Data Governance vs Data Analytics: Which is Best for You?
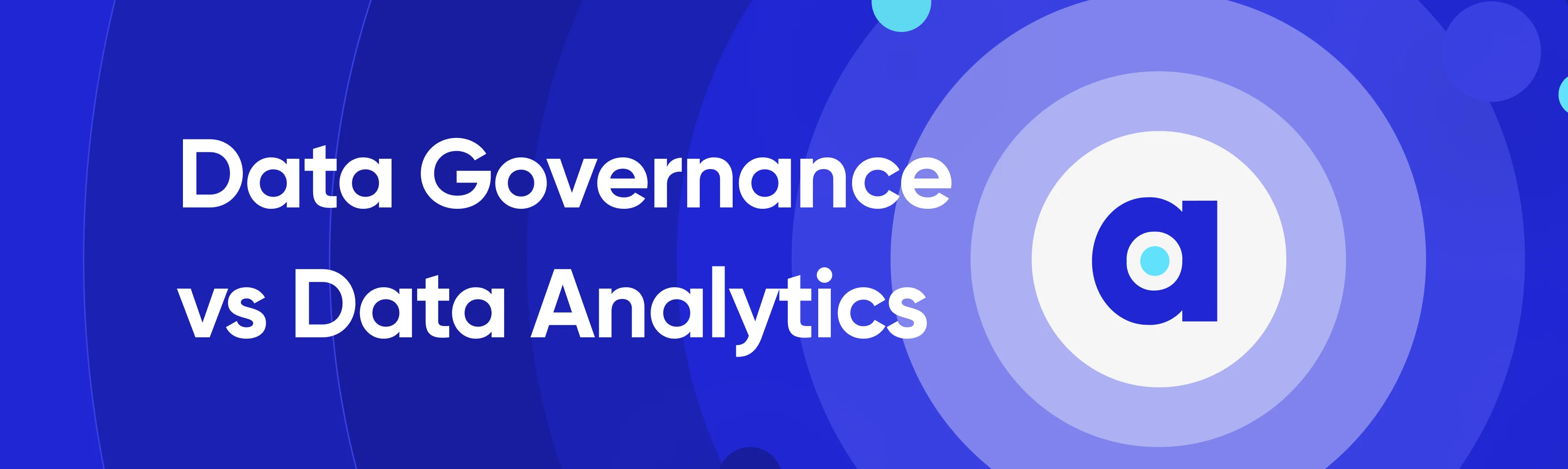
Share this article
Data analytics and data governance are two essential components of a successful data-driven organization, each serving distinct purposes in managing and utilizing data effectively. While they have different objectives, they are both critical for maximizing the value and security of an organization’s data assets.
Both data analytics and data governance play pivotal roles in a data-driven organization. Data analytics unlocks the potential of data by extracting valuable insights, while data governance provides the foundation for reliable, secure, and compliant data management.
Embracing both aspects ensures that an organization can leverage data to its fullest potential while maintaining data integrity and security, ultimately leading to better decision-making and a competitive advantage in the digital age.
In this article, we will learn the key differences between data governance and data analytics, their objectives, and how robust data governance can aid better data analytics.
Let’s dive in!
Table of contents #
- Data governance vs data analytics: Understanding the basics
- Data governance and data analytics
- A tabular view
- Is data analytics part of data governance?
- Objective of data governance in data analytics
- How does data governance help in data analytics?
- Summarizing it all together
- Related reads
Data governance vs data analytics: Understanding the basics #
What is data analytics? #
Data analytics is the process of examining and interpreting large datasets to extract valuable insights, patterns, and trends. It involves using various statistical and computational techniques to identify meaningful information, allowing organizations to make informed decisions, optimize processes, and gain a competitive edge.
Data analytics empowers businesses to uncover valuable information hidden within their data, enabling them to understand customer behavior, predict market trends, optimize operations, and identify new opportunities.
What is data governance? #
Data governance, on the other hand, is the framework of policies, processes, and standards that govern how an organization manages, protects, and utilizes its data. It involves defining roles and responsibilities, establishing data quality guidelines, ensuring data compliance with regulations, and setting data access controls.
Data governance aims to maintain data integrity, accuracy, and security throughout its lifecycle, ensuring that data is reliable, trustworthy, and aligned with the organization’s strategic goals.
In the next section, we will explore the key differences between data governance and data analytics in detail.
What is the difference between data governance and data analytics? #
Data governance and data analytics are two distinct but interrelated concepts that play crucial roles in managing and leveraging data within an organization. Here are the key differences between data governance and data analytics:
1. Focus #
- Data governance: Data governance focuses on the governance and management aspects of data. It deals with issues related to data quality, data security, data privacy, data access controls, data sharing, and compliance with regulations and policies.
- Data analytics: Data analytics, on the other hand, concentrates on the analytical process itself. It involves applying statistical, machine learning, and data mining techniques to discover patterns, trends, and correlations in the data to derive valuable insights.
2. Activities #
- Data governance: Data governance activities include defining data policies, data standards, data classification, data ownership, data access controls, data stewardship, data lineage, and data compliance management.
- Data analytics: Data analytics activities involve data exploration, data cleaning, data transformation, data modeling, data visualization, and the application of statistical and machine learning algorithms to gain insights and draw conclusions from the data.
3. Scope #
- Data governance: Data governance covers the entire data lifecycle, from data creation to data archival or disposal. It is concerned with the broader data management framework and ensures that data is well-maintained and protected throughout its lifecycle.
- Data analytics: Data analytics focuses specifically on the analysis phase of the data lifecycle. It deals with data at the point of analysis and uses various techniques to extract meaningful information from the data.
4. Relationship #
- Data governance and data analytics: Data governance and data analytics are closely related and complementary. Effective data analytics depends on the quality and reliability of the underlying data, which data governance helps ensure. Data governance, in turn, benefits from the insights generated by data analytics, as it enables data-driven decision-making and continuous improvement of data management processes.
It is essential to understand that data analytics and data governance are not in direct competition but rather complement each other to achieve overall data success. The effectiveness of data analytics depends on the quality, consistency, and security of the underlying data, which data governance helps ensure. Data governance, in turn, benefits from the insights generated by data analytics, as it allows organizations to make data-driven decisions to improve their data management processes continually.
Data governance vs data analytics: A tabular view #
Below is a tabular differentiation between data governance and data analytics:
Aspect | Data Governance | Data Analytics |
---|---|---|
Definition | Data governance refers to the management and control of an organization’s data assets, policies, and procedures to ensure data is used appropriately. | Data analytics involves the examination, interpretation, and transformation of data to discover insights, patterns, and trends for making data-driven decisions. |
Focus | Focuses on data management, security, compliance, and data quality. | Focuses on extracting knowledge and actionable insights from data to drive business decisions. |
Purpose | Ensures data is properly collected, stored, and utilized to align with business objectives and comply with regulations. | Utilizes data to answer specific business questions, optimize processes, and gain competitive advantages. |
Role of Data | Treats data as a critical asset and defines its ownership, usage, and access policies. | Views data as raw material and applies various analytical techniques to extract valuable insights. |
Activities | Establishes data policies, data standards, data classification, access controls, and data lineage. | Involves data exploration, data cleaning, data modeling, data visualization, and predictive modeling. |
Stakeholders Involved | Involves data stewards, data owners, compliance officers, and data management teams. | Involves data analysts, data scientists, business analysts, and domain experts. |
Timeframe | Encompasses the entire data lifecycle, from data creation to data disposal. | Focused on specific data analysis tasks or projects within a defined timeframe. |
Relationship to Data | Manages the data infrastructure and ensures data accuracy, consistency, and security. | Utilizes data as inputs to extract insights and generate meaningful outcomes. |
Impact on Decision-Making | Ensures data used for decision-making is reliable, trustworthy, and complies with regulations. | Enhances decision-making by providing valuable insights and predictions based on data analysis. |
Benefits | Improved data quality, compliance with regulations, minimized data risks, and increased data-driven decision-making. | Better understanding of business performance, enhanced productivity, and competitive advantage through data-driven insights. |
In summary, data governance focuses on managing and securing data assets, while data analytics focuses on analyzing and extracting valuable insights from data to support decision-making and drive business success. Both concepts are essential and complementary in the modern data-driven landscape.
Is data analytics part of data governance? #
Yes, data analytics is part of data governance, but they serve different functions within the overall data management framework. Here’s how:
- Data governance provides the foundation for effective data analytics. The success and accuracy of data analytics heavily rely on the quality, consistency, and security of the underlying data.
- Data governance ensures that data is properly managed, validated, and classified, reducing the risk of erroneous conclusions drawn from flawed data.
- Furthermore, data governance also plays a role in guiding data analytics practices. It establishes the rules and guidelines for data usage, access permissions, and compliance, ensuring that data is utilized in a way that aligns with the organization’s policies and legal requirements.
In summary, data analytics is an important application of data within the broader data governance framework. Data governance sets the rules and standards for data management, while data analytics leverages the governed data to gain valuable insights and support data-driven decision-making. Together, they create a synergistic relationship, promoting effective data management and maximizing the value derived from an organization’s data assets.
What is the objective of data governance in data analytics? #
Data governance in data analytics refers to the process of establishing policies, procedures, and guidelines to ensure the proper management, quality, security, and privacy of data used in data analytics initiatives. It is a crucial aspect of data analytics that focuses on creating a structured and well-governed data environment to support accurate, reliable, and meaningful data analysis.
The main objectives of data governance in data analytics are as follows:
- Data quality
- Data security and privacy
- Data classification and metadata management
- Data integration and sharing
- Data lifecycle management
- Compliance and risk management
- Data ownership and accountability
Let us understand these objectives in detail:
1. Data quality #
Data governance ensures that the data used in analytics is accurate, consistent, and reliable. It involves defining data quality standards, performing data profiling, and implementing data cleansing and validation processes to improve the overall data quality.
2. Data security and privacy #
Data governance establishes security measures and access controls to protect sensitive data used in analytics. It ensures that only authorized personnel have access to specific datasets and that data privacy regulations and compliance requirements are met.
3. Data classification and metadata management #
Data governance involves classifying data based on its sensitivity and criticality. Proper metadata management is also part of data governance, ensuring that data analysts can easily discover and understand the content and context of the data they are working with.
4. Data integration and sharing #
Data governance facilitates the integration of data from various sources to provide a unified view for analytics. It also establishes rules for data sharing and data exchange with other teams or external partners, ensuring data is used appropriately and securely.
5. Data lifecycle management #
Data governance defines the lifecycle of data, from its creation to archival or deletion. This ensures that data remains relevant and useful for analytics and is properly retired when it is no longer needed.
6. Compliance and risk management #
Data governance ensures that data analytics practices adhere to legal and regulatory requirements. It minimizes risks associated with data breaches, data misuse, and non-compliance with data protection regulations.
7. Data ownership and accountability #
Data governance establishes clear data ownership and assigns responsibilities for data management and data analytics initiatives. This ensures accountability and fosters a culture of data responsibility within the organization.
By implementing robust data governance practices in data analytics, organizations can enhance the effectiveness and reliability of their data analysis, make more informed decisions, and build trust in the insights derived from data. It also helps mitigate risks associated with data usage and ensures that data is treated as a valuable asset, contributing to the organization’s overall success and competitiveness.
How does data governance help in data analytics? #
Data governance plays a critical role in supporting and enhancing data analytics initiatives in several ways:
- Data quality improvement
- Data integration and consistency
- Metadata management
- Data security and privacy
- Data classification and sensitivity
- Data ownership and accountability
- Data governance policies for analytics
- Compliance and risk mitigation
- Data lifecycle management
- Cross-functional collaboration
Let us understand the roles in detail:
1. Data quality improvement #
Data governance ensures that data used in analytics is of high quality and accuracy. By defining data quality standards, data governance helps identify and rectify data inconsistencies, errors, and redundancies, which improves the reliability and trustworthiness of analytical insights.
2. Data integration and consistency #
Data governance establishes rules for data integration from various sources. It ensures that data is standardized and consistent across different datasets, enabling analysts to perform comprehensive and meaningful cross-functional analysis.
3. Metadata management #
Data governance maintains a well-organized and comprehensive metadata repository. This information provides context to the data being analyzed, making it easier for data analysts to understand the meaning, origin, and usage of the data, leading to more insightful interpretations.
4. Data security and privacy #
Data governance ensures that data analytics processes adhere to security and privacy guidelines. By setting access controls, encryption, and data masking, data governance protects sensitive information and ensures compliance with data protection regulations.
5. Data classification and sensitivity #
Data governance classifies data based on its sensitivity and criticality. This classification helps data analysts and other stakeholders understand the level of confidentiality and handling requirements for different datasets, reducing the risk of data misuse.
6. Data ownership and accountability #
Data governance defines data ownership and assigns responsibilities for data management. This accountability ensures that data analysts and other stakeholders take ownership of the data they use and interpret, promoting data integrity and responsibility.
7. Data governance policies for analytics #
Data governance establishes policies specific to data analytics. These policies guide the selection of tools, methodologies, and best practices for conducting data analysis, ensuring consistent and standardized analytical processes.
8. Compliance and risk mitigation #
Data governance ensures that data analytics practices align with relevant regulations and industry standards. This compliance minimizes the risk of legal and financial repercussions associated with data misuse or non-compliance.
9. Data lifecycle management #
Data governance defines data lifecycle management policies, including data retention and archival. This ensures that data analysts work with relevant and up-to-date information while maintaining data efficiency and reducing data storage costs.
10. Cross-functional collaboration #
Data governance fosters collaboration between different teams involved in data analytics, such as data analysts, data engineers, and data stewards. This collaboration promotes better data sharing and understanding, leading to more comprehensive and accurate analyses.
By incorporating data governance principles into data analytics practices, organizations can ensure that their data is of high quality, secure, and compliant with regulations. This, in turn, leads to more reliable insights, better decision-making, and improved overall data-driven performance. Data governance serves as the backbone of data analytics, supporting its success and enabling organizations to unlock the full potential of their data assets.
Summarizing it all together #
In summary, data governance is concerned with the governance, management, and protection of data assets, while data analytics involves the process of analyzing and extracting insights from data.
Both are vital components of a successful data-driven organization and work together to maximize the value and security of an organization’s data.
Data governance vs data analytics: Related reads #
- What is Data Governance? Its Importance & Principles
- Data Governance 101: Principles, Examples, Strategy & Programs
- What is Metadata? - Examples, Benefits, and Use Cases
- Metadata Management: Benefits, Automation & Use Cases
- What Is a Data Catalog? & Why Do You Need One in 2023?
- Business glossary
- Data Governance Framework — Guide, Examples, Template
- Data Governance Roles and Their Responsibilities
Share this article