What is Operational Metadata & How It Drives Business Success?
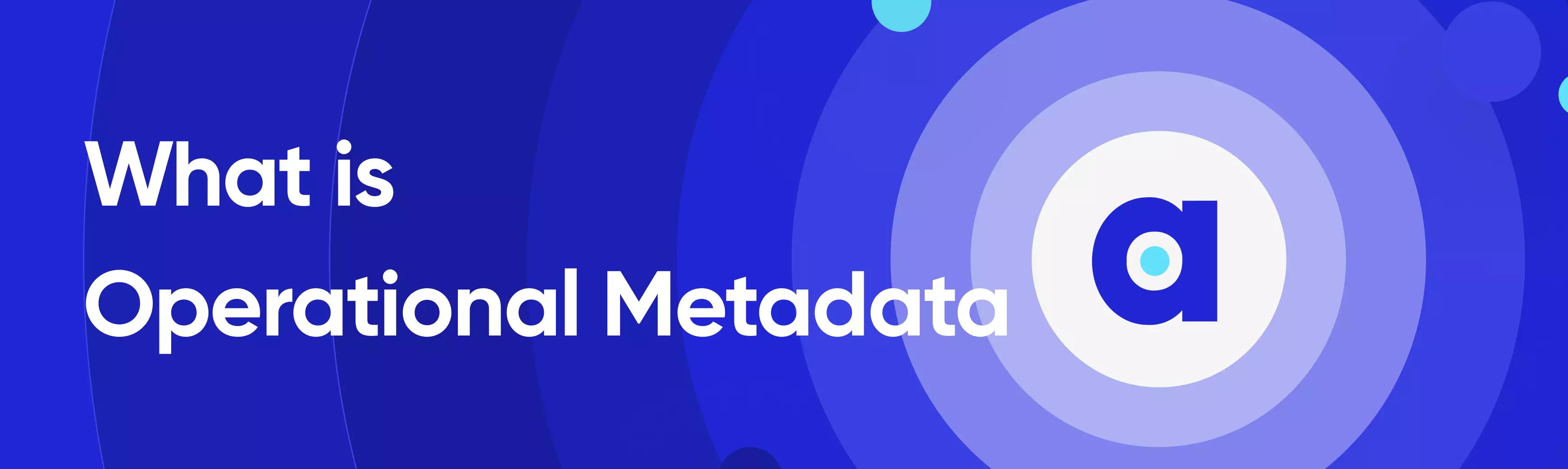
Share this article
Operational metadata refers to information that describes the technical aspects of your data and how it is used within your systems. This type of metadata is essential for managing, monitoring, and optimizing the performance of your data infrastructure, as well as ensuring data quality, consistency, and accessibility across your organization.
In this article, we will explore what is operational metadata in-depth, what are its benefits/purposes, why integrating it with data catalog is crucial and how to use it for business success.
Let’s dive in!
Table of contents
- Understanding the benefits/purposes of operational metadata
- 10 Examples of operational metadata you need to know
- Operational metadata collection 101: How to get started
- Why integrating operational metadata with data catalog and data lineage is crucial for effective data management?
- What is operational metadata in data warehouse?
- How to prepare for leveraging operational metadata for business success?
- Operational metadata: Related reads
Understanding the benefits/purposes of operational metadata
Leveraging operational metadata can drive several crucial outcomes:
1. Improved data discoverability and accessibility
Operational metadata helps users locate and access the required data more efficiently by providing context and details about data sources, formats, and structure. This reduces the time spent searching for data and helps users make informed decisions.
2. Enhanced data quality and consistency
By providing information about data lineage, transformations, and quality checks, operational metadata allows data teams to trace data back to its original source, identify any discrepancies, and ensure data consistency across the organization.
3. Reduced tribal knowledge and reliance on subject matter experts
Operational metadata enables users to understand the data without relying on experts or undocumented knowledge. This reduces bottlenecks and streamlines communication among different teams, improving overall productivity.
4. Better monitoring and optimization of data processes
Operational metadata helps teams monitor the performance of data pipelines and ETL processes, identify bottlenecks, and optimize data workflows. This, in turn, can result in more efficient data processing and lower infrastructure costs.
5. Streamlined compliance and data governance
By providing details on data lineage, usage, and transformations, operational metadata allows organizations to maintain a comprehensive audit trail. This information is vital for meeting regulatory requirements, ensuring data privacy, and implementing effective data governance policies.
6. Enhanced collaboration and communication
Operational metadata can help bridge the gap between technical and non-technical users by providing a common language for discussing data assets. This fosters better collaboration, knowledge sharing, and decision-making across the organization.
7. Single source of truth for data
A well-structured operational metadata management solution ensures that all teams have access to the same, up-to-date information about data assets. That way, it prevents confusion and inconsistency that can arise from multiple sources of truth.
In summary, operational metadata plays a critical role in improving data management, quality, and efficiency within an organization. By investing in a robust metadata management solution, your company will be better equipped to handle the challenges of data management, fostering a data-driven culture and driving better business outcomes.
10 Examples of operational metadata you need to know
We know operational metadata refers to the data that is related to the administration, operation, and management of a system. Now, let us look at some general examples of operational metadata:
1. Execution logs
These logs record details of various processes running within a system, such as start time, end time, execution status (success or failure), and any error messages encountered.
2. Scheduling information
This metadata describes the scheduling of automated tasks, including the frequency, time of execution, and any dependencies between tasks.
3. User access logs
These logs contain information about who accessed a particular resource, when they accessed it, and what actions they performed. It’s critical for security and compliance purposes.
4. Resource utilization
Information about CPU, memory, disk space, and network usage can be considered operational metadata as it relates to the operation and performance of a system.
5. Data lineage
This captures the path that data takes through a system, including where it originates, where it’s been, transformations it’s undergone, and where it ends up. It’s used to track how data flows and changes throughout a system.
6. Versioning information
In software development, operational metadata might include details about the various versions of code, when changes were made, who made them, and what those changes were.
7. Service level agreements (SLAs)
Information related to SLAs, including uptime, response time, and other agreed-upon performance metrics, is another example of operational metadata that helps in managing expectations and commitments between service providers and consumers.
8. Configuration details
Information related to system configuration, such as software settings, hardware configuration, network topology, etc., can be considered operational metadata as well.
9. Backup and recovery information
Details regarding backup schedules, recovery plans, and locations of stored backups fall under operational metadata, aiding in disaster recovery and data integrity efforts.
10. Dependency mapping
This includes details about how different components or services within a system are interconnected and depend on one another. It’s crucial for understanding the impact of changes or failures within a complex system.
These examples highlight the multifaceted nature of operational metadata, revealing how it plays a role in various aspects of managing, operating, and understanding a system. Whether tracking user activity, managing resources, or understanding data flows, operational metadata provides vital insights and control mechanisms.
Operational metadata collection 101: How to get started
To start collecting, leveraging, and automating operational metadata, follow these steps:
1. Assess your current metadata landscape
Begin by evaluating your existing data sources, systems, and processes. Identify the types of operational metadata that are currently being collected, if any, and determine any gaps or inconsistencies in the metadata.
2. Define metadata requirements
Engage stakeholders from various teams (data analysts, data scientists, engineers, business users, etc.) to determine the specific operational metadata that is most relevant and valuable to your organization. This may include information on data lineage, data quality, schema, data transformations, and more.
3. Choose the right tools and technologies
Research and select appropriate metadata management tools and technologies that align with your organization’s requirements and infrastructure. Look for tools that support metadata collection, storage, automation, and integration with your existing data systems.
4. Develop a metadata collection strategy
Design a strategy for collecting operational metadata from your data sources, ETL processes, and data pipelines. This may involve configuring your data systems to automatically generate and store metadata or using specialized tools to capture metadata from your data workflows.
5. Implement metadata automation
Automate the collection, storage, and updating of operational metadata to ensure that it remains current and accurate. This may involve using tools with built-in automation features, or creating custom scripts and workflows to automate metadata processes.
6. Integrate metadata with data catalog and data lineage tools
Connect your operational metadata with data catalog and data lineage tools to provide users with a comprehensive view of your data assets. This will enhance discoverability, traceability, and overall understanding of your data landscape.
7. Establish metadata governance policies
Define governance policies and processes to ensure the quality, consistency, and accuracy of your operational metadata. This may include guidelines on metadata naming conventions, data quality checks, and data lineage documentation.
8. Train and educate users
Provide training and educational resources to your data team and other users to help them understand the importance of operational metadata and how to leverage it effectively. This may include workshops, documentation, or hands-on training sessions.
9. Monitor and refine
Continuously monitor the effectiveness of your operational metadata management processes and make adjustments as needed. This may involve refining your metadata requirements, updating your tools and technologies, or improving your automation processes.
By following these steps, you can successfully collect, leverage, and automate operational metadata within your organization, driving better data management, quality, and efficiency.
Why integrating operational metadata with data catalog and data lineage is crucial for effective data management
Integrating operational metadata with data catalog and data lineage tools provides a holistic view of your data landscape, making it easier for users to understand, discover, and trace data assets. This integration allows users to quickly access relevant operational metadata within the context of your data catalog and data lineage visualization, fostering more effective collaboration and decision-making.
Let us take a hypothetical example:
Let’s say your organization has multiple data sources, including customer data from your loan application system, transaction data from payment gateways, and credit history data from credit bureaus. Each data source undergoes several transformations and merges to create a unified dataset for credit risk analysis.
Integrating operational metadata with your data catalog and data lineage tools would involve the following steps:
1. Collect operational metadata
Capture metadata from each data source, transformation, and data pipeline. This could include information about data formats, schema, data quality checks, and transformation logic.
2. Store metadata in a centralized repository
Organize and store the collected operational metadata in a centralized metadata repository, ensuring that it’s easily accessible and up-to-date.
3. Connect metadata to data catalog
Integrate the metadata repository with your data catalog, so that users can easily access relevant operational metadata while browsing data assets. This helps users understand the data better, ensuring they choose the right datasets for their analysis.
4. Incorporate metadata into data lineage visualization
Include operational metadata in your data lineage tools to provide context and details about each step in the data transformation process. This helps users trace data back to its original source and understand the impact of any changes made to the data.
By integrating operational metadata with data catalog and data lineage tools, you can achieve the following objectives:
1. Improved data discoverability
Users can quickly locate the data they need, as operational metadata provides context about the data sources, formats, and structure.
2. Better understanding of data quality
By including information on data quality checks and validation, users can assess the reliability of the data and make more informed decisions.
3. Enhanced traceability and data lineage
Integrating operational metadata with data lineage tools allows users to trace data back to its origin and understand the transformations applied, enabling them to identify and address data issues more effectively.
4. Reduced reliance on tribal knowledge
With operational metadata readily available in the data catalog and data lineage tools, users can access the information they need without relying on subject matter experts, reducing bottlenecks and streamlining communication.
5. Improved collaboration and decision-making
By providing a comprehensive view of the data landscape, operational metadata integrated with data catalog and data lineage tools helps bridge the gap between technical and non-technical users, fostering better collaboration and data-driven decision-making.
Overall, integrating operational metadata with data catalog and data lineage tools can significantly enhance your organization’s ability to manage, understand, and leverage its data assets, driving better business outcomes.
What is operational metadata in data warehouse?
Operational metadata in a data warehouse is a critical concept, and it refers to the information that is required to manage, administer, and track data warehouse processes. It provides insight into the daily operations and management of the warehouse and is vital for tasks like monitoring, error tracking, performance optimization, and others.
Here’s a list of key points related to operational metadata in a data warehouse:
- Definition and role
- Types of operational metadata
- Relationship with other metadata
- Importance in ETL (Extract, Transform, Load) processes
- Integration with data quality
- Monitoring and auditing capabilities
- Impact on performance optimization
- Challenges and best practices
Let’s look at the above points in brief:
1. Definition and role
Operational metadata is information about the execution of warehouse processes, including data lineage, execution time, error logs, and more. It helps in managing, scheduling, and monitoring the flow of data. Essentially, it’s a set of details about how, when, and where the data is processed.
2. Types of operational metadata
Operational metadata can be classified into different types, such as:
- Execution Metadata: Information about scheduling, performance, and success or failure of operations.
- Audit Metadata: Tracks user access and operation.
- Lineage Metadata: Tracks the path of data from source to destination.
3. Relationship with other metadata
Operational metadata works in conjunction with other types of metadata like technical metadata and business metadata. It contributes to providing a complete view of the data flow and structure within the data warehouse.
4. Importance in ETL processes
In ETL processes, operational metadata plays a crucial role in monitoring and managing the extraction, transformation, and loading of data. It helps in error handling, scheduling, and understanding how data is moved and transformed.
5. Integration with data quality
Operational metadata is vital in maintaining data quality by ensuring that the data is correctly processed and errors are quickly identified and resolved.
6. Monitoring and auditing capabilities
Monitoring and auditing involve tracking user access, changes to data, and execution of various processes. Operational metadata helps in maintaining logs and ensuring that compliance and security standards are met.
7. Impact on performance optimization
By understanding the operational details through metadata, the data warehouse can be optimized for performance. It enables bottleneck identification and helps in tuning the system for better efficiency.
8. Challenges and best practices
Managing operational metadata comes with its challenges like ensuring accuracy, consistency, and adhering to privacy regulations. Best practices involve having proper policies, tools, and methodologies to maintain and utilize this metadata effectively.
Operational metadata in a data warehouse serves as a roadmap for how data is processed within the system. It is essential for various managerial tasks and ensures that data is handled efficiently, securely, and in compliance with relevant regulations and standards.
How to prepare for leveraging operational metadata for business success
Here’s a summarized version of the key elements to have in place before leveraging operational metadata to drive business outcomes:
1. Clear objectives
Define specific outcomes you aim to achieve with operational metadata, such as data discoverability, data quality, or data governance.
2. Metadata requirements
Engage stakeholders to determine relevant and valuable operational metadata types, like data lineage, schema, and data quality checks.
3. Metadata management strategy
Develop a strategy for collecting, storing, and maintaining operational metadata, including plans for automation and integration.
4. Tools and technologies
Select appropriate metadata management tools that support collection, storage, automation, and integration within your data infrastructure.
5. Governance policies
Establish data governance policies to ensure quality, consistency, and accuracy of operational metadata.
6. Centralized metadata repository
Create a centralized repository for storing and managing operational metadata.
7. Integration with data catalog and lineage tools
Connect your metadata repository with data catalog and lineage tools to enhance discoverability, traceability, and understanding.
8. Training and education
Provide resources to help users understand the importance of operational metadata and how to leverage it effectively.
9. Monitoring and refinement
Establish processes for monitoring the effectiveness of your operational metadata management and making adjustments as needed.
Robust metadata management is the key for data-driven teams to discover, understand, trust, and collaborate on data assets across your data universe. If you are looking to implement a metadata management tool for your organization, do take Atlan for a spin.
Operational metadata: Related reads
- Active Metadata: 101 Guide From Pioneers Of The Concept
- What is Business Metadata: 8 Steps for Data Excellence
- What is Technical Metadata: Everything You Need to Know
- What is Metadata? - Examples, Benefits, and Use Cases
- What Is a Metadata Catalog? — Basics & Use Cases
- What is Metadata Management and Why is it So Important?
Share this article