundamentals of data science. The field provides the foundation for making informed decisions, spotting trends, and predicting future outcomes.
For business owners and decision-makers, having a grasp on these concepts can help in leveraging data effectively. The core concepts of data science are:
- Data collection and preprocessing
- Statistical analysis and inference
- Machine learning and predictive modeling
- Data visualization
- Big data technologies and infrastructure
Information science vs data science: 5 Key differences #
Information science focuses on the study and management of information systems, emphasizing the organization and retrieval of knowledge. On the other hand, data science involves extracting insights and knowledge from large volumes of structured and unstructured data through statistical, mathematical, and programming techniques.
For business owners and decision-makers, grasping the distinctions between information science and data science is paramount. Both disciplines can reshape the way organizations manage and use data.
Now we will understand the detailed differences in clear, concise, and jargon-free terms, ensuring that you’re equipped to make informed decisions:
- Scope and objectives
- Tools and methodologies
- Interdisciplinary nature
- Duration and speed of outcomes
- Roles and professionals
Let’s look at them in detail:
1. Scope and objectives #
- Information science
Think of information science as the foundation of a library. It ensures books are well-organized, categorized, and easily retrievable. In the business realm, it translates to how data is stored, cataloged, and accessed. Its primary goal is to ensure information is available, reliable, and comprehensible.
- Data Science
Data science, in contrast, is akin to the analysts who read, interpret, and derive meanings from the books in our metaphorical library. It dives into complex and scattered data, extracting patterns, insights, or predictions. Businesses rely on data science to harness actionable insights from their data, guiding strategic decisions.
2. Tools and methodologies #
- Information science
Predominantly uses databases, content management systems, and taxonomy structures. Its tools aim at optimizing data categorization, storage, and retrieval. A business might lean on information science tools for tasks like storing product catalogs or maintaining customer records.
- Data Science
Leverages statistical tools, machine learning algorithms, data visualization software, and big data technologies. The goal is to analyze, represent, and sometimes, even predict trends based on data. For instance, a business might employ data science tools to forecast sales or understand customer preferences.
3. Interdisciplinary nature #
- Information science
Intersects with areas like library science, cognitive science, and even design principles. This ensures the information presentation and retrieval processes are user-friendly and intuitive.
- Data Science
Rooted in mathematics, statistics, and computer science. Its interdisciplinary approach ensures that insights are robust, comprehensive, and actionable.
4. Duration and speed of outcomes #
- Information science
Often focuses on long-term solutions like creating sustainable data storage systems or designing information retrieval protocols. Results can be seen over extended periods as systems are fine-tuned for optimal performance.
- Data Science
Can provide quicker results, especially when it comes to analytics. Data scientists might analyze a week’s worth of sales data to provide insights for the next marketing strategy, leading to immediate actionable steps.
5. Roles and professionals #
- Information science
Roles in this field include information architects, database administrators, and librarians in the digital domain. These professionals ensure that data systems are structured, reliable, and user-friendly.
- Data Science
Data scientists, data analysts, and machine learning engineers are some of the key roles here. They focus on dissecting data, understanding its nuances, and providing actionable insights or predictive models based on it.
While both information science and data science are crucial in today’s data-rich business environment, they serve distinct yet complementary roles. A holistic approach that understands and integrates the strengths of both domains will position organizations at the forefront of data-driven success.
Overlap and intersections between information science vs. data science #
In today’s digital landscape, it’s hard to discuss information and data without acknowledging how closely the two fields intertwine. Both information science and data science contribute to the way we store, manage, and extract value from data.
By understanding their overlaps and intersections, businesses can better harness the strengths of both to make informed decisions.
#1 How data science techniques are used in information science tasks #
- Information retrieval with machine learning
When we talk about retrieving information, we often think of search engines. Data science, particularly machine learning, plays a pivotal role in improving the accuracy and efficiency of these engines.
Machine learning algorithms can predict what a user is looking for by analyzing patterns in search queries, thus providing more relevant results. For instance, when you type a query into a search engine, it’s not just looking for keyword matches; it’s predicting what you might be looking for based on countless other queries and user behaviors.
- Data classification and categorization
Information science is all about organizing information in a manner that makes it easily accessible. With vast amounts of data coming in every day, manual categorization is not feasible.
Data science techniques, like clustering algorithms, help automatically categorize and segment data into relevant groups. This ensures that when a user is looking for a specific piece of information, the system can pull up related and relevant data without much hassle.
- Recommendation systems
Whether it’s suggesting a book in an online library or a product on an e-commerce site, recommendation systems are a direct application of data science in information science. By analyzing user behaviors, preferences, and historical data, algorithms can suggest items that a user is likely to be interested in.
#2 How information science principles guide data organization and representation in data science #
- Structured data storage
Information science emphasizes the importance of structuring data in a way that makes it easily retrievable. In data science, this principle is crucial. Before any analysis can be done, data must be stored in structured formats like tables or databases, ensuring that the analysis is efficient and accurate.
- Metadata and annotation
Just as a library book might come with an index or a summary, data often comes with metadata – data about the data. This principle from information science ensures that data scientists have context for the data they’re working with.
Metadata can provide crucial information about when, where, and how data was collected, which can impact how it’s analyzed.
- Data integrity and quality
Information science has always highlighted the need for accurate, reliable information. When transferred to data science, this principle emphasizes the importance of clean, high-quality data.
Before any data analysis, processes are applied to clean and preprocess data, ensuring that the results are based on accurate and trustworthy information.
While information science and data science may seem like distinct fields, they deeply influence and enrich each other. By understanding their overlaps, businesses can ensure that they’re leveraging the full potential of their data and information resources.
Whether it’s improving search capabilities, ensuring data is organized efficiently, or guaranteeing the accuracy of analysis, the intersection of these fields is driving the future of how we use and understand data.
To conclude #
Understanding the distinction between information science and data science is crucial for business leaders. While information science focuses on organizing and accessing information, data science extracts valuable insights from large datasets.
For businesses, these fields are not just separate entities but complementary tools. Grasping their nuances ensures not only organized data but also meaningful insights for informed decision-making.
In a world driven by data, a clear comprehension of these areas is indispensable for sustained success.
Information science vs data science: Related reads #
- Data Governance vs Data Analytics: Which is Best for You?
- Modern Data Management: 8 Things You Can Gain From It
- Data Consistency 101: Causes, Types and Examples
- Modern Data Team 101: A Roster of Diverse Talent
- Bad Data: How It Sneaks in & How to Manage It in 2023
- Who is Responsible for a Data Catalog? Demystifying Roles and Responsibilities
Share this article