Data Quality vs Data Governance: Learn the Differences & Relationships!
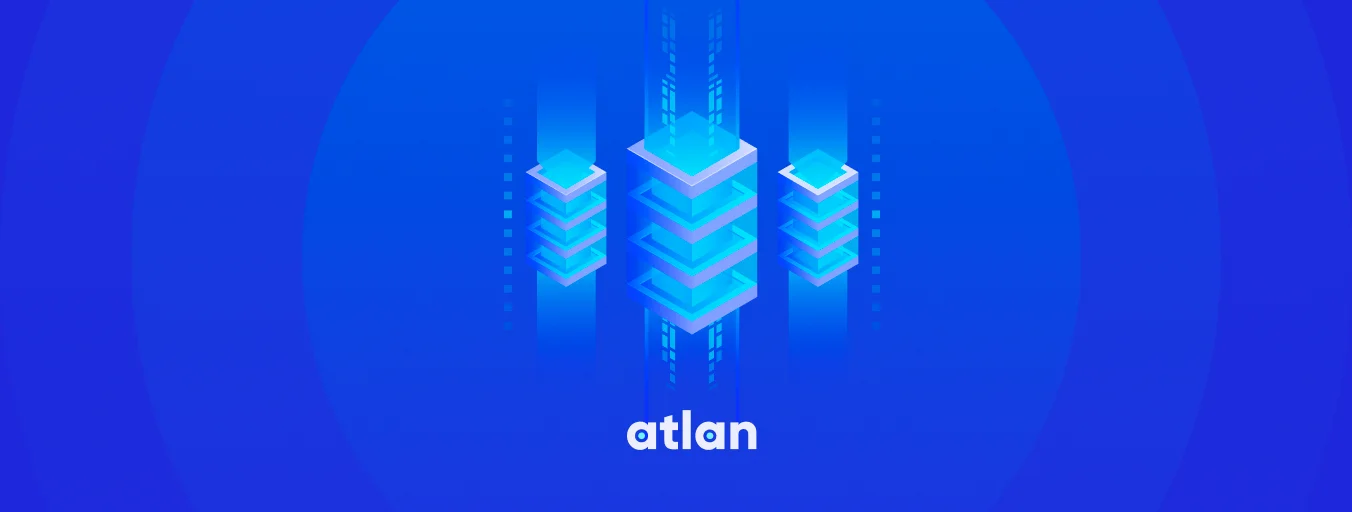
Share this article
Data quality vs. data governance: What’s the difference? #
The main difference between data quality and data governance is that data quality refers to the accuracy, completeness, reliability, and consistency of data, ensuring that it is fit for its intended use, whereas data governance encompasses the overall management, control, and strategic utilization of data assets within an organization.
Inferior data quality not only jeopardizes the accuracy of our insights but also undermines the very foundations of our business strategies.
In response, data governance emerges as the sentinel of integrity, working diligently to establish a harmonious realm where data is nurtured for optimum utilization while vigilantly upholding the bastions of security and privacy.
In this article, let’s understand what the actual difference is between data quality and data governance and why both are essential to organizations.
Table of contents #
- What is data quality?
- What is data governance?
- Data quality vs. data governance: A deep dive
- Data quality vs. data governance: A bird’s-eye view
- Data quality vs. data governance: Why are both non-negotiable?
- Bottom line
- Related reads
What is data quality? #
Data quality refers to the condition of a set of values of qualitative or quantitative variables. It’s the degree to which data is accurate, reliable, and applicable for its intended purpose.
High-quality data can be trusted to be a true representation of the real-world scenario it represents, while low-quality data may lead to misunderstandings, mistakes, and misleading outcomes.
6 Key dimensions of data quality #
Here are six key dimensions of data quality that you need to know:
- Accuracy
The extent to which data is correct, reliable, and free from errors.
Example: If a system records an individual’s age as 20 when they are actually 25, the data is inaccurate.
- Completeness
Ensuring that all the necessary data is present without any omissions.
Example: If a database of customers’ details lacks addresses for some, it is incomplete.
- Timeliness
Data should be up-to-date and available when needed.
Example: If a company’s sales figures are updated annually rather than monthly, they may not reflect the current situation.
- Consistency
Data across the system should not give contradictory information.
Example: If one database lists a customer’s last purchase as January 1st, while another says January 10th, there’s inconsistency.
- Reliability
Data should produce consistent results over time and across different users.
Example: If repeated measurements or inputs give different outputs without any reason, the data source can be considered unreliable.
- Relevance
Data should be pertinent and applicable to the task at hand.
Example: If you’re analyzing data to improve a website’s user experience, page view counts might be relevant, but the CEO’s favorite color probably isn’t.
Data quality is paramount in today’s data-driven world. Whether for decision-making, analytics, or operations, ensuring that data is accurate, complete, timely, and relevant can make the difference between success and failure. Investing in maintaining high data quality can save time, money, and reputational damage in the long run.
What is data governance? #
Data governance is a set of processes, roles, policies, standards, and metrics that ensure the effective and efficient use of information in enabling an organization to achieve its goals.
It involves the orchestration of various roles, responsibilities, and processes to ensure that data is managed as a valuable resource.
5 Key components of data governance #
Here are five key components of data governance that are highly integral:
- Policies and procedures
Guidelines and rules set to define how data should be used, protected, and maintained.
Example: A policy might dictate that personal customer data must be anonymized before analysis.
- Data stewards
Individuals or teams responsible for data quality and the enforcement of data governance policies within specific departments or domains.
Example: A data steward in a sales department would ensure that sales data is accurate, consistent, and used appropriately.
- Data quality
Ensuring the data used and stored is of high quality, accurate, and relevant.
Example: Regular audits of data to identify and rectify inconsistencies or inaccuracies.
- Data lineage
Tracking the flow of data through its lifecycle from creation and storage to usage and archiving.
Example: Mapping out how customer data flows from online forms, through various databases, and into marketing analytics tools.
- Data security
Ensuring that data is protected from unauthorized access, breaches, and theft.
Example: Implementing encryption and access controls on sensitive datasets.
Data governance isn’t just about rules and restrictions. It’s about ensuring that data, one of the most valuable resources in the modern business landscape, is used effectively, safely, and responsibly. Proper data governance can lead to improved decision-making, increased trust in data, and better compliance with industry regulations.
Data quality vs. data governance: A deep dive! #
In the world of data management, the terms “data quality” and “data governance” often come up, and while they are related, they address different aspects of data management. Let’s delve deeper into their meanings, functionalities, and how they interplay with each other.
Data quality is one of the critical components within the larger framework of data governance. While data governance offers the strategies, roles, and tools to manage data, data quality ensures that this data is fit for use.
Without a robust data governance structure, maintaining consistent data quality becomes challenging.
Here are 4 core differences between data quality and data governance
1. Scope #
- Data quality: Focuses specifically on the attributes of the data itself. It ensures the data is correct, complete, and usable.
- Data governance: Has a broader scope, encompassing the roles, responsibilities, processes, and tools involved in the overall management of data.
2. Goal #
- Data quality: Aims to ensure that data sets meet the specific standards set for accuracy, consistency, and relevance.
- Data governance: Aims to provide an organizational framework for data management, ensuring alignment with business goals, regulatory compliance, and efficient data usage.
3. Processes #
- Data quality: Involves data cleansing, data enrichment, validation, and auditing.
- Data governance: Incorporates establishing policies, assigning roles like data stewards, ensuring data security, and defining data lineage.
4. Impact of failure #
- Data quality: Poor data quality can lead to misguided decisions, inefficiencies, reduced trust, and financial losses.
- Data governance: Weak governance can result in regulatory penalties, security breaches, inefficiencies, and organizational chaos related to data management.
Data quality vs. data governance: A bird’s-eye view #
Let’s understand the difference between data quality and data governance in a tabular format.
Attribute | Data Quality | Data Governance |
---|---|---|
Definition | Measures the degree to which data is accurate, reliable, and applicable for its use. | An overarching strategy and set of processes to manage data as a valuable resource. |
Scope | Focuses on the attributes of the data itself. | Encompasses the roles, responsibilities, processes, and tools involved in overall data management. |
Goal | Ensure data meets standards for accuracy, consistency, and relevance. | Provide an organizational framework for data management, aligning with business goals and regulations. |
Processes | Data cleansing, enrichment, validation, auditing. | Establishing policies, assigning roles (e.g., data stewards), ensuring data security, defining data lineage. |
Impact of Failure | Leads to misguided decisions, inefficiencies, reduced trust, financial losses. | Results in regulatory penalties, security breaches, inefficiencies, and organizational chaos. |
Key Components | Accuracy, completeness, consistency, reliability, timeliness. | Policies, procedures, data stewards, data lineage, data security. |
Now, let’s quickly understand why both data quality and data governance are essential for organizations.
Data quality vs. data governance: Why are both non-negotiable? #
While they have distinct roles, the importance of both data quality and data governance cannot be understated:
- Strategic decision-making: High-quality data under a robust governance framework ensures that decisions are made using reliable and accurate information.
- Regulatory compliance: Proper governance helps organizations adhere to data-related regulations, while data quality ensures that the information used and reported is correct.
- Efficiency: With both in place, organizations can avoid redundancies, reduce errors, and streamline data-related operations.
- Trustworthiness: Stakeholders, both internal and external, are more likely to trust data that’s governed well and maintains high quality.
Data quality and data governance are two sides of the same coin. While data quality emphasizes the intrinsic attributes of data, making sure it’s fit for use, data governance offers a holistic framework ensuring that data is managed, secured, and used optimally.
In today’s data-driven landscape, both are indispensable for organizations aiming for success.
Bottom line? #
- In today’s data-driven landscape, the quality of information we rely on has a profound influence on the decisions we make, the insights we extract, and the strategies we formulate.
- Inferior data quality not only jeopardizes the accuracy of our insights but also undermines the very foundations of our business strategies.
- In response, data governance emerges as the sentinel of integrity, working diligently to establish a harmonious realm where data is nurtured for optimum utilization while vigilantly upholding the bastions of security and privacy.
- As two essential pillars of modern data management, both data quality and data governance play vital roles in strategic decision-making, regulatory compliance, operational efficiency, and building trust with stakeholders.
- Together, they create a robust foundation upon which organizations can thrive in the intricate landscape of data-driven success.
Data quality vs data governance: Related reads #
- How to Improve Data Quality in 10 Actionable Steps?
- Data Quality Measures: Best Practices to Implement
- Data Quality in Data Governance: The Crucial Link that Ensures Data Accuracy and Integrity
- 6 Popular Open Source Data Quality Tools in 2023: Overview, Features & Resources
- What is Data Governance? Its Importance, Principles & How to Get Started?
- Data Governance and Its Importance in the Modern Data Stack
- Data Governance Framework — Examples, Templates, Standards, Best practices & How to Create One?
- Snowflake Data Governance — Features, Frameworks & Best practices
- Open Source Data Governance Tools - 7 Best to Consider in 2023
Share this article