What is Data Validity? Types, Differences, Example & More!
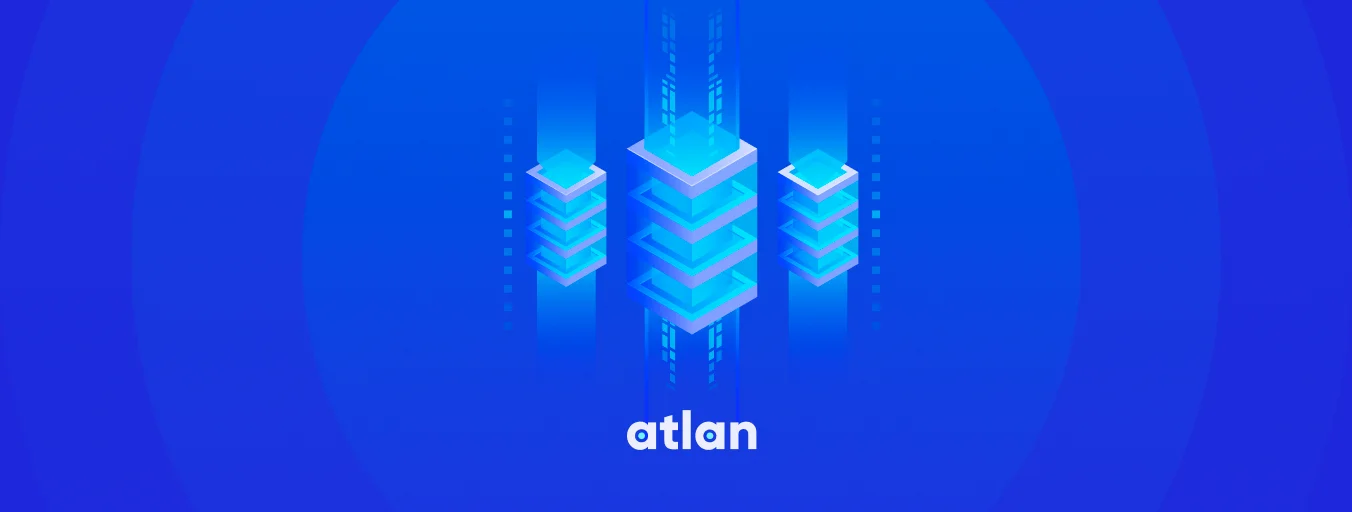
Share this article
Data validity is the measure of accuracy and reliability of data, ensuring it meets specified criteria or rules; it helps maintain data quality and integrity in databases.
Ensuring data validity is critical for making informed decisions and preventing errors; neglecting it poses the risk of unreliable insights and compromised business processes, leading to misinformed strategies and operational inefficiencies.
In essence, while data reliability focuses on the consistency and repeatability of data over time, data validity is concerned with how well the data measures what it is intended to measure. In other words, valid data accurately represents the real-world construct, attribute, or phenomenon that it is designed to capture.
Modern data problems require modern solutions - Try Atlan, the data catalog of choice for forward-looking data teams! 👉 Book your demo today
In this article, we will understand:
- What is data validity?
- 9 Reasons why it is important for data teams
- 8 Types of data validity every data team member should be aware of
- How to check for data validity?
- Differences between data validity, reliability, and accuracy
Ready? Let’s dive in!
Table of contents #
- What is data validity?
- 9 Reasons why data validity is important
- Data validity example
- 8 Different types of data validity
- How to validate data?
- Data validity vs data reliability vs data accuracy
- Summary
- What is data validity: Related reads
What is data validity? #
Data validity is the measure of the accuracy and reliability of information within a dataset or database. It involves verifying that the data conforms to predefined standards, rules, or constraints, ensuring the information is trustworthy and fit for its intended purpose.
Valid data enhances the overall quality and integrity of databases, supporting informed decision-making and preventing errors or inconsistencies in analyses. In essence, data validity assesses whether the data accurately represents the real-world entities, events, or measurements it is meant to describe.
Achieving data validity involves several key components:
- First, it entails the validation of data at the point of entry, where data is collected or recorded, to ensure that it meets predefined criteria and conforms to established standards.
- Second, data validity extends to ongoing data maintenance, where regular checks and audits are conducted to identify and rectify any issues that may arise over time.
- Ultimately, data validity is crucial for decision-making, as decisions based on inaccurate or unreliable data can lead to costly errors and misinformed strategies.
It forms the foundation upon which data-driven organizations build trust in their information assets and rely on them for critical insights and actions.
9 Reasons why data validity is important #
For a data-oriented company, achieving high data validity means a more accurate representation of customer behavior, market trends, internal processes, and other crucial business factors. This directly contributes to the efficiency, competitiveness, and success of the organization.
Here are the nine reasons why data validity is critical for organizations:
- Strategic planning
- Competitive edge
- Customer trust
- Strategic decision-making
- Customer relationship
- Financial planning
- Regulatory compliance
- Market positioning
- Risk assessment
Now, let us look into each of the above purposes of data validity in brief:
1. Strategic planning #
Companies allocate resources and set long-term plans based on data. Invalid data could lead to misallocation of resources and flawed strategies.
2. Competitive edge #
Data analytics can provide insights that offer a competitive edge. The reliability of such insights depends on the validity of the data.
3. Customer trust #
Companies collect data about customer behaviours, preferences, etc. Invalid data could lead to incorrect targeting and communication, which may erode customer trust.
4. Strategic decision-making #
For companies that base their strategic moves on data, the validity of that data directly impacts the effectiveness of the decisions made. Invalid data can lead to poor decisions that have long-term consequences.
5. Customer relationship #
Data validity is crucial for maintaining customer trust. If a company uses invalid data to personalize customer experiences or make recommendations, it risks alienating its customer base.
6. Financial planning #
Companies often use data for budgeting and financial planning. Invalid data can result in underestimation or overestimation of budgets, affecting the financial health of the company.
7. Regulatory compliance #
Many industries have strict regulations about data collection and usage. Using invalid data can lead to non-compliance, resulting in legal troubles and penalties.
8. Market positioning #
Invalid data can paint a distorted picture of market dynamics, leading companies to misposition their products or services.
9. Risk assessment #
Valid data is essential for accurately assessing risks, be it in terms of investments, new market entry, or technological advancements.
Check out the following article to see -> how data validation helps in accurate data collection
Data validity example: Explaining data validity with a typical example #
Let’s explore data validity with an example from the healthcare technology sector, specifically a company that develops machine learning algorithms to predict patient readmissions to hospitals. Accurate prediction is crucial both for improving patient outcomes and for reducing costs.
Background #
The company has a partnership with several hospitals and uses Electronic Health Records (EHR) data to train its algorithms. The goal is to identify the likelihood of a patient being readmitted within 30 days of discharge. Hospital administrators rely on this algorithm to make several key decisions:
- Resource allocation: Hospitals might set aside dedicated beds and staff for high-risk patients.
- Post-discharge care: More intensive follow-up programs can be arranged for high-risk patients.
- Cost estimation: Insurance companies may adjust their premiums or coverage plans based on readmission rates.
Data validity concerns #
Given how important the algorithm is, ensuring the validity of the underlying data is crucial. Here are some points of consideration:
- Content validity: Does the EHR data cover all relevant aspects like patient medical history, treatment given during the hospital stay, socioeconomic factors, etc.?
- Criterion validity: Does a high-risk score from the algorithm actually correlate with real-world readmissions?
- Construct validity: Is the algorithm capturing the complexity of readmissions, or is it perhaps oversimplifying by focusing only on, say, the severity of the illness?
Scenario: Finding invalid data #
During a quarterly review, the data science team notices that the algorithm’s performance has declined. The rate of false positives has increased, identifying patients as high-risk when they are not readmitted within 30 days.
After an internal audit, it is discovered that:
- The data collection method for capturing the patient’s medication upon discharge was faulty.
- The database had not been updated to include new medicines that were recently approved for treating specific conditions.
Impact of invalid data #
- Strategic errors: The hospital had been allocating extra resources for patients falsely identified as high-risk, leading to inefficiencies.
- Erosion of trust: The company risks losing its partnership with the hospitals and other stakeholders if the algorithm continues to underperform.
Corrective actions #
- Pilot testing: The company runs a smaller pilot program to test the effects of including the newly approved medicines in the data.
- Data auditing: The data science team sets up automated checks to flag data that might be incomplete or outdated.
- Cross-validation: The team also starts using additional data sources to validate their existing EHR data, like pharmacy records for medication compliance.
- Expert review: A board of healthcare professionals is consulted to review the variables considered by the algorithm.
Once these corrective measures are implemented, the algorithm’s performance improves, confirming that the data validity issues were the root cause of the previous decline in performance.
Ensuring data validity, in this case, was not just about maintaining the algorithm’s accuracy but also had real-world implications for patient care and resource allocation in hospitals. It serves as a complex, but telling, example of how data validity can significantly impact decision-making in data-oriented companies.
Types of data validity: 8 Types every data team member needs to know #
In the context of data-oriented companies making business decisions, data validity can be categorized into different types, each affecting the quality and usability of the data in distinct ways. Understanding these types is vital for companies to make informed, reliable business decisions.
Below are some key types of data validity:
- Face validity
- Content validity
- Criterion-related validity
- Construct validity
- Internal validity
- External validity
- Statistical conclusion validity
- Ecological validity
Let’s look at them in detail:
1. Face validity #
This is the most basic level of validity where the data appears to be valid at face value, without rigorous statistical checks.
For example, if a company is collecting data on monthly revenue, a quick glance at the data should not reveal any negative numbers or other obvious inaccuracies.
2. Content validity #
Content validity ensures that the data collected adequately covers the research domain of interest.
For example, if a financial services company wants to evaluate customer satisfaction, the data should encompass multiple dimensions like customer service, ease of transactions, and trust in the company.
3. Criterion-related validity #
This involves establishing that the data has predictive or concurrent validity with some external variables or outcomes.
- Predictive validity: If a software company has created an algorithm to forecast churn rates, the predictive validity of their customer behavior data could be determined by how accurately it predicts future churn.
- Concurrent validity: In this case, the data is compared with an existing model or benchmark. For instance, an energy company might compare its predictive model for electricity consumption against actual usage rates in real-time.
4. Construct validity #
This is concerned with whether the data measures the theoretical construct it is designed to measure.
If a consulting firm has developed a complex index to measure the innovative potential of a company, construct validity would test whether the index actually measures innovation rather than something else, like company size.
5. Internal validity #
Internal validity is about the integrity of the experimental design in causal relationships. In business, this would be relevant in A/B testing scenarios.
For example, a tech company could be assessing the impact of a new feature on user engagement. High internal validity would mean that any changes in engagement can be attributed directly to the new feature, ruling out external factors.
6. External validity #
This deals with the generalizability of the data to other settings or populations. For instance, a multinational corporation would be interested in whether consumer behavior data collected in one country holds true in other markets.
7. Statistical conclusion validity #
This is focused on the degree to which conclusions derived from the data are statistically valid. For data-oriented companies, this could relate to ensuring that statistical tests are appropriate, and that sample sizes are sufficiently large to draw meaningful conclusions.
8. Ecological validity #
This type of validity examines how well the data and its interpretations apply to real-world conditions.
For example, a logistics company might simulate delivery routes under ideal conditions. Ecological validity would question how well these simulations translate to real-world scenarios with variables like traffic, weather, etc.
Understanding these types of validity can help data-oriented companies ensure that their data collection and analysis methods are robust, thereby increasing confidence in the business decisions based on that data.
How to validate data? 8 Key strategies #
Ensuring data validity is crucial for making reliable and accurate business decisions. For data-oriented companies, the following are some effective strategies to check for various types of data validity:
- Preliminary checks
- For face and content validity
- For criterion-related validity
- For construct validity
- For internal and external validity
- For statistical conclusion validity
- For ecological validity
- Ongoing monitoring and audits
Let’s understand them in detail.
1. Preliminary checks #
- Data source verification
The first step is to ensure that the data sources are reliable. Whether it’s internal databases or third-party vendors, the reputation and reliability of the source can offer an initial gauge on data validity.
- Descriptive statistics and visualization
Running basic descriptive statistics and creating visualizations can provide insights into anomalies, outliers, or patterns that may question the data’s validity.
2. For face and content validity #
- Expert review
For checking face and content validity, subject matter experts can assess whether the data appears to be valid at face value and whether it sufficiently covers all the dimensions relevant to the decision-making process.
- User feedback
Sometimes, employees or end-users who work closely with the data can provide insights into its validity based on their practical experience.
3. For criterion-related validity #
- Correlation analysis
To determine if the data accurately predicts or correlates with external variables, statistical tests for correlation can be performed.
- Benchmarking
Comparing key metrics against industry benchmarks or similar datasets can validate whether your data is aligned with broader trends or expectations.
4. For construct validity #
- Factor analysis
This statistical method can be used to assess whether the data actually represents the constructs it’s supposed to measure.
- Hypothesis testing
Conduct controlled experiments to test hypotheses related to the construct in question. If the data supports your hypotheses, this provides evidence of construct validity.
5. For internal and external validity #
- Control groups
In experimental designs, use control groups to isolate variables and ensure that any changes can be attributed to the factor you are studying.
- Random sampling
For external validity, ensure that the sample is representative of the population. This makes it more likely that findings can be generalized.
6. For statistical conclusion validity #
- P-value analysis
Ensure that statistical tests are conducted appropriately and that the p-values support the validity of your conclusions.
- Sample size calculation
Check that your sample size is sufficiently large to draw meaningful conclusions.
7. For ecological validity #
- Real-world testing
Validate your findings under real-world conditions. For instance, if you’ve developed a predictive model for equipment failure, test it under actual operational conditions.
- Time-series analysis
Ensure that data validity holds across different time periods, especially if the data will be used for long-term decision-making.
8. Ongoing monitoring and audits #
- Automated checks
Implement automated data quality checks to flag anomalies, outliers, or inconsistencies in real-time.
- Regular audits
Schedule regular data audits that involve detailed analysis and expert reviews to ensure ongoing validity.
- Feedback loops
Set up mechanisms to gather feedback from decision-makers and other stakeholders who rely on the data, to continuously validate its utility and relevance.
By employing these techniques and strategies, data-oriented companies can rigorously validate their data, thereby enhancing the reliability of the business decisions made based on that data.
Data validity vs data reliability vs data accuracy: How are they different? #
Data validity assesses conformity to predefined standards, data reliability ensures consistent results over time, and data accuracy measures the closeness of data to the true values. Together, they form a comprehensive framework for evaluating the quality, consistency, and truthfulness of data in various applications.
Here are the key differences among data validity, data reliability, and data accuracy:
Aspect | Data Validity | Data Reliability | Data Accuracy |
---|---|---|---|
Definition | Measures if data accurately represents the concept it's meant to measure. | Measures the consistency and stability of data over time. | Measures how closely the data matches the true or actual values. |
Importance | Ensures that the data is appropriate and meaningful for the specific context. | Ensures that the data can be replicated under the same conditions and still yield the same results. | Ensures that the data is free from errors and inaccuracies. |
Concerns | Whether data comprehensively covers the domain it claims to measure. | Whether the same data would be collected if the measurement were repeated. | Whether the data precisely matches the real-world values it represents. |
Example | In a customer sentiment analysis tool, data validity would concern whether the tool actually measures sentiment rather than some other variable like frequency of communication. | In the same tool, reliability would be tested by seeing if the tool produces the same sentiment score when analyzing the same set of customer reviews multiple times. | Accuracy would relate to whether the sentiment scores correctly reflect the customers' actual feelings. |
Testing Methods | Expert review, correlation analysis, hypothesis testing, factor analysis. | Test-retest method, parallel forms method, internal consistency tests. | Error analysis, comparison with a "gold standard," or verified measurements. |
Implication for business decisions | Invalid data could lead to a flawed strategy or misinterpretation of a situation. | Unreliable data can cause inconsistency in decisions, making it difficult to establish long-term strategies. | Inaccurate data can result in immediate operational issues and mistrust. |
In summary #
Data validity ensures that data accurately captures the intended concept, crucial for contextual relevance in decision-making.
The types of data validity include face validity, content validity, criterion-related validity, construct validity, internal validity, external validity, statistical conclusion validity, and ecological validity.
Businesses must validate data through expert reviews and statistical tests, maintain reliability with repeated measurements, and check accuracy by comparing to verified standards. These aspects are interconnected but distinct, and understanding them is vital for the credibility and effectiveness of data-driven business decisions.
What is data validity? Related reads #
- Data Integrity vs Data Validity: Proving They Are Different
- Data Validation vs Data Quality: 12 Key Differences
- What is Data Reliability & How to Go About It in 2023?
- Data Validation Demystified: Ensuring Accuracy in Every Byte
- Data Accuracy in 2023: A Roadmap for Data Quality
- 18 Data Validations That Will Help You Collect Accurate Data
- How to Improve Data Quality in 12 Actionable Steps?
- Data Quality Explained: Causes, Detection, and Fixes
- Automated quality control of data pipelines
- Data Quality Measures: Best Practices to Implement
Share this article