Data Lineage in Data Governance: Unlocking the Potential of Modern Data Landscapes With Comprehensive Data Tracking
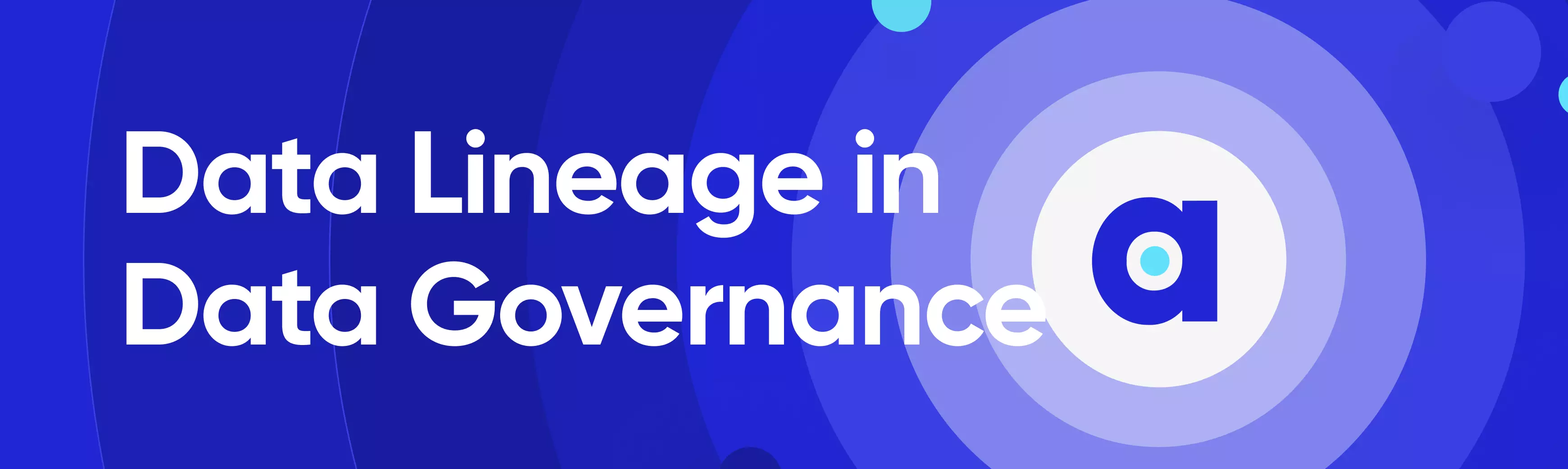
Share this article
Why is data lineage important for data governance? #
Data lineage is closely connected to data governance as a vital component in managing an organization’s data ecosystem. It bolsters data governance by providing transparency and traceability in data movement and transformations, ensuring data quality, and supporting compliance with regulations. Essentially, data lineage serves as one of the foundations for effective data governance.
In this article, we will delve into the significance of data lineage within data governance and its connection to various crucial aspects.
Table of contents #
- Why is data lineage important for data governance?
- Transparency and traceability
- Data quality
- Compliance and regulatory requirements
- Impact analysis
- Root cause analysis
- Collaboration and communication
- Data lineage for data governance: How to evaluate the right solution
- Data lineage in data governance: Related reads
Understanding the importance of data lineage in data governance can be best approached by considering the following key aspects:
- Transparency and traceability
- Data quality
- Compliance and regulatory requirements
- Impact analysis
- Root cause analysis
- Collaboration and communication
- Supporting advanced data initiatives
Transparency and traceability #
Real-life example: Imagine a grocery store that regularly updates its inventory data, including product details, prices, and stock levels. To keep customers satisfied and maintain efficient operations, the store must trace all inventory information back to its source.
Why is data lineage the solution? #
Data lineage creates a comprehensive map of how inventory data travels and transforms within the store’s system, helping data users in the stores track changes, understand relationships between data elements, and identify errors or inconsistencies. This ensures transparency and traceability across the organization.
Data lineage mandates #
Utilizing data lineage tools that offer complete visibility, maintain an audit trail, and automate data mapping procedures aligns with data governance best practices.
Find more about trust and transparency in the data governance framework here
Data quality #
Real-life example: A healthcare organization collects and processes patient data from multiple sources. Inaccurate or inconsistent data can lead to misdiagnosis or improper treatment.
Why is data lineage the solution? #
Data lineage helps organizations identify data quality issues by tracking data transformations and validating data accuracy throughout the data lifecycle.
Data lineage mandates #
Employing data lineage solutions that detect and resolve data quality issues while enforcing data validation rules adhere to data governance best practices.
Learn more about data validation rules and data governance best practices here
Compliance and regulatory requirements #
Real-life example: Companies that handle sensitive customer information must adhere to data protection regulations, such as the General Data Protection Regulation or HIPAA, to avoid hefty fines and reputational damage.
Why is data lineage the solution? #
Data lineage enables organizations to demonstrate compliance by providing a detailed audit trail of data processing activities and maintaining proper documentation.
Data lineage mandates #
Implementing data lineage tools that support regulatory frameworks and ensure adherence to data privacy and security guidelines comply with data governance best practices.
Read more about data governance and compliance here
Impact analysis #
Real-life example: A retail business wants to update its data processing pipeline, which could affect systems such as inventory tracking, sales report generation, and customer relationship management. Before making changes, it is essential to evaluate the potential impact on these downstream systems and processes.
Why is data lineage the solution? #
Data lineage allows organizations to understand data dependencies and evaluate the impact of proposed changes, minimizing potential disruptions.
Data lineage mandates #
Adopt data lineage solutions that enable organizations to simulate and analyze the effects of changes on data workflows to align with data governance best practices.
Root cause analysis #
Real-life example: An e-commerce company discovers discrepancies in its sales reports. To resolve the issue, they must trace the problem back to its origin.
Why is data lineage the solution? #
Data lineage helps pinpoint the root cause of data discrepancies by visualizing the entire data journey and identifying points where errors may have occurred.
Data lineage mandates #
Utilizing data lineage tools that facilitate quick and accurate root cause analysis adheres to data governance best practices.
You can also learn how automated data lineage can help with fast root cause analysis. Read here.
Collaboration and communication #
Real-life example: Imagine a marketing team and a data analytics team working together on a project analyzing the success of an advertising campaign. For them to work effectively, they need to communicate and collaborate seamlessly.
Why is data lineage the solution? #
Data lineage, especially column-level lineage, helps both teams understand the journey of data, including where it comes from and how it’s transformed. This shared understanding makes it easier for them to exchange insights, resolve issues, and work together efficiently.
Data lineage mandates #
Using data lineage tools that encourage teamwork and clear communication between departments is crucial for successful data governance. These solutions help create a smooth collaboration process, ensuring everyone stays on the same page and works towards the same goals.
Learn more about column-level lineage here
Supporting advanced data initiatives #
Real-life example: A manufacturing company wants to implement advanced analytics and machine learning to optimize its supply chain.
Why is data lineage the solution? #
Data lineage helps organizations ensure the reliability and accuracy of their data, which is crucial for the success of advanced data initiatives like analytics and machine learning.
Data lineage mandates #
In this scenario, data lineage should provide a comprehensive view of the data’s journey, allowing the company to build trustworthy data pipelines for its advanced analytics initiatives.
Find more about data governance framework creation and strategy here
Data lineage for data governance: How to evaluate the right solution #
When evaluating data governance solutions, it is crucial to develop a framework for determining which capabilities and features are necessary and which are merely desirable.
The article 14 Questions for Evaluating Data Lineage provides valuable insights to help you make an informed decision. Below, we summarize the critical aspects to consider in your evaluation framework:
Must-have features #
- Comprehensive data source coverage: The data lineage tool should support various data sources, including databases, data lakes, data warehouses, and APIs, ensuring seamless integration into your organization’s data ecosystem.
- Automated data lineage discovery: The solution should automate the discovery and mapping of data lineage, minimizing manual effort and potential errors.
- Visualizations: The tool should provide intuitive, interactive visualizations that make it easy to explore and understand data lineage and dependencies.
- Impact and root cause analysis: The solution should support impact analysis for planned changes and root cause analysis for data issues.
- Metadata management: The tool should integrate metadata management features to enrich data lineage with essential context, enabling informed decision-making.
- Data governance integration: The data lineage solution should easily integrate with your data governance framework, supporting data cataloging, data quality management, and data policy enforcement.
Good-to-have features #
- Collaboration and communication: The tool should facilitate collaboration and communication among team members by allowing annotations, comments, or sharing data lineage visualizations.
- Customizability: The solution should offer customization options to tailor visualizations, reporting, and notifications to your organization’s specific needs.
- Scalability: The data lineage tool should be scalable to accommodate growing data volumes and the addition of new data sources.
- Security and compliance: The solution should incorporate security and compliance features that align with industry best practices and regulatory requirements.
- APIs and extensibility: The tool should provide APIs or extensions to integrate with other tools and platforms, supporting a cohesive data ecosystem.
Incorporating a checklist of these features into your evaluation framework, you can make a well-informed decision when selecting a data lineage solution that aligns with your data requirements.
Conclusion #
Data lineage is crucial in data governance, as it offers transparency, and traceability, and maintains data quality throughout an organization’s data ecosystem.
By grasping the essential aspects of data lineage and its role in data governance, organizations can effectively manage the intricacies of contemporary data landscapes, adhere to regulatory requirements, and enhance collaboration among teams.
When assessing data lineage solutions, it’s vital to weigh both essential and desirable features and capabilities, ensuring the selected tool corresponds with your organization’s data governance principles and goals.
Implementing a strong data lineage strategy enables organizations to harness the full potential of their data, leading to improved decision-making and the facilitation of advanced data initiatives.
Data lineage in data governance: Related reads #
- Data Lineage Explained: A 10-min Guide to Understanding the Importance of Tracking Your Data’s Journey
- Data Lineage 101: Importance, Use Cases, and Their Role in Governance
- Automated Data Lineage: Key Benefits, Tools Evaluation Guide
- Open Source Data Lineage Tools — 5 Popular to Consider in 2023
- How AI in Data Governance Shows Potential To Help You Scale Data Security, Integrity, Privacy, and Compliance
- Data Governance and Compliance: Act of Checks & Balances
- What is Business Lineage: 10 Use Cases to Apply in 2023
Share this article