What is metadata management and why is it so important?
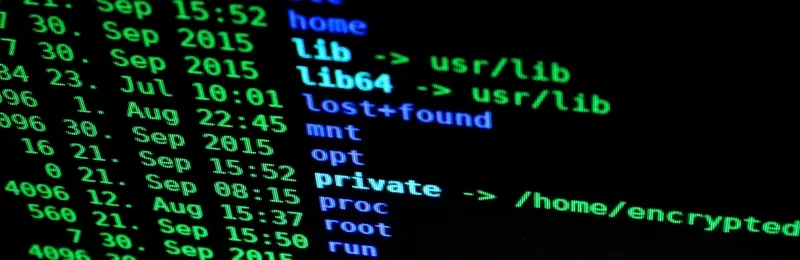
Share this article
Metadata management helps you clean up your messy data and ensure its accuracy, integrity and consistency for better decision-making. This article will explore the evolution of metadata management, its benefits, architecture and implementation within organizations.
What is metadata? #
At the risk of sounding like a broken record, metadata is “data about data”. That’s how the Oxford English Dictionary defines it: a set of data that describes and gives information about other data.
Looking for a non-academic, more hands-on version? Here’s how Gartner defines metadata:
Metadata is information that describes various facets of an information asset to improve its usability throughout its life cycle.
[Ebook] → Data Catalog 3.0: The Modern Data Stack, Active Metadata & DataOps
Why is metadata so important? #
In a rapidly digitizing world, data is everywhere. However, it’s not easy to find, interpret or trust data. For that to happen, organizations must have information that goes beyond asset names or descriptions. They need context such as:
- When was a particular data set created?
- Where was it created?
- Who created it?
- Why did they create it?
Here’s an example. The sales report from APAC region can have multiple columns covering the quarterly sales over the years. Without context, an analyst has no way of knowing the most recent sales figures at a glance.
A lack of context makes it easy to get confused about the data you have, leading to frustrating questions like “what does column XYZ in this data set mean?” or “where can I find the most accurate and up-to-date information for my report?”
Metadata is the invisible glue that connects all your organizational data, making it simple for your data and business teams to discover what they need, when they need it.
Types of metadata #
When you think of metadata, it’s easy to imagine a spreadsheet with headers and data. However, there are different types of metadata that describe the content and context around your information.
Before we proceed, know that there’s no official categorization available. For instance, Ralph Kimball (author of The Data Warehouse Toolkit) lists three types of metadata: technical, business and process metadata.
Whereas NISO (National Information Standards Organization) classifies metadata as descriptive, structural, and administrative.
The three types of metadata based on NISO's classification. Source: O'rielly.
That’s why we’ve compiled all the various descriptions of metadata types that we could find in one place. Let’s take a look.
- Technical metadata: Raw technical details about a data set such as file type, size, creation date, or database schema.
- Business metadata: Detailed information on the contents of a data set, such as category, owner’s details, keywords, or subject matter.
- Process metadata: Operational details from data warehouses and lakes that provide logs (on CPU consumption or transformation steps) and audit reports for traceability and lineage mapping.
- Semantic metadata: Adds meaning to data by referring to the contents of knowledge graphs.
- Structural metadata: Technical information on the physical organization of a data set, which is generally used to set up technical data dictionaries.
- Descriptive metadata: Business-oriented details about a data set’s origins, contents, or availability that add context.
- Administrative metadata: Information on the lineage of a data set, access rights, and permissions to help manage a data set and establish its credibility.
- Rights management metadata: A subset of administrative metadata that refers to intellectual property rights.
- Preservation metadata: A subset of administrative metadata that provides information on archiving and preserving a data set.
We can group these various types in two broad categories, for better understanding of how they exist within a system. Passive metadata and active metadata.
- Passive metadata: Technical metadata that provides basic data definitions such as schema, data types, models, owner name, and so on.
- Active metadata: Descriptive metadata that adds context to your data by providing details on everything that happens to the data. So it’s also operational, business and social metadata, besides technical metadata.
Four types of metadata. Source: Atlan.
With so many types of metadata, it’s challenging to understand and analyze data without proper context.
Even modern data teams with modern infrastructure struggle to find and document their data and that’s why metadata management is so important.
What is metadata management? #
Gartner defines enterprise metadata management (EMM) as the business discipline for managing the metadata about the information assets of the organization.
In other words, it’s a framework of processes, policies, and technologies to catalog information assets within your organization throughout their lifecycles.
Why is metadata management so important? #
For example, every time you add a new data set into your data lake, the information about the same gets added to the asset’s metadata. Or, when you’re querying across databases to consolidate the sales report for a certain quarter, that information gets stored as well.
Such efforts add context to data assets and help keep everyone involved and aware of all the changes made to data. Plus, it makes communication and collaboration across the organization effortless.
Now, metadata management isn’t a new concept. On the contrary, it has been around for ages.
Did you know that the earliest examples of metadata date back to 280 BC to the Great Library of Alexandria?
The library attached tiny tags to each scroll to mention each scroll’s title, subject, and author. As a result, users could find the right scrolls quickly and put the scrolls back at their proper locations after using them
We will not go that far back in time. So instead, let’s begin with the digital examples of metadata management. It’s essential to grasp the evolution of metadata management over time to understand its significance today.
So, let’s sneak a quick peek into its history.
How has metadata management evolved over the years? #
By the 1990s, we’d come a long way from using flat files on servers or local databases with fragmented documentation. Thanks to the internet and advances in computing and data storage, organizations were dealing with vast amounts of data.
To put this vast repository to use, enterprises tasked their IT teams with inventorying data and establishing processes to ensure its availability, integrity, and security.
That brings us to the first-generation metadata management tools, which facilitated passive metadata management, i.e., cataloging, storing, and documenting metadata by relying on manual efforts.
As digital environments matured around us, the amount of data we were dealing with increased exponentially. Staying on top of new data pouring in continuously remained a challenge.
Eventually, as data started becoming more mainstream in the 2000s, managing it went beyond IT, leading to the emergence of data governance.
Here’s how our co-founder Prukalpa Sankar puts it:
As the idea of data governance started catching on, many companies went all-in on data. They created entire departments for data governance, built new roles, invested in data governance committees, and more. These teams started realizing they needed software to manage all this metadata. That kickstarted a golden era for metadata management.
Companies leveraged this and blended inventorying data with adding relevant business context. This ushered in the era of second-generation tools for metadata management.
However, they involved complicated technologies with steep learning curves and prolonged implementation cycles.
Additionally, these tools came with a monolith architecture and only supported on-premise deployment. Each data system required a separate software installation — companies couldn’t simply roll out updates by pushing an update on the cloud. The entire setup could take over 18 months and the onus of maintaining such a complex infrastructure fell onto the shoulders of individual organizations.
Another thing — the second-generation tools weren’t flexible and couldn’t be adapted to match the requirements of an organization.
As a result, the largest tech companies with enormous resources at their disposal started building metadata management solutions in-house. Think of it as trying to build your own car — getting the brakes from one shop, the engine from a mechanic and then putting it all together in your garage. Such a makeshift solution might serve its purpose, but it might not integrate all the aspects of metadata management.
Also, cobbling together various parts to build something that works might be feasible for companies like (Airbnb, Lyft, and Netflix) with vast engineering resources at their disposal. Unfortunately, those who can’t afford the same become laggards and struggle with metadata management.
Now, in the aftermath of a deadly pandemic, we’ve entered the age of accelerated digital transformation and the importance of data-driven decision-making has skyrocketed. Distributed work and BYOD policies have become the norm.
In such an era, organizations need modern solutions that foster collaboration, data sharing, and simplify access to the right data and manage metadata actively — enable continuous access and processing of metadata.
Say hello to metadata management 3.0 or active metadata management!
Now let’s look at the benefits of active metadata management. If you’re still racking your brains comparing metadata versus active metadata, then this section should make things clear.
The Ultimate Guide to Evaluating a Data Catalog
Download free ebook
Benefits of active metadata management #
1. Data discovery #
Effective metadata management ensures that all data is classified and stored in an easy-to-use manner. However, it still requires a certain amount of manual effort to keep things updated.
With active metadata management, you can add context to data continuously and automatically by auto-classifying assets and generating automated business glossaries. This makes it easy to find, understand and use data.
Self-service search and browse. Source: Atlan
Since data teams spend 2 out of 5 days just to find the right data asset, solving data discovery problems will significantly boost productivity — faster insights, quicker time-to-market, better cost savings, and lesser risks.
According to Dataversity:
Automated enterprise metadata management provides greater accuracy and up to 70% acceleration in project delivery, harvesting metadata from various data sources, mapping any data element from source to target, and harmonizing data integration across platforms.
2. Data quality #
Automated data profiling takes care of inconsistencies, outliers, and anomalies in data sets. With active metadata management, you can employ algorithms for frequency distribution, variable type detection, outlier detection, and more, making it easy to spot and eliminate bad data in real-time.
Automated data quality profiles. Source: Atlan
Such tools also let users schedule automated quality audits to monitor data and ensure its accuracy and integrity.
Moreover, since these tools come equipped with data quality dashboards, you get a bird’ eye view of data quality across your organization’s ecosystem.
3. Data lineage and governance #
Metadata management documents everything from the origins of a data set to each transformation that it undergoes, with detailed logs and records.
Active metadata management brings automation to the mix by ensuring auto-construction of data lineage and auto-classification of assets like PII data.
Auto-constructed data lineage. Source: Atlan
Additionally, tools facilitating active metadata management are built for granular access controls, which bolsters data security. As a result, metadata can tell you the origins of data, its location at all times, and its usage, making both lineage tracking and compliance with data privacy regulations a breeze.
4. Embedded collaboration #
Embedded Collaboration is about work happening where you are with the least amount of friction. With active metadata management, embedded collaboration can become a reality.
What embedded collaboration could look like. Source: Atlan
As we’ve mentioned earlier, active metadata management:
- Improves data discovery
- Solves access-related issues
- Ensures data quality, integrity and compliance
So, any data consumer within an organization can access the data they need instantly, using the systems and tools that they prefer.
Moreover, active metadata management tools are built for the modern workspace — employees distributed across locations and timezones. They’re equipped with in-line chats, annotations and also make data easy-to-share (with a single link).
In-line chats and annotations. Source: Atlan
Now that the importance of metadata management is evident, let’s explore its architecture.
Metadata management architecture #
The architecture for metadata management could be centralized, decentralized, or a mix of both.
A centralized architecture builds a single repository — a single source of truth — that integrates data from all sources. This makes it easier to implement standard policies, standards, definitions and rules for data management and governance.
A centralized approach to metadata management. Source: TDAN.
On the other hand, a decentralized architecture involves setting up a central (federal) repository for all metadata, facilitating the sharing and reusing of metadata with several local metadata repositories.
A decentralized approach to metadata management. Source: TDAN.
While both approaches come with pros and cons, we recommend establishing “a unified repository that stores all kinds of metadata, in raw and processed forms”.
What would that look like? We imagine it as diverse components of the metadata architecture grouped under an overarching layer of metadata management.
Here’s how the Eckerson Group envisions it:
Metadata management is a cross-sectional task that spans an entire Business Intelligence and Analytics architecture. The goal is usually to establish centralized metadata management in order to provide a holistic perspective of an entire organization.
Metadata management architecture and its components. Source: Eckerson Group
Components of the metadata management architecture #
While metadata management acts as an overarching layer, the architecture itself has three core components — a metadata storage repository, process workflows, and access control.
1. A centralized repository #
Organizations use data from various sources and in different formats to inform their processes. It’s not uncommon to have data lakes storing vast amounts of raw, unstructured data and then building several data stores to categorize the data for further use.
That’s why it’s essential to set up a centralized repository that acts as a single source of truth for all data and is accessible to all users with the right access credentials.
Wondering how this would look in active metadata management? The repository would be built using open APIs and interfaces to simplify discovery and access. Additionally, it would be powered by knowledge graphs to break data silos, bring disparate data assets to life, and keep them connected.
The repository would be powered by intelligent business glossaries and Google-like search to add context and simplify data discovery. As a result, business users can access the data they want without relying on your engineers or using complex SQL queries.
2. Process workflows #
Data undergoes several transformations and informs various business operations across an enterprise. For example, adhering to compliance guidelines requires companies to organize, classify, tag, and define their data assets according to a set of regulatory standards.
Another example is that of an analyst pulling data assets from multiple sources to compile a comprehensive sales report for an entire quarter.
In most modern organizations, power users of data set up numerous staging areas, apply 3-4 types of ETLs, and develop hand-written SQL scripts on the fly. The engine dictating these process workflows is responsible for keeping track of all such changes to the metadata, establishing lineage, monitoring data quality and integrity, and enforcing governance.
While a second-generation metadata management platform might require a degree of human intervention at this stage, an active metadata management tool would rely on AI-powered algorithms and automation to eliminate any manual efforts.
3. Access control and governance #
A major challenge that’s keeping organizations from achieving complete data democratization is finding the right balance between discoverability and security. A solid metadata management architecture might just be the key to solving this problem.
For instance, granular access policies defined at the metadata level can restrict access based on preset permissions and ensure continuous access to relevant business data. That’s why it’s crucial to weave such access controls into the framework governing the metadata management architecture.
Just like the previous component, access control policies might require human intervention in conventional metadata management tools.
However, an active metadata management solution would auto-classify, group, and tag data and then restrict access based on pre-defined policies. It can propagate access policies in real-time via the lineage map so that every table or column derived from a column tagged as sensitive will inherit the same classification and security controls.
What is a metadata management tool? #
While we’ve covered bits and pieces that define the attributes of a metadata management tool in the previous sections, we’re going to summarize it all here.
Any metadata management tool you choose must:
- Simplify and automate inventorying of data assets from diverse sources and enrich them with adequate business context
- Enable easy discovery of the data assets you need with the right search tools
- Set up a centralized repository for all organizational data and keep it updated
- Facilitate the design of workflows explaining how metadata gets created, transformed, and consumed across the organization
- Enforce data security, privacy, and compliance policies to preserve the integrity and credibility of your data
Thinking about metadata versus active metadata again, to pick the right tool for a modern data stack? We’ve got you covered.
The architecture of an active metadata management tool. Image by Atlan
An active metadata management tool would — in addition to meeting the requirements mentioned above — also:
- Be built using an open API architecture
- Facilitate auto-crawling and auto-classification of metadata
- Automate data quality checks
- Employ RPA to emulate human decision-making and oversee the entire metadata ecosystem
- Support integrations and plugins that bolster embedded collaboration
One last thing — a modern metadata management platform should take reverse metadata into account to guarantee that a data consumer always gets the context, no matter which tool they are in. This could be anything from information on the owner of a data asset to its data quality metrics.
Reverse metadata is about metadata not being available in a “standalone data catalog”. Instead, it’s about making relevant metadata available to the end-user, wherever and whenever they need it, to help them to do their job better.
Data is a powerful tool, but it’s useless without effective metadata management. So, if you’ve been stuck in the vicious cycle of buying expensive tools with archaic licensing fees and then, 2-3 years later, realizing that it isn’t working, then it might be time for a change.
An active metadata management platform like Atlan can help you stop wasting time finding and understanding data and instead, spend more time making strategic decisions that drive your business.
Related reads on metadata management #
- Types of metadata: How each helps with faster data discovery and better insights
- Metadata management 101: Benefits, tools, and best practices
- Active metadata management: What is it and how to get started
- Top 6 best practices for effective enterprise metadata management
- Enterprise metadata management and its importance in the modern data stack
Share this article