Data Quality Fundamentals: Why It Matters in 2024!
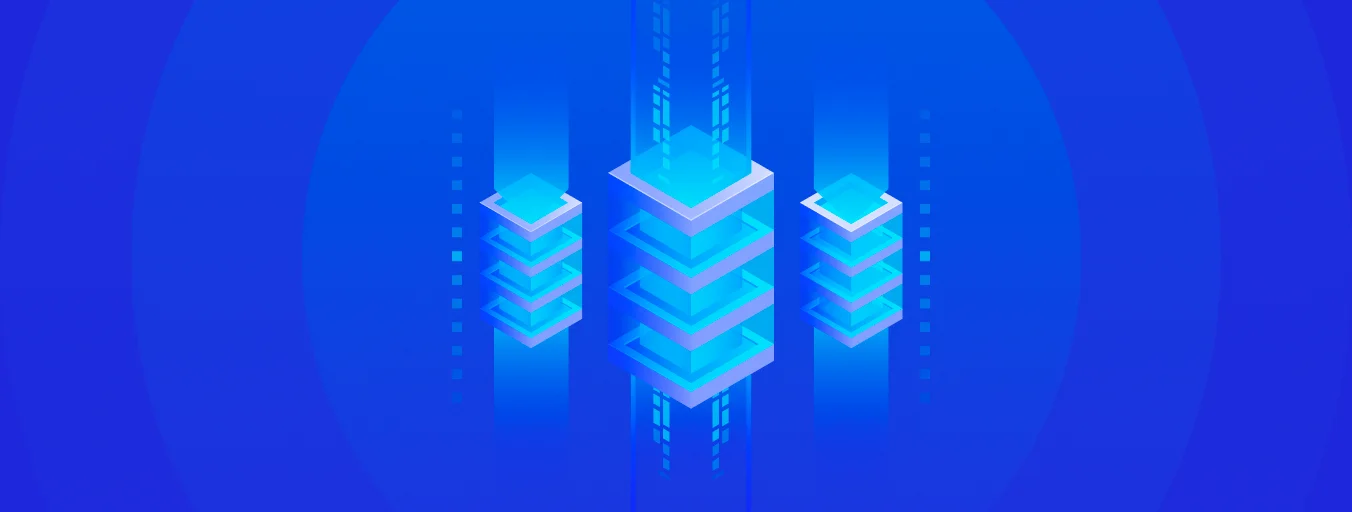
Share this article
In business, government, healthcare, and numerous other sectors, decisions are increasingly data-driven. When the data is of high quality – accurate, complete, timely, consistent, and relevant – it ensures that decision-makers have reliable information. This leads to more effective strategies, better resource allocation, and improved outcomes.
The fundamentals of data quality refer to the accuracy, completeness, reliability, relevance, and timeliness of data.
Modern data problems require modern solutions - Try Atlan, the data catalog of choice for forward-looking data teams! 👉 Book your demo today
From its foundational principles to its wide-ranging impact on organizational success, data quality shapes the very core of effective business strategies. Moreover, recognizing when and how to initiate qualitative data analysis is a critical consideration that can significantly influence research outcomes.
In this comprehensive exploration, we delve into the fundamentals of data quality, its profound organizational impact, and the strategic art of timing qualitative data analysis.
Table of contents #
- What is data quality fundamentals?
- What are the values of data quality fundamentals?
- What is the impact of data quality in an organization?
- 10 Data quality challenges you can face
- How to measure your data quality: 11 Tips to follow
- O’Reilly’s data quality fundamentals: Maintaining high-quality data systems
- Summing up
- Related reads
What is data quality fundamentals? #
Data quality fundamentals include factors such as accuracy, completeness, consistency, reliability, and timeliness. Essentially, good data quality means that the data is fit for its intended uses in operations, decision-making, and planning.
Understanding the fundamentals of data quality can provide a solid foundation for any data-driven initiative.
Here are some key data quality fundamentals:
- Dimensions of data quality
- Importance of data governance
- Data stewardship
- Quality assurance processes
- Data cleansing
- Monitoring and auditing
- Compliance and security
- User feedback loop
- Cost of poor data quality
- Data quality tools
- Integration with business processes
Let us understand each of them in detail:
1. Dimensions of data quality #
The various dimensions that are used to evaluate data quality include:
- Accuracy: Does the data correctly represent the real-world objects or events?
- Completeness: Is all necessary data available?
- Consistency: Is the same data uniformly represented across different systems and datasets?
- Timeliness: Is the data up-to-date and available when needed?
- Uniqueness: Are there duplicate records, and if so, have they been identified and managed?
2. Importance of data governance #
Data governance involves the establishment of policies, procedures, and responsibilities that govern the management of data, including its quality. A well-defined governance model is crucial for maintaining high data quality standards.
3. Data stewardship #
Data stewards are responsible for maintaining the quality of data in an organization. They define rules, create metadata, and monitor data usage, thereby ensuring that the data remains accurate, complete, and secure.
4. Quality assurance processes #
Regularly running data quality checks using automated or semi-automated tools can help identify and fix issues before they become serious problems. This includes validation rules, consistency checks, and data profiling.
5. Data cleansing #
Data cleansing involves the identification and correction or removal of errors and inconsistencies in data to improve its quality. This is often done during ETL (Extract, Transform, Load) processes but can also be a dedicated operation.
6. Monitoring and auditing #
Continuous monitoring and auditing are essential for maintaining high data quality. Key performance indicators (KPIs) and metrics should be defined and monitored to ensure that data quality objectives are being met.
7. Compliance and security #
Data quality is not just about the content; it’s also about ensuring that the data complies with legal and business policies, including security measures to protect sensitive data.
8. User feedback loop #
User feedback is a valuable resource for assessing data quality. End-users often encounter data quality issues that are not immediately obvious through automated checks. Their feedback can be used to make continuous improvements.
9. Cost of poor data quality #
Poor data quality can have significant financial impact through incorrect decisions, inefficiencies, and loss of customer trust. Understanding this cost can help in justifying the investments needed for data quality initiatives.
10. Data quality tools #
Various software tools are available for managing data quality. These tools can automate many aspects of data quality management, from data profiling to cleansing to monitoring.
11. Integration with business processes #
Data quality is not a stand-alone activity but should be integrated into daily business processes and decision-making. This ensures that high-quality data is used at all touchpoints, from operational tasks to strategic planning.
Understanding these fundamentals provides the groundwork for implementing a robust data quality management program. High-quality data is essential for analytics, machine learning, reporting, and ultimately, making informed business decisions.
What are the values of data quality fundamentals? #
The values of data quality fundamentals are crucial aspects that ensure the usability and reliability of data in various applications. Here’s a detailed explanation of each value:
1. Accuracy #
This refers to the correctness and precision of the data. Accurate data correctly represents the real-world values or conditions it is meant to depict. Inaccurate data can lead to erroneous conclusions and decisions.
For example, in a medical context, accurate patient data is critical for proper diagnosis and treatment.
2. Completeness #
Completeness is about having all the necessary data without missing elements. Incomplete data can lead to biased analysis and conclusions.
For instance, a customer database missing key contact information would be less effective for marketing purposes.
3. Reliability #
This value speaks to the consistency and dependability of the data over time. Reliable data can be trusted to give consistent results under similar conditions.
Inconsistent data, which varies unpredictably, can undermine confidence in decision-making processes.
4. Relevance #
Relevance involves the data being appropriate and applicable for the intended purpose. Data should be relevant to the context in which it is being used.
For example, in market analysis, data about current consumer trends is more relevant than outdated information.
5. Timeliness #
Timely data is available when it is needed and reflects the most current situation. The value of data often diminishes over time; hence, having up-to-date information is crucial for decision-making.
For example, real-time traffic data is essential for route planning applications.
Each of these values plays a critical role in ensuring that data is a useful and trustworthy asset. High-quality data leads to better decision-making, efficient processes, and can provide a significant competitive advantage in various fields. Conversely, poor data quality can lead to misinformed decisions, inefficiencies, and potential financial losses.
What is the impact of data quality in an organization? #
Data quality is more than just a technical issue; it has far-reaching implications on a corporation’s health, reputation, and bottom line. As corporations increasingly rely on data-driven strategies, the impact of data quality reverberates through various aspects of business.
- Financial performance
- Strategic planning
- Risk management
- Employee productivity
- Market positioning
- Stakeholder relations
Let us look at them in detail:
1. Financial performance #
Poor data quality can significantly impact a corporation’s finances. Erroneous data can lead to faulty decision-making, resulting in ineffective budget allocation, missed revenue opportunities, and increased operational costs. On the flip side, high-quality data can optimize financial performance by driving accurate forecasting and targeted investment.
2. Strategic planning #
High-quality data plays a critical role in formulating corporate strategies. From market analysis to customer segmentation, data informs various elements of strategic planning. Poor data quality can misguide strategy, potentially leading to investments in unprofitable ventures or neglecting lucrative opportunities.
3. Risk management #
Accurate data is crucial for effective risk assessment and management. Inaccurate or inconsistent data can underestimate or overlook risks, leaving the corporation vulnerable to financial instability or reputational damage. Conversely, high-quality data helps in accurate risk modeling, allowing for better preparedness and response mechanisms.
4. Employee productivity #
Low-quality data can be a time sink for employees who have to correct errors, reconcile inconsistencies, or search for missing information. This decreases productivity and increases employee frustration. High-quality data enables smooth operations and frees employees to focus on more value-added tasks.
5. Market positioning #
Data quality impacts how a corporation is perceived in the marketplace. High-quality, reliable data can provide insights into market trends, customer preferences, and competitive landscapes, allowing a corporation to position itself advantageously. Poor data quality can result in missed market opportunities and ineffective marketing strategies.
6. Stakeholder relations #
The quality of data a corporation holds affects its relationships with various stakeholders, including investors, suppliers, and customers. High-quality data builds trust and transparency, crucial for long-term partnerships and customer loyalty. On the other hand, poor data quality can erode trust and potentially lead to legal complications.
10 Data quality challenges you can face #
Data quality management presents various challenges, each with its own intricacies and potential impact on business operations and decision-making. Below are 10 data quality challenges:
1. Data inconsistency across systems #
In larger organizations with multiple data sources like CRMs, ERPs, and databases, inconsistencies often arise. For example, one system may record addresses differently from another, causing confusion and leading to issues in data integration and analytics.
2. Incomplete data #
Missing fields or incomplete records can severely hamper analytics and decision-making. The absence of key pieces of data might lead to incorrect conclusions, causing enterprises to miss out on opportunities or make suboptimal decisions.
3. Duplicate records #
Duplicated data entries can inflate numbers, leading to inaccurate reporting and insights. For instance, duplicate customer records in a CRM system can cause overestimation of customer count, affecting marketing strategies.
4. Real-time data quality management #
As businesses increasingly rely on real-time analytics, the ability to manage data quality in real-time becomes essential. This presents a challenge in terms of resources and technology to continuously validate, clean, and enrich data as it flows into systems.
5. Lack of data governance #
Without a formal data governance model, there is no accountability for data quality. The absence of standardized policies and procedures can lead to ad hoc data management practices, resulting in poor data quality.
6. Human error #
Despite automation and advanced data capture technologies, human intervention is still a significant factor in data entry and management. Errors made during data entry, coding, or transferring can introduce inaccuracies that are often hard to trace and correct.
7. Complexity of data structures #
The increasing complexity of data, especially with the advent of big data and unstructured data types like text, images, and videos, adds another layer of difficulty to maintaining data quality. Traditional data quality tools may not be equipped to handle this complexity.
8. Data security and compliance #
Data quality isn’t just about the accuracy or completeness of data but also about ensuring its security and compliance with regulations like GDPR or CCPA. Failure to comply can result in legal repercussions and loss of customer trust.
9. Legacy systems #
Older systems may not be equipped to handle modern data quality requirements. They can be difficult to update or integrate with newer systems, leading to isolated data silos with poor data quality.
10. Scalability #
As businesses grow, the volume of data also increases, adding stress to existing data management systems. Scalability becomes a challenge, as the tools and processes that worked for smaller datasets may not be sufficient for larger ones.
Addressing these challenges often requires a multi-disciplinary approach, combining technology, governance models, and human expertise. By understanding these challenges in detail, organizations can better prepare for them, ensuring that their data quality management efforts are more effective and robust.
How to measure your data quality: 11 Tips to follow #
Measuring data quality is a crucial step in ensuring that the data you’re using for decision-making, analytics, and operational activities is accurate, reliable, and timely. A structured approach to measuring data quality can involve several methodologies, frameworks, and tools. Here’s how to go about it:
- Define data quality metrics
- Establish a baseline
- Create a data quality scorecard
- Use data profiling tools
- Perform data audits
- Implement validation rules
- User feedback
- Conduct reconciliation checks
- Historical trend analysis
- Cost analysis
- Benchmark against industry standards
Let us explain each of them in detail:
1. Define data quality metrics #
The first step in measuring data quality is to define the metrics or dimensions against which you’ll evaluate the data. Common dimensions include accuracy, completeness, consistency, timeliness, uniqueness, and validity.
2. Establish a baseline #
Before you can measure improvements or identify issues, you need to establish a baseline. This means capturing the current state of your data for each metric. This could involve complex queries, statistical methods, or specialized data quality tools.
3. Create a data quality scorecard #
A scorecard provides an at-a-glance view of data quality across various dimensions. This is usually a document or dashboard that aggregates the measurements from multiple data quality checks, often rated on a scale for easy interpretation.
4. Use data profiling tools #
Data profiling tools analyze a dataset to identify anomalies like missing values, duplicates, or outliers. These tools can give you a quick, automated overview of data quality and can be integrated into data pipelines for real-time assessment.
5. Perform data audits #
A data audit is a more manual, in-depth review of a dataset. It involves checking samples of data for quality issues and is usually done at regular intervals. This is particularly important for highly sensitive or regulated data.
6. Implement validation rules #
Validation rules are automated checks that can be implemented in your data entry or data integration layers to catch issues in real-time. For example, a validation rule could ensure that email fields contain a valid email format.
7. User feedback #
The end-users of data are often the first to notice quality issues. Implement a system where users can easily flag suspect data and provide feedback, then feed this information back into your quality measurement process.
8. Conduct reconciliation checks #
These are checks to ensure that data is consistent across different systems or datasets. For example, the total sales figures in your CRM should match those in your accounting software.
9. Historical trend analysis #
Tracking the quality of data over time can provide insights into the efficacy of your data management efforts. If your data quality is improving, your current strategies are probably effective. If not, it’s a cue to investigate.
10. Cost analysis #
Measuring the financial impact of poor data quality can be an eye-opener. This could include the cost of incorrect decisions made based on bad data, lost opportunities, or the manual effort required to clean data.
11. Benchmark against industry standards #
Finally, compare your data quality metrics against industry standards or best practices to see how you fare against peers or guidelines.
By systematically measuring data quality across various dimensions, using both automated and manual checks, and involving end-users in the process, organizations can gain a comprehensive view of their data’s reliability. This not only helps in improving existing processes but also instills greater confidence in data-driven decision-making.
O’Reilly’s data quality fundamentals: Maintaining high-quality data systems #
“O’Reilly’s Data Quality Fundamentals” is a comprehensive guide that delves deeply into the various aspects of building and maintaining high-quality data systems. The book is structured to provide both a theoretical understanding and practical insights into the field of data quality.
It begins by highlighting the importance of data quality in the current technological landscape, discussing the concept of “data downtime” and its parallels with site reliability engineering principles. This sets the stage for applying similar principles to data engineering workflows.
Further chapters focus on building resilient data systems, addressing data quality across key data pipeline technologies such as data warehouses, lakes, and catalogs. The book also emphasizes proactive anomaly detection and monitoring, offering a tutorial on building a data quality monitor.
Finally, the book concludes with a tangible calculation for measuring the financial impact of poor data on businesses and predictions for the future of data quality about broader industry trends like distributed data management and the rise of data lake houses.
Overall, “O’Reilly’s Data Quality Fundamentals” is a valuable resource for data professionals, providing both a theoretical foundation and practical advice for building trustworthy data pipelines and addressing data quality issues at scale.
Summing up #
In today’s increasingly data-dependent corporate landscape, data quality is not a luxury but a necessity. From financial performance to stakeholder relations, the quality of data a corporation maintains can be a decisive factor in its success or failure.
Ensuring data quality, therefore, should be a strategic priority for any forward-thinking corporation.
Data quality fundamentals: Related reads #
- 6 Popular Open Source Data Quality Tools To Know in 2024: Overview, Features & Resources
- Data Quality Measures: Best Practices to Implement
- How to Ensure Data Quality in Healthcare Data: Best Practices and Key Considerations
- Automated quality control of data pipelines
- Data Management 101: Four Things Every Human of Data Should Know
- 9 Components to Build the Best Data Quality Framework
- How To Improve Data Quality In 12 Actionable Steps?
- Gartner Magic Quadrant for Data Quality: Overview, Capabilities, Criteria
- What is Data Integrity and Why Should It Be a Priority of Every Data Team?
Share this article