5 Steps to Evaluate a Data Catalog: A Foundation for Data Collaboration
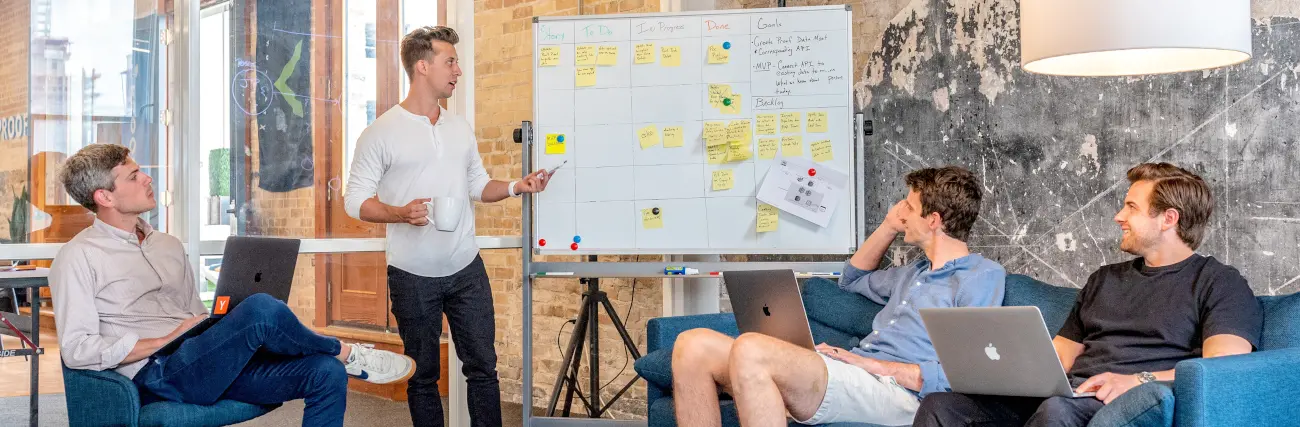
Share this article
Quick answer:
TL;DR? Here’s a 2-minute summary of the contents of this article and what you should expect from it:
- Evaluating a data catalog starts by:
- Understanding your needs and resource availability
- Developing essential criteria for your use cases
- This approach helps you assess and compare the available solutions and set up proofs of concept (POCs).
- In this article, we’ll look into the 5 steps to evaluating a data catalog, along with examples and best practices.
Five steps to evaluate a data catalog #
Evaluating a data catalog can be tricky. There are lots of options on the market. If you’re just starting down this path, you may not have a lot of direction on how to choose a data catalog…so here are five steps to help guide you in that decision:
- Identify your organizational needs and budget
- Creating evaluation criteria
- Understand the providers and offerings in the market
- Shortlist and demo the prospective solutions
- Execute proofs of concept (POCs)
Let’s consider each of these steps in detail.
Start by identifying your organizational needs and budget. #
It’s important to consider the reasons data initiatives are not reaching their full potential in order to identify the functionality that will be most useful. Here are some common roadblocks you might be facing:
- Constant back-and-forths between business users and data engineers as they attempt to define the meaning of variables (siloed business domain knowledge).
- Business users have to ask data engineers whenever they need a new report to be run(53% of global business and IT professionals say empowering users with self-service functionality is an essential step to improve their organization’s business intelligence and analytics, according to HBR).
- Data teams build reports but other employees are unaware of them (data is a black box).
- Data goes through complicated ETL processes before appearing in business intelligence tools, making it nearly impossible to track and control sensitive information for compliance purposes (poor data governance).
Once you’ve identified the primary areas for improvement, connect them to the core functionality you should expect from a data catalog in 2024.
a) Discovery
A data catalog provides a clear and comprehensive view of all data sets within the organization. It should include sophisticated search options that allow you to perform Google-like searches based on keywords or context.
“Recent research by IBM shows that businesses spend 70% of their time looking for data and only 30% of the time utilizing it.” - Data Science Central
b) Knowledge
Within a data catalog, you should be able to find context, information, and business know-how around each data asset. This metadata should cover the 5W’s and 1H framework to ensure any user can understand everything they need to know about a data asset without contacting its owner or other collaborators.
c) Trust
One of the prerequisites for self-service data culture is trust. Business users need to feel they can trust data so they can use it to inform decisions with confidence. Capabilities like manual verification tracking, automated anomaly checks, and data lineage visualizations help data managers ensure data is clean, accurate, and up to date so users can rely on it.
d) Collaboration
While collaborating as a data team requires those soft, human skills — active listening, proactive communication, etc. — a data catalog provides a foundation for collaboration with features like an intuitive user interface (UI), the ability to add tribal knowledge with data tagging, and embedded collaboration that unifies workflows within the tool itself.
e) Governance
Regulations such as GDPR are forcing organizations to closely control how they store and use data, but data governance goes beyond compliance. Robust governance ensures team members across the organization have the ability to use data while controlling access at a granular level. Look for capabilities like user-defined rules for storage or deletion, a single dashboard for access management, and policy enforcement through data lineage.
f) Security
The value of data means it also needs to be secured against unauthorized breaches. Much of this security happens at the level of data storage and warehousing, but the data catalog should protect data itself through access controls and the use of techniques like data masking to ensure any sensitive data remains protected. Look for industry standard certifications like SOC 2 to ensure your data catalog employs the same security measures you’d expect in the rest of your data stack.
“By 2023, organizations that promote data sharing will outperform their peers on most business value metrics.” - Gartner
Create your evaluation criteria #
At this point, it’s great to prioritize requirements to ensure the data catalog you’re evaluating meets your core needs. Consider rating each category of core functionality on a scale of 1 (highest priority) to 3 (lowest priority). Is there any functionality that is mandatory? If you work with sensitive data in a highly regulated environment, for example, security will clearly be a top priority. A shipping company might prioritize the ability to share tribal knowledge with metadata so users across physical locations can understand each data asset.
It’s also important to consider other requirements such as integrations, onboarding time, and the product pricing model. An organization with a collection of on-premise data centers will have different requirements than a distributed, remote-first company, for example. If you use tools like Jupyter, Microsoft Excel, Tableau, or Power BI, it will be necessary to ensure the data catalog has pre-built integrations with each tool in your stack.
Understand the providers and offerings in the market #
Historically speaking, metadata has been around for thousands of years, such as with the cataloging system at the Library of Alexandria. The current way of thinking about data catalogs emerged in the late 20th century with the innovation made possible by the internet. It has slowly evolved into what we know as modern data catalogs. As a general framework we can think of their evolution in terms of three generations of data catalogs:
Traditional Data Catalogs #
These are defined by their on-premise and legacy nature. They’re optimized for on-premise deployments and designed to be run by IT professionals. As such, they are challenging for business users, and they are limited in their capabilities for cloud-based storage. Traditional data catalogs are ideal for large companies with a robust IT team that are operating on-premise infrastructure. Because IT resources are available, challenges with business users are easier to mitigate.
“The metadata and semantic data integration visionaries of 20 or 30 years ago — who toiled in frustration due to the limitations of the data management systems of their era — would be excited by the potential of today’s technologies.” - David Stodder, TDWI
Open Source Catalogs #
These cloud-native catalogs were developed by companies to solve internal challenges, then open-sourced for external teams. They are built by engineers, for engineers, and require significant time and resources to develop into a functioning data catalog for your organization, but they are free of licensing costs, come with documentation, and allow you to build on work others have done.
Modern Data Catalogs #
These are flexible, comprehensive catalogs that are designed for business users working in a cloud-native environment. They are ideal for distributed, digital- and remote-first businesses that wish to make it easy for non-technical business team members to use the data effectively. Their extensible nature means they can connect all types of data assets in a single source of truth, intelligently leverage metadata as a form of big data, and integrate seamlessly into data consumers’ current workflows. Modular and scalable pricing models are characteristic of modern catalogs, but they are not optimized for on-premise data sources.
Shortlist and demo prospective solutions #
After researching and identifying data catalog service providers in the market, set up deep dive demos to find which provider best meets your needs. Here are some best practices for setting up a successful demo:
- Help providers understand your needs by sharing your challenges and organizational context. This gives a great starting point to keep your demo focused and relevant.
- Bring different user personas to the demo. Ensure you have stakeholders from each team on hand so you can gather the most comprehensive feedback.
- Check your data architecture is compatible. It’s critical that the platform works with both your current architecture as well as where you’re going over the next few years.
Execute Proofs of Concept (POC) to carry out hands-on tests #
Before the proof of concept (POC), identify the top use cases you want to test, prepare the test architecture, and onboard business users that will be involved. When you’re carrying out the POC, ensure you focus on execution and capture detailed feedback at each stage. Finally, set up calls with the service provider to share feedback on your experience and gauge their response.
What is the importance of a data catalog? #
Data catalogs are valuable because they help tap into the value of raw data stored in data lakes. In particular, they help address the following:
- Empowering users to engage with data in unique ways
- Enabling business users to find and access the right dataset
- Building organizational trust in the quality and accuracy of data
- Providing context for the data team use to make decisions
“Data lakes need proper governance, operationalization and data catalogs to facilitate data consumption." - Gartner
Data catalogs play a key role in the modern data stack. It’s important to carefully select a data catalog that addresses your organization’s specific requirements and needs. Interested in taking a deeper dive into evaluating a data catalog? Head over here to learn more. Read The Ultimate Guide to Evaluating a Data Catalog.
If you are evaluating a data catalog solution for your team, do take Atlan for a spin — Atlan is a 3rd generation data catalog built on the premise of embedded collaboration that is key in today’s modern workplace, borrowing principles from GitHub, Figma, Slack, Notion, Superhuman, and other modern tools that are commonplace today.
Share this article