What is Data Reliability? Examples, How to Measure & Ensure!
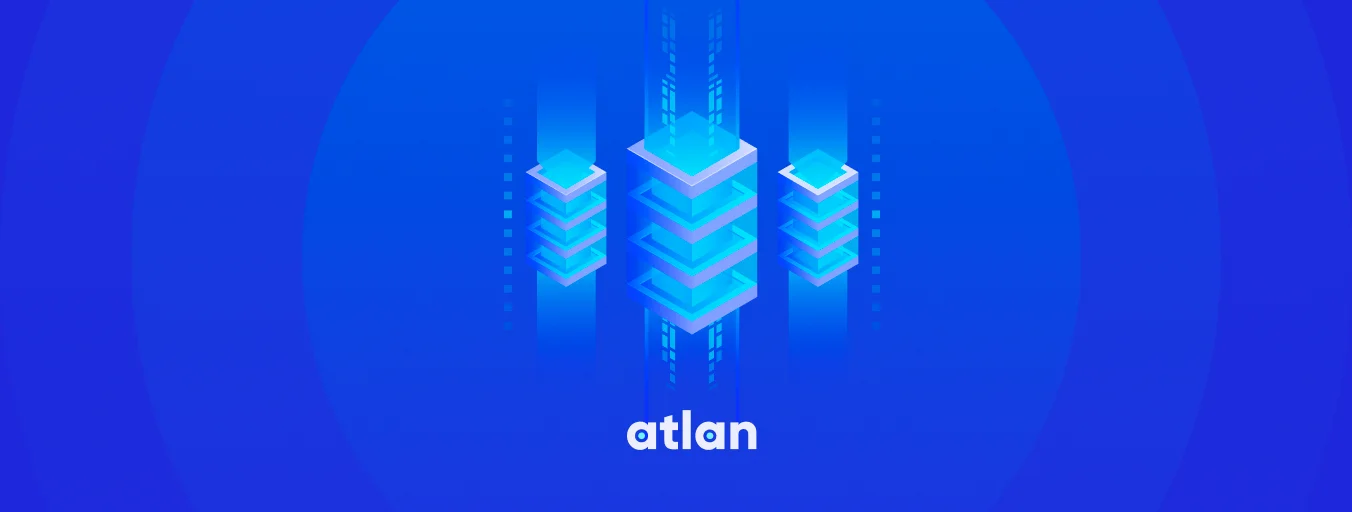
Share this article
Data reliability refers to the extent to which data can be trusted to provide a consistent and accurate representation of some measurement over time.
Reliable data is crucial for making informed business decisions, conducting analyses, and generally supporting the strategic, operational, and tactical aspects of the business.
Besides, it also helps improve compliance and provides a distinct competitive advantage to data-driven businesses.
Modern data problems require modern solutions - Try Atlan, the data catalog of choice for forward-looking data teams! 👉 Book your demo today
In this article, we will understand:
- What is the meaning of data reliability?
- Its practical example
- 14 Steps to ensure data reliability
- 15 Steps to measure reliability of data
- 9 Tools to achieve data reliability
- Differences between data reliability vs validity
Ready? Let’s dive in!
Table of contents #
- What is data reliability?
- Data reliability explained with practical examples
- How to ensure data reliability: 14 Things to get done today
- How to measure data reliability? 15 Ways to go about it
- 9 Essential data reliability tools: Key to achieving data reliabity
- Data reliability vs. data validity: 6 Key differences
- Summary
- Related reads
What is data reliability? #
Data reliability means that data remains consistent and stable over time, producing similar results when conditions stay the same. It is crucial for maintaining the integrity and credibility of any study, research, or analysis, as unreliable data can lead to incorrect conclusions and flawed decision-making.
The purpose of ensuring data reliability is to provide a solid foundation for drawing meaningful insights and making well-informed decisions, whether in academic research, business analytics, or public policy.
Data reliability explained with practical examples #
Data is the consistency and dependability of data over time, ensuring that it accurately reflects the true scenario or conditions it’s supposed to represent. Here are five practical examples to illustrate the concept:
1. E-Commerce inventory management #
An e-commerce platform relies on reliable data to manage its inventory. If the data shows that 100 units of a product are available but in reality, only 10 are in stock, this discrepancy can lead to customer dissatisfaction and loss of trust, as orders cannot be fulfilled as promised.
2. Healthcare patient records #
In healthcare, reliable data is crucial for patient treatment. For instance, if a patient’s electronic health record inaccurately reflects their allergy information, it could lead to incorrect treatment or medication being administered, potentially causing serious harm.
3. Banking transaction processing #
In the banking sector, data reliability is essential for transaction processing. Suppose a bank’s system shows a balance of $1000 in a customer’s account when the actual balance is $100 due to a data glitch. This could result in the customer unknowingly overdrawing their account, leading to financial penalties and trust issues.
4. Manufacturing process control #
In a manufacturing setting, data reliability is key for maintaining product quality. If sensor data inaccurately reports the temperature of a machine, this might lead to defects in the manufactured products, affecting quality control and potentially leading to recalls.
5. Traffic management systems #
In smart city infrastructure, traffic management systems rely on accurate, reliable data for traffic flow control. If the data about vehicle numbers on a road is unreliable, it could lead to inefficient traffic light control, causing unnecessary traffic jams and delays.
In each of these examples, the reliability of data is paramount to ensuring efficient operations, safety, customer satisfaction, and overall success in the respective fields.
How to ensure data reliability: 14 Things to get done today #
Ensuring data reliability is an ongoing process that combines well-defined policies, technology, and human diligence. It’s a cornerstone for data-oriented companies for whom data is not just information but a valuable asset that drives business decisions. Below are key points to ensure data reliability:
- Data governance framework
- Data collection standards
- Data cleaning and validation
- Data auditing and monitoring
- Version control
- Data security measures
- Data backup and recovery
- Data lineage tracking
- Access control and authentication
- Staff training and awareness
- Data quality metrics and KPIs
- Third-party data validation
- Real-time data updates
- Documentation and metadata management
Let us look at the different ways to measure data reliability in brief:
1. Data governance framework #
Establish a data governance framework to standardize data management practices across the organization. This includes policies regarding data collection, storage, usage, and security. A data governance committee should be responsible for formulating these policies and ensuring compliance.
2. Data collection standards #
Set guidelines for how data should be collected. These standards should specify the types of data to be collected, the acceptable sources, and the methodologies for collection. Following consistent data collection practices helps ensure accuracy and comparability.
3. Data cleaning and validation #
Implement automated and manual processes for cleaning and validating data. This includes identifying and correcting errors, filling in missing values, and eliminating duplicates. Validated data is more reliable for analysis and decision-making.
4. Data auditing and monitoring #
Regularly audit data to check for inconsistencies, gaps, or anomalies. Implement real-time monitoring systems that can send alerts in case of irregularities or suspected breaches.
5. Version control #
Use version control systems for datasets to track any changes or updates. This ensures that you can revert to previous versions if needed and offers a transparent history of alterations.
6. Data security measures #
Employ robust security measures, such as encryption and firewalls, to protect against unauthorized access or tampering. Security isn’t just about preventing breaches; it also contributes to data integrity and, thus, reliability.
7. Data backup and recovery #
Establish comprehensive backup and recovery procedures. Regularly back up data to multiple locations, and periodically test recovery processes to ensure they are effective.
8. Data lineage tracking #
Record the lineage of each data element, tracing back to its original source. This practice helps in auditing and ensures that the data can be trusted.
9. Access control and authentication #
Implement strong access control measures, with role-based authentication to ensure that only authorized individuals can access or modify the data.
10. Staff training and awareness #
Conduct regular training programs to make staff aware of the importance of data reliability and how to maintain it. This human factor is often the weakest link in data reliability, so constant awareness is crucial.
11. Data quality metrics and KPIs #
Define and monitor key performance indicators (KPIs) for data quality. Regular reports should be generated and reviewed to measure the effectiveness of your data reliability efforts.
12. Third-party data validation #
If you rely on external data, ensure it goes through a validation process similar to your internal data. Be cautious about the reliability of third-party sources.
13. Real-time data updates #
Ensure that your data systems are capable of real-time updates to reflect current information. This is particularly important for businesses that require immediate data-driven decisions.
14. Documentation and metadata management #
Maintain comprehensive documentation and manage metadata effectively. This should include details about data collection methods, changes made to the data, and the logic behind those changes.
By adopting these practices and incorporating them into your daily operations, you can significantly improve the reliability of your data, thereby enhancing the quality of your business decisions, analytics, and customer experiences.
How to measure data reliability? 15 Ways to go about it #
Measuring data reliability is a crucial step to understand and ensure that the data you’re working with is trustworthy and consistent over time. If data is not reliable, then any decisions made using it can be misinformed.
Here are the different ways to measure data reliability:
- Test-retest reliability
- Split-half reliability
- Internal consistency
- Inter-rater reliability
- Alternate form reliability
- Face validity
- Content validity
- Data source verification
- Cross-verification with external data
- Usage of data quality metrics and tools
- Historical consistency check
- Random sampling and testing
- Monitoring data quality KPIs
- User feedback collection
- Frequency of data errors and anomalies
Let us look at the above points in brief:
1. Test-retest reliability #
It measures the consistency of data over time by comparing data collected at two different points in time under the same conditions. High correlation indicates high reliability.
2. Split-half reliability #
In this method, a dataset is split into two halves, and the results from each half are compared. If they are consistent, the data is considered reliable.
3. Internal consistency #
This assesses the consistency of results across items within a test. Cronbach’s alpha is a common statistical method used to measure this form of reliability.
4. Inter-rater reliability #
It gauges the degree to which different raters or observers agree in their assessments. Cohen’s kappa is a metric often used for this purpose.
5. Alternate form reliability #
This involves comparing the results of two different tests that measure the same phenomenon to determine their consistency.
6. Face validity #
While this isn’t a direct measure of reliability, ensuring that data appears to be a plausible representation of what it’s supposed to measure is an essential step.
7. Content validity #
It assesses how well data covers the full range of a concept. It’s essential when you want to ensure that your data collection method isn’t leaving out important facets of what you’re studying.
8. Data source verification #
Validate the reliability of your data sources. Reliable sources tend to produce reliable data consistently.
9. Cross-verification with external data #
Compare your data with similar data from external, trusted sources. If they align, your data’s reliability is more assured.
10. Usage of data quality metrics and tools #
Use software tools and metrics to assess data quality automatically. They can help identify missing data, inconsistencies, or outliers.
11. Historical consistency check #
Analyze data for historical consistency. If similar conditions produce vastly different data over time without an apparent reason, the data’s reliability might be in question.
12. Random sampling and testing #
Randomly sample parts of your data and conduct in-depth tests. If random samples consistently show high quality, the entire dataset is likely reliable.
13. Monitoring data quality KPIs #
Monitor Key Performance Indicators (KPIs) related to data quality, like the frequency of missing data, the number of detected anomalies, etc.
14. User feedback collection #
Collect feedback from the end-users of the data. Their experience can often highlight reliability issues that might not be evident through quantitative tests.
15. Frequency of data errors and anomalies #
Track how often errors or anomalies arise in your data. A high frequency might indicate underlying reliability issues.
By systematically measuring data reliability through these methods and ensuring regular checks, businesses can have greater confidence in their data-driven decisions. This ensures not only the robustness of business strategies but also upholds the company’s credibility in the eyes of stakeholders.
9 Essential data reliability tools: Key to achieving data reliabity #
Data reliability is ensured through a combination of methodologies, software tools, and hardware infrastructure that work together to provide consistent and accurate results. The crucial tools for achieving data reliability can be categorized into several areas:
1. Data collection instruments #
Surveys, sensors, and other data collection mechanisms must be calibrated and tested to ensure they produce consistent results.
2. Data storage solutions #
Robust databases and data lakes that provide high availability, fault tolerance, and data integrity are key to reliable data storage.
3. Data quality software #
These are specialized software tools that help clean and validate data, removing inaccuracies and inconsistencies.
4. Data analytics platforms #
Software that can reliably analyze data, ranging from simple statistical tools to complex machine learning algorithms, plays a role in ensuring the final results are consistent.
5. Version control systems #
These tools help manage changes in data sets and analytic code, enabling the tracking of changes and facilitating collaboration without compromising reliability.
6. Monitoring and audit tools #
To continuously verify data reliability, automated systems and dashboards monitor data pipelines for errors, inconsistencies, or security issues.
7. Backup and recovery solutions #
Regular backup solutions and fail-safe mechanisms are critical for recovering from data loss or corruption, thereby maintaining data reliability.
8. Validation and testing frameworks #
These methodologies help in assessing the reliability of the data through techniques like cross-validation, backtesting, and peer review.
9. Governance and compliance tools #
These ensure that all data operations comply with legal and policy frameworks, which is critical for maintaining public trust and ensuring long-term reliability.
Each of these tools contributes to the overall reliability of data in its lifecycle from collection to analysis and reporting. Investing in these tools is crucial for any organization that relies on data for decision-making, as it ultimately affects the quality of insights and the integrity of conclusions drawn from the data.
Data reliability vs. data validity: 6 Key differences #
Now, let us understand the main differences between data reliability and data validity:
Aspect | Data reliability | Data validity |
---|---|---|
Focus | Consistency and repeatability of data | Accuracy and truthfulness of data |
Measurement | Assesses if data yields the same results over multiple tests or observations | Assesses if data accurately represents what it's intended to measure |
Concerns | Stability over time, internal coherence, inter-observer agreement | Coverage of the concept, alignment with external standards, face validity |
Methods | Test-retest, Split-half, Inter-rater, Cronbach's alpha | Face validity, Content validity, Criterion validity |
Objective | To ensure that data can be trusted to show the same results under the same conditions | To ensure that data accurately represents the real-world phenomenon it aims to describe |
Implications for poor quality | Results and decisions based on unreliable data may be inconsistent | Results and decisions based on invalid data may be incorrect or misleading |
In summary #
In conclusion, understanding and ensuring data reliability is a multifaceted process critical to the integrity and success of any data-driven operation. This article has explored the essence of data reliability, offering practical examples that highlight its importance across various sectors.
The 9 cutting-edge tools can aid in achieving high levels of data reliability, ensuring that your data systems are robust, dependable, and trustworthy. Finally, the difference between data reliability and validity, are two crucial concepts in data management, to provide a clearer understanding of their roles in maintaining data integrity.
Remember, in a world increasingly driven by data, the reliability of your data is not just a technical requirement; it’s a cornerstone of trust and efficiency in your operations. By adhering to these steps and utilizing the right tools, you can build a data framework that stands the test of time and change, propelling your organization towards informed decision-making and operational excellence.
What is data reliability: Related reads #
- How to Improve the Reliability of Your Data in 11 Easy Steps
- 11 Proven Strategies for Achieving Enterprise-Scale Data Reliability
- How to Choose a Data Reliability Engineer: 9 Skills to Look For!
- Data Quality Explained: Causes, Detection, and Fixes
- Data Quality Measures: Best Practices to Implement
- What is a Data Catalog? & Why Do You Need One in 2023?
- What is Data Validity & Why is It Crucial for Your Data Teams?
Share this article