How to Build a Robust Data Quality Integrity Framework?
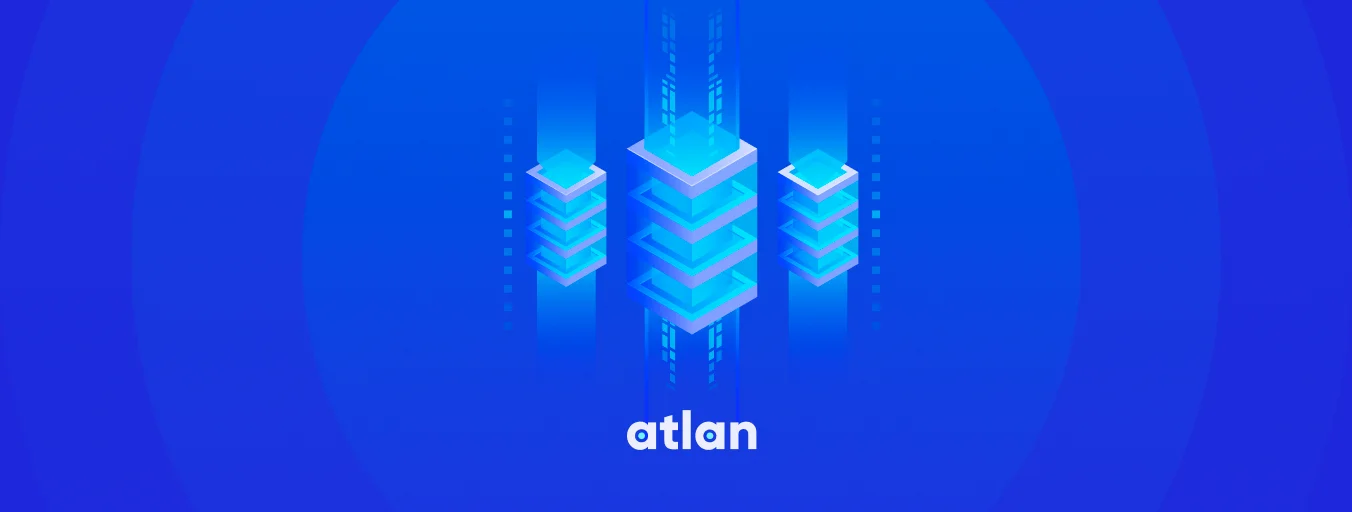
Share this article
Table of contents #
- What is a data quality integrity framework?
- Components of a data quality integrity framework
- How to build a data quality integrity framework?
- Summing up
- Building a data quality integrity framework: Related reads
What is a data quality integrity framework? #
A data quality integrity framework is a structured set of guidelines, policies, and tools designed to ensure the reliability, accuracy, and security of data within an organization. It serves as the bedrock for high-quality data management, providing a consistent methodology to measure, control, and improve data quality across different business units and datasets.
Let us look at the benefits of data quality integrity framework :
- Standardizes data quality
- Ensures compliance
- Facilitates decision-making
- Enhances data security
- Promotes organizational culture
Let us understand these benefits in detail:
1. Standardizes data quality #
A data quality integrity framework serves as the authoritative guide for defining what ‘quality’ means in terms of data within an organization. It sets universal metrics such as accuracy, completeness, consistency, reliability, and timeliness. These metrics create a common language for discussing data quality, making it easier for departments to collaborate.
The standardization also simplifies the process of data integration, especially when merging data from different sources or departments. It ensures that the same yardsticks are being used to measure quality, whether the data is for marketing, finance, or operations.
The framework often incorporates automated validation checks based on these metrics. Standardizing data quality means that when errors or inconsistencies occur, they are easier to identify and rectify.
2. Ensures compliance #
Ensuring compliance is another critical aspect of a data quality integrity framework. The framework usually incorporates specific guidelines to adhere to local, state, federal, and even international regulations regarding data management and privacy, such as GDPR or HIPAA.
Understanding these rules is essential for not just legal safety but also for maintaining consumer trust. The framework will often outline procedures for audits, both internal and external, to ensure ongoing compliance. In regulated industries, demonstrating data quality compliance can be a requirement for licensing and operation.
The framework provides a roadmap for meeting these regulatory standards. Standard templates and documentation processes within the framework facilitate easier compliance verification. Beyond mere legality, a commitment to compliance often improves overall data quality.
3. Facilitates decision-making #
Quality data is the backbone of informed decision-making. A data quality integrity framework ensures that data is accurate, up-to-date, and relevant, enhancing the quality of the insights derived from it. This is particularly important in today’s fast-paced business environment where decisions need to be made quickly and efficiently.
A standardized data quality process means that decision-makers can trust the data they are using. This cuts down the time spent on data verification and allows for more focus on strategic thinking.
Quality data reduces the risk of making decisions based on false or outdated information. Better decisions lead to improved operational efficiencies, cost savings, and competitive advantage.
4. Enhances data security #
Data security is woven into the fabric of a data quality integrity framework. The framework establishes the protocols for secure data storage, access, and transfer. Encryption standards and access control measures are explicitly outlined to protect sensitive information. A robust framework will also include procedures for regular security audits to identify vulnerabilities and implement corrective measures.
Data breaches not only risk exposure of sensitive information but also compromise the integrity and reliability of data sets. Ensuring data security is thus integral to maintaining overall data quality. The framework often integrates with existing it security protocols, providing an additional layer of protection.
Employees are usually trained on data security measures as a part of the framework’s implementation, reducing the risk of breaches due to human error. Data security is an ongoing process, and a robust framework provides the necessary tools for continuous monitoring and improvement.
5. Promotes organizational culture #
A data quality integrity framework is not just a set of technical guidelines; it’s a cultural shift towards treating data as an organizational asset. This promotes accountability and encourages an organization-wide commitment to data stewardship.
Regular training sessions and workshops educate employees about the importance of data quality and its impact on the business. The framework may also include reward systems or KPIs related to data quality to incentivize staff.
A data quality integrity framework is not just a checklist or a set of tools; it’s a holistic approach aimed at instilling a culture of data quality within the organization. Through standardization, compliance, and continuous improvement, it provides a solid foundation for leveraging data as a strategic asset.
Components of a data quality integrity framework #
A data quality integrity framework is not a one-size-fits-all solution; it’s a composite of multiple elements tailored to fit an organization’s specific needs. These components collectively contribute to the effective management, monitoring, and enhancement of data quality across the board.
- Data governance policy
- Data catalog
- Quality metrics and standards
- Data stewards and owners
- Quality control tools and systems
- Monitoring and alerting mechanisms
- Training programs
- Compliance guidelines
- Documentation and record-keeping
- KPIs and success metrics
1. Data governance policy #
A data governance policy is the cornerstone of a data quality integrity framework. It outlines the procedures, responsibilities, and protocols surrounding data management within an organization. This policy standardizes how data should be collected, stored, accessed, and used across different business units. It identifies key stakeholders, from executives to data managers, and their roles in governance.
The policy also includes specific guidelines on data security, privacy, and compliance. It provides a blueprint for the implementation of other framework components like data catalogs and quality metrics. Ultimately, the data governance policy serves as the backbone that aligns all data-related activities with organizational objectives.
2. Data catalog #
A data catalog serves as a centralized inventory of all data assets across the organization. It details the types of data, their sources, and who owns them. This catalog enhances visibility and accessibility of data, facilitating smoother data operations and collaborations.
The data catalog often includes metadata, explaining the context and usage of each data set. It also aids in data lineage, allowing you to trace back to the origins of a particular piece of data. This is crucial for audits and ensuring data quality and integrity. Overall, a data catalog simplifies data management and improves the efficiency of data-related tasks.
3. Quality metrics and standards #
Quality metrics and standards are vital for quantifying data quality. They turn the abstract concept of “quality” into measurable attributes like accuracy, completeness, and timeliness. These metrics serve as benchmarks for assessing the current state of data quality and areas for improvement.
Standardized metrics allow for consistent evaluation across different data sets and departments. They are often automated through quality control tools to provide real-time assessments. These metrics are integral for both internal audits and external compliance checks. Overall, quality metrics and standards provide the yardsticks for continuous data quality improvement.
4. Data stewards and owners #
Data stewards and owners are the custodians of data quality within an organization. They are assigned specific data sets and are responsible for maintaining their quality, security, and usage. These roles create accountability, ensuring that there’s someone responsible for each segment of data.
Stewards and owners work closely with data governance bodies to align their activities with broader organizational goals. They often have the authority to enforce data quality standards and policies within their domain. Their work is essential for the sustained success of a data quality integrity framework.
5. Quality control tools and systems #
Quality control tools and systems are software solutions designed to automate data quality checks. These tools validate, clean, and transform data based on predefined quality metrics and standards. They scan for errors, inconsistencies, and duplicates in real-time or through scheduled checks. Automated alerts notify data stewards or owners when issues arise, enabling prompt corrective actions.
These tools often integrate with existing databases and data processing pipelines for seamless operations. Quality control tools and systems provide the technical backbone for maintaining data integrity, reliability, and accuracy.
6. Monitoring and alerting mechanisms #
Monitoring and alerting mechanisms continuously scan the data landscape to identify quality issues. These mechanisms are often built into quality control tools but can also function independently. They trigger alerts for predefined events, like data anomalies or security breaches, allowing for immediate intervention. This real-time vigilance is crucial for maintaining ongoing data quality and integrity.
Monitoring also provides data for performance metrics, contributing to the ongoing assessment of the framework’s effectiveness. Alerting mechanisms expedite issue resolution, minimizing the impact of data quality issues on operations.
7. Training programs #
Training programs are essential for equipping employees with the skills and knowledge to uphold data quality. These programs cover a range of topics, from the basics of data management to advanced analytics techniques.
Regular training ensures that staff are up-to-date with the latest tools and best practices. It also reinforces the importance of data quality, fostering a culture of data stewardship across the organization.
Training is often tailored to different roles, ensuring relevance and applicability. Employee assessments following the training help gauge its effectiveness and identify areas for improvement. Overall, training programs are an investment in human capital that pays off in sustained data quality.
8. Compliance guidelines #
Compliance guidelines within a data quality integrity framework outline the legal and regulatory obligations related to data management. These guidelines are particularly vital for organizations in heavily regulated industries like healthcare, finance, or energy. They provide a roadmap for aligning data management practices with laws like GDPR, HIPAA, or other industry-specific regulations. Failure to adhere to these guidelines can result in severe penalties, including fines and reputational damage.
Compliance checks are usually integrated into monitoring mechanisms and audited regularly. Adhering to compliance guidelines not only minimizes legal risk but also often enhances overall data quality.
9. Documentation and record-keeping #
Documentation and record-keeping are critical for capturing policies, procedures, and data quality reports. This documentation serves multiple purposes, including internal reviews, external audits, and historical reference. It enables organizations to demonstrate compliance with regulatory guidelines.
Good documentation practices also make it easier to onboard new employees, providing them with a solid understanding of the data landscape. Records of data quality checks and resolutions serve as a historical log, helping to identify recurring issues or trends. Thus, proper documentation and record-keeping are instrumental for both accountability and continuous improvement.
10. KPIs and success metrics #
Key performance indicators (KPIs) and success metrics measure the effectiveness of a data quality integrity framework. These metrics can range from the accuracy and completeness of data to the time taken to resolve data quality issues. Monitoring these kpis provides ongoing insights into the framework’s performance.
It also helps in identifying areas that may require adjustments or improvements. These metrics are often shared with key stakeholders, including executives and data governance boards, to keep them informed. By measuring against KPIs and success metrics, an organization can quantify the ROIs of their data quality efforts, guiding future strategy and investments.
A comprehensive data quality integrity framework is an intricate web of multiple components that work in synergy. Understanding these components is the first step in constructing a robust framework that not only solves immediate quality issues but also establishes a sustainable model for long-term data excellence.
How to build a data quality integrity framework? #
In the era of data-driven decisions, ensuring data quality is crucial. A data quality integrity framework serves as a blueprint for maintaining and improving the caliber of your data. It’s not just about cleaning data but about establishing a robust ecosystem where data can be trusted, understood, and effectively utilized.
- Conduct data audit
- Define quality metrics
- Identify data owners
- Implement data governance
- Develop quality control mechanisms
- Conduct regular quality checks
- Train and educate staff
- Continuous monitoring and improvement
- Compliance and documentation
- Measure success metrics
Let us understand them in detail:
1. Conduct data audit #
A data audit is the initial examination of all data sources, types, and storage systems in an organization. This step serves as a diagnostic tool, providing a snapshot of the current data landscape.
It identifies data inconsistencies, redundancies, and gaps. During an audit, the data’s origin, transformation processes, and end-usage are mapped. This helps in setting a baseline for data quality and understanding which areas need immediate attention.
2. Define quality metrics #
Quality metrics are standardized measures that help gauge the quality of data. These metrics can vary but often include factors like accuracy, completeness, timeliness, and relevance.
Setting these metrics provides a uniform way to assess data and ensures that everyone in the organization has the same understanding of what ‘high-quality data’ means. This becomes the criteria against which all data is evaluated.
3. Identify data owners #
Data ownership means assigning specific individuals or teams as custodians for different sets of data. These owners are responsible for the quality, maintenance, and safe storage of the data.
They act as the go-to persons for any questions, changes, or issues related to that particular data set. This centralization of responsibility ensures that there’s accountability in maintaining data quality.
4. Implement data governance #
Data governance involves establishing policies, roles, and responsibilities related to data management. It serves as the overarching structure that dictates how data is acquired, stored, processed, and shared within the organization.
Effective governance aligns data management with business objectives and ensures that data quality is not compromised at any stage.
5. Develop quality control mechanisms #
Quality control mechanisms are automated or semi-automated systems integrated into the data lifecycle for validation and error correction.
They can include things like data cleansing tools, validation checks, and anomaly detection algorithms. These mechanisms work in real-time or batches to ensure that incoming and existing data conform to the set quality metrics.
6. Conduct regular quality checks #
Regular quality checks involve periodic evaluations of data against the defined quality metrics. This ensures that the data stays reliable over time and adjusts to new business needs or market changes. These checks can be scheduled monthly, quarterly, or yearly and are crucial for maintaining long-term data integrity.
7. Train and educate staff #
Staff training and education on data quality involve workshops, seminars, or training sessions that educate employees about the significance of maintaining high data quality. It equips them with the skills needed to detect issues and enforce quality control, turning data quality maintenance into a collective organizational habit.
8. Continuous monitoring and improvement #
Continuous monitoring means consistently evaluating the performance of your data quality framework. This includes real-time alerts for anomalies and periodic reviews for strategic improvement.
The aim is to not just maintain but continuously improve data quality, adapting to new data sources, technologies, and business requirements.
9. Compliance and documentation #
Compliance ensures that your data management processes are in line with local, national, and international regulations like GDPR or HIPAA.
Documentation includes maintaining records of all data quality processes, audits, and metrics. This not only aids in compliance but also serves as a resource for internal reviews and potential audits.
10. Measure success metrics #
Success metrics are key performance indicators (KPIs) that help evaluate the effectiveness of the data quality framework. These could range from error rates in data to the time saved in data processing due to high-quality data.
Monitoring these kpis provides insights into the roi of your data quality initiatives and guides future strategies.
Building a data quality integrity framework is an ongoing process that involves multiple stakeholders and continuous improvement. It’s not just a one-time effort but a cultural shift towards respecting data as a valuable asset. By implementing a comprehensive framework, organizations can significantly enhance decision-making, compliance, and operational efficiency.
Summing up #
A robust data quality integrity framework is the foundation for leveraging data as a strategic asset. By standardizing quality metrics, strengthening governance, implementing automated checks, and promoting a culture of quality, organizations can drive better decision making.
Building a data quality integrity framework: Related reads #
- Data Quality Measures: Best Practices to Implement
- Data Quality Explained: Causes, Detection, and Fixes
- Benefits of Moving On-Premise to Cloud: Empowering Your Business for Success
- How To Improve Data Quality In 12 Actionable Steps?
- Data Quality Fundamentals: Why It Matters in 2023!
Share this article