Data Quality Measures: Best Practices to Implement
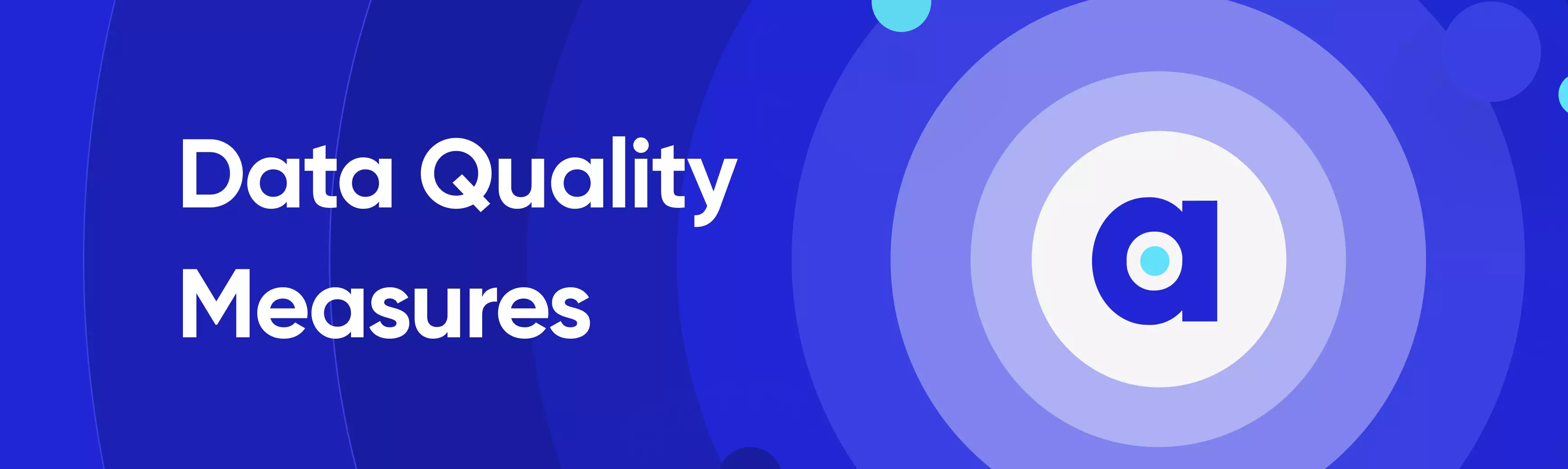
Share this article
Data quality measures are a set of processes and procedures implemented to ensure that data used in an organization is accurate, complete, consistent, and timely.
These measures are designed to improve the reliability of data by addressing errors, inconsistencies, and other issues that may affect its quality.
Modern data problems require modern solutions - Try Atlan, the data catalog of choice for forward-looking data teams! 👉 Book your demo today
Examples of data quality measures include data profiling, data cleansing, data validation, data integration, and data governance. The purpose of data quality measures is to provide high-quality data that can be used to make informed decisions, support business operations, and drive business growth.
Table of contents #
- What are data quality measures and how to measure them?
- 10 Essential data quality measurement tools
- 9 Best practices to implement data quality measures
- What are the challenges of implementing data quality measures?
- Summary
- Data quality measures: Related reads
What are data quality measures and how to measure them? #
Data quality measures are critical in ensuring the integrity, reliability, and usefulness of data in various applications. These measures assess different aspects of the data to ensure it is suitable for its intended use.
Here are some key data quality measures and methods to evaluate them:
- Accuracy
- Completeness
- Consistency
- Timeliness
- Uniqueness
- Validity
- Reliability
- Relevance
- Accessibility
Now, let us look at each of the above aspects in brief:
1. Accuracy #
Refers to the extent to which data is correct, reliable, and free from errors. For example, ensuring that a customer’s address in a database is current and correctly formatted.
How to measure accuracy of data quality?
High accuracy ensures that data closely matches the true or original values. For example, in a customer database, the contact details should accurately represent each customer’s real-life contact information. Mistakes in such data can lead to miscommunication or missed opportunities.
2. Completeness #
Measures if any essential data is missing from the collection. For example, a database record without a zip code might be considered incomplete.
How to measure completeness of data quality?
Completeness ensures that every required piece of data is present. For instance, in a medical dataset, if the age or gender information is missing for a patient, it might affect the analysis or research outcomes.
3. Consistency #
This evaluates whether data is consistent across different data sources or systems. If one database shows a client’s balance as $100 and another one as $150 for the same client at the same time, there’s an inconsistency.
How to measure consistency of data quality?
Data inconsistency can arise when different systems or processes produce conflicting information about the same entity. By ensuring consistency, organizations can avoid confusion and derive coherent insights.
4. Timeliness #
Assesses if data is available when it’s needed and whether it’s up-to-date. For instance, data about last month’s sales might not be considered timely if it’s only available three months later.
How to measure timeliness of data quality?
Timely data ensures that stakeholders have access to the most current and relevant information. For example, stock market data should be near real-time for traders to make informed decisions.
5. Uniqueness #
Refers to the elimination or reduction of duplicate records. For example, ensuring that a customer is not listed twice in a mailing list.
How to measure uniqueness of data quality?
Reducing or eliminating duplicate records helps streamline data processes, improve efficiency, and ensure that insights aren’t skewed by repeated data.
6. Validity #
This involves data being in the proper format, within acceptable ranges, and aligned with predetermined standards. A date field containing “32/01/2023” would be invalid since there’s no 32nd day in a month.
How to measure validity of data quality?
Ensuring validity means that the data follows set formats and standards. For example, a date entry should follow a specific format like “DD/MM/YYYY” and not contain unrealistic values like “32/02/2023”.
7. Reliability #
Reliability refers to the consistency of the data collection process. If the same data is collected multiple times under the same conditions, it should yield the same results.
How to measure reliability of data quality?
Reliable data can be trusted when collected repeatedly under similar conditions. It ensures that the methods used to capture and record data yield consistent results over different periods.
8. Relevance #
Measures the degree to which data meets the current and potential needs of users.
How to measure relevance of data quality?
Data that is pertinent and germane to a specific task or decision-making process adds value. Irrelevant data, on the other hand, can clutter databases and impede analysis.
9. Accessibility #
Ensures that data can be easily accessed and is available to those who need it, but also includes proper access controls to protect sensitive information.
How to measure accessibility of data quality?
Data should be structured and stored in ways that make it readily available for analysis and reporting. However, it’s equally important to have access controls in place to protect sensitive data and ensure privacy.
Organizations often employ data quality tools and frameworks to systematically measure and improve the quality of their data. High-quality data is crucial for making informed decisions, understanding customer behavior, and driving innovations. On the contrary, poor data quality can lead to inaccurate analyses, bad business decisions, and a loss of trust among stakeholders.
10 Essential data quality measurement tools #
Data quality tools are specialized software solutions designed to identify, correct, and prevent data quality issues. They can validate, cleanse, match, and enrich data to ensure it meets the standards required by businesses. The selection of the right tool often depends on the nature and volume of data, as well as the specific business requirements.
Here are a few popular data quality tools:
- Informatica Data Quality (IDQ)
- IBM InfoSphere QualityStage
- Talend Data Quality
- SAS Data Quality
- Trillium Software System
- Microsoft SQL Server Data Quality Services (DQS)
- Oracle Enterprise Data Quality
- Data Ladder DataMatch Enterprise
- Ataccama DQ Analyzer
- Experian Pandora
Let us look into each of the above tools in brief:
1. Informatica Data Quality (IDQ) #
IDQ is a comprehensive suite of applications designed for data quality tasks. IDQ offers profiling, standardization, matching, and enrichment capabilities. It integrates well with other Informatica products and offers a visual interface for data quality logic and rules without requiring extensive coding.
2. IBM InfoSphere QualityStage #
It is part of the IBM InfoSphere Information Server, designed for data cleansing. QualityStage allows users to create and monitor data quality projects with a set of predefined routines and can handle large volumes of data, making it ideal for enterprise-level businesses.
3. Talend Data Quality #
It is an open-source data quality tool that integrates with Talend’s broader data management platform. This tool offers profiling, cleansing, and matching functionalities. Its open-source nature makes it adaptable, and its graphical interface is user-friendly, making data quality tasks easier.
4. SAS Data Quality #
It is a component of the SAS software suite focused on improving data quality. SAS Data Quality can cleanse, standardize, and enrich data. It also integrates seamlessly with other SAS products, benefiting businesses already invested in the SAS ecosystem.
5. Trillium Software System #
It is a data quality solution known for its data profiling, cleansing, and matching features. Trillium offers robust functionalities and is particularly known for its ability to handle complex data scenarios across various industries.
6. Microsoft SQL Server Data Quality Services (DQS) #
It is a SQL Server component that provides data cleansing services. DQS offers data correction, deduplication, and semantic profiling. It’s integrated with SQL Server, making it a convenient option for businesses using the Microsoft stack.
7. Oracle Enterprise Data Quality #
Oracle’s data quality solution that offers profiling, cleansing, and matching. This tool is integrated with other Oracle products, ensuring smooth data operations across the board. Its dashboards are user-friendly and offer deep insights into data quality metrics.
8. Data Ladder DataMatch Enterprise #
It is a tool known for its data matching and deduplication capabilities. DataMatch offers a suite of functionalities like standardization, enrichment, and cleansing, with a strong emphasis on matching and merging data records.
9. Ataccama DQ Analyzer #
It is a free tool that focuses on data profiling and analysis. While it may not have as extensive a feature set as some commercial options, it offers a strong set of profiling features ideal for businesses just beginning their data quality journey.
10. Experian Pandora #
It is a data quality software that provides insights, analytics, and transformation capabilities. Experian Pandora is known for its rapid data profiling and analysis, and its intuitive interface makes data quality management tasks more straightforward.
Choosing the right tool often depends on the specific needs and existing infrastructure of the organization. However, regardless of the tool chosen, a consistent focus on data quality is crucial for business success in an increasingly data-driven world.
9 Best practices to implement data quality measures #
Implementing data quality measures can be a challenging task, but it’s crucial for organizations to ensure reliable and accurate data for making informed decisions.
In this section, we’ll take a closer look at the best practices to implement data quality measures and how they can help organizations establish a data-driven culture.
1. Data governance policy #
- Form a Data Governance Committee with representatives from various departments to oversee the development and enforcement of the policy.
- Define roles and responsibilities related to data management, such as data owners, data stewards, and data custodians.
- Document the processes for data collection, storage, access, sharing, and security.
- Establish guidelines for data privacy and compliance with relevant regulations.
- Regularly review and update the data governance policy to ensure it remains relevant and effective.
2. Data quality framework #
- Identify the key dimensions of data quality for your organization, such as accuracy, completeness, consistency, timeliness, and relevancy.
- Develop a set of data quality metrics and indicators for each dimension.
- Establish data quality goals and targets for each metric.
- Create a process for assessing and monitoring data quality using the defined metrics and indicators.
- Set up regular data quality audits to ensure adherence to the framework.
3. Data stewards #
- Appoint data stewards from various departments or functional areas.
- Define the responsibilities of data stewards, such as identifying data quality issues, resolving them, and ensuring data adheres to the organization’s data governance policy and quality standards.
- Provide training and support to data stewards to help them effectively perform their roles.
- Establish communication channels for data stewards to report on data quality issues and collaborate with other stakeholders.
4. Data quality tools #
- Research and evaluate data quality tools that align with your organization’s needs.
- Implement chosen tools to automate data validation, cleansing, profiling, and enrichment.
- Train employees on the proper usage of these tools.
- Continuously monitor the effectiveness of the tools and make adjustments as needed.
5. Data catalog #
- Identify the key data sources, data sets, and data elements within your organization.
- Collect and document metadata, including data definitions, data lineage, and data sources.
- Develop a centralized, searchable data catalog to store and manage the metadata.
- Regularly update the catalog to reflect any changes in data sources or data elements.
6. Data quality dashboards and reports #
- Identify key data quality metrics to monitor.
- Design and develop data quality dashboards and reports that display these metrics.
- Set up automated processes to regularly update the dashboards and reports with the latest data.
- Share the dashboards and reports with relevant stakeholders to promote transparency and accountability.
7. Training and education #
- Develop training materials and resources on data quality, data governance principles, and best practices for working with data.
- Offer regular training sessions or workshops for employees.
- Encourage participation in external training events, conferences, or webinars to stay up-to-date with industry best practices.
- Include data quality and governance topics in onboarding processes for new employees.
8. Continuous improvement #
- Establish a process for gathering feedback from users, data stewards, and other stakeholders on data quality processes and tools.
- Analyze feedback and identify areas for improvement.
- Implement changes and improvements based on feedback.
- Regularly review and update data quality processes, tools, and metrics to ensure their effectiveness.
9. Collaboration and communication #
- Set up regular meetings or forums for the data curation and modification team to collaborate with other stakeholders.
- Create channels for open communication, such as chat groups, email lists, or shared project spaces.
- Encourage a culture of openness and transparency around data quality issues and initiatives.
- Share data quality successes and challenges with the organization to foster a data-driven culture.
By following this blueprint, your organization can effectively implement data quality measures that result in high-quality, reliable data for decision-making.
What are the challenges of implementing data quality measures? #
Implementing data quality measures can come with several challenges. Some of the most common challenges include:
- Resistance to change
- Inadequate resources
- Lack of clear ownership
- Data silos
- Inconsistent data standards
- Legacy systems and outdated technology
- Data privacy and security concerns
- Complexity of data
- Monitoring and measuring data quality
- Skills gap
Let us look into each of these challenges in brief:
1. Resistance to change #
Employees may be resistant to changes in their workflows or processes, especially if they perceive the new data quality measures as burdensome or time-consuming. It’s important to communicate the benefits of improved data quality and involve employees in the decision-making process to foster buy-in and commitment.
2. Inadequate resources #
Implementing data quality measures may require additional resources, such as personnel, tools, and training. Securing the necessary budget and resources can be challenging, especially in organizations where data quality is not yet a high priority.
3. Lack of clear ownership #
Data quality efforts may suffer from a lack of clear ownership and accountability. It’s important to establish roles and responsibilities for data quality, such as data stewards and data owners, to ensure that everyone knows their part in maintaining high-quality data.
4. Data silos #
Data silos can hinder data quality efforts by making it difficult to access, share, and integrate data across the organization. Overcoming data silos requires collaboration between departments and the implementation of data integration tools and processes.
5. Inconsistent data standards #
Inconsistencies in data standards, such as naming conventions, formats, and units of measurement, can make it difficult to maintain data quality. Developing and enforcing data standards across the organization is essential for ensuring consistency and accuracy.
6. Legacy systems and outdated technology #
Outdated technology and legacy systems can hinder data quality efforts by making it difficult to implement new tools and processes. Upgrading or replacing legacy systems may be necessary to support data quality initiatives effectively.
7. Data privacy and security concerns #
Implementing data quality measures may require increased access to sensitive or personal data, raising concerns about privacy and security. Ensuring compliance with data protection regulations and establishing strong data security measures are crucial in mitigating these concerns.
8. Complexity of data #
The sheer volume, variety, and complexity of data can make it challenging to implement data quality measures. Effective data management, including data cataloging and metadata management, can help organizations better understand and manage their data.
9. Monitoring and measuring data quality #
Identifying and tracking appropriate data quality metrics can be challenging. Regular data quality audits and continuous monitoring are necessary to ensure that data quality measures remain effective over time.
10. Skills gap #
Implementing data quality measures may require specialized skills and expertise that are not readily available within the organization. Investing in training and development or hiring new talent can help address this skills gap.
Addressing these challenges requires a strong commitment to data quality, effective communication and collaboration, and a willingness to invest in the necessary resources and expertise. By anticipating and addressing these challenges, organizations can successfully implement data quality measures and improve the reliability and accuracy of their data.
Summary #
Today, organizations need to ensure high-quality data to make informed decisions. Implementing data quality measures can be daunting, but a blueprint for success involves various components such as data governance policy, data quality framework, data quality tools, data catalog, etc.
While implementing these measures, organizations may encounter several challenges, such as a lack of resources or resistance to change. However, by following a systematic approach and staying committed to continuous improvement, organizations can establish a data-driven culture with reliable and accurate data.
Data quality measures: Related reads #
- How to Improve Data Quality in 10 Actionable Steps?
- How to Ensure Data Quality in Healthcare Data: Best Practices and Key Considerations
- What is Data Governance? Its Importance, Principles & How to Get Started?
- What is Metadata? - Examples, Benefits, and Use Cases
- What Is a Data Catalog? & Why Do You Need One in 2023?
- Is Atlan compatible with data quality tools?
Share this article