The Active Governance Manifesto
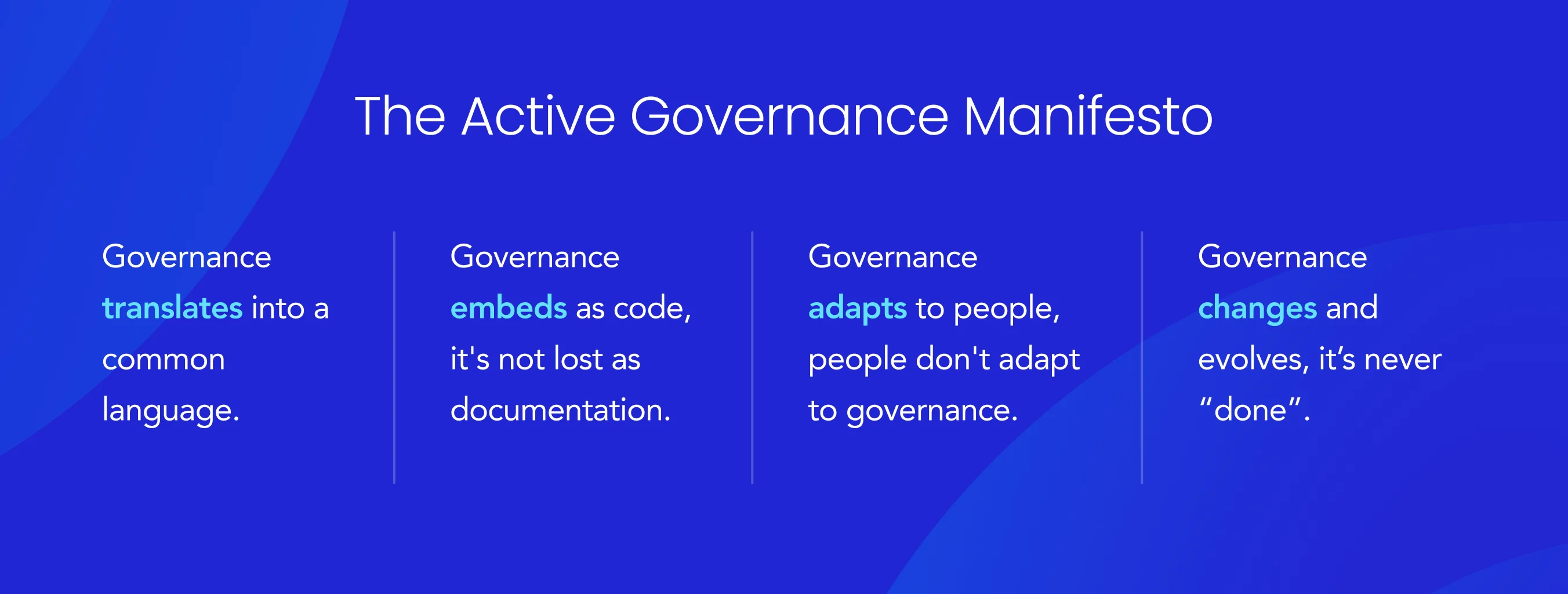
Share this article
Active Governance is a new paradigm of data and AI governance, built for ever-increasing change and diversity. #
The data landscape is evolving at an unprecedented pace. Tools, teams, and outcomes are multiplying rapidly. In this era of accelerating diversity and change, traditional governance approaches are outmoded and ineffective at channeling change into innovation. Active Governance is a new paradigm of data and AI governance, built for ever-increasing change and diversity.
The four principles of Active Governance #
- Governance translates diversity into a common language: Active Governance translates the diversity of data and AI into a common, understandable language accessible to all. It is the connective tissue unifying an organization’s tools, people, and data objectives.
- Governance adapts to the people, people don’t adapt to governance: Active Governance does not force people to conform, but adapts itself to fit teams’ existing processes, cultures, and needs. It is flexible and configurable, capable of operating in centralized or decentralized structures.
- Governance embeds as code, it’s not lost as documentation: Active Governance embeds guardrails and policies directly into code and workflows, not static documentation doomed to obscurity. Its rules live within the tools that humans of data use daily.
- Governance changes and evolves, it’s never done: Active Governance evolves alongside innovations in data and AI. It provides a future-proof foundation, extensible to new technologies, roles, and use cases as they emerge.
Understanding the principles of Active Governance #
- Governance translates diversity into a common language.
Data governance relies on information flowing from person to person and team to team. The key problem is that different groups of people talk about data differently, either in the form of different outcomes they want to enable or the different tools and data assets they work with day-in and day-out.
For example, data producers may come into a conversation talking about data in terms of data pipelines or Python code, while data consumers are talking about SQL code and their data visualization needs. Meanwhile, data stewards are talking about policies and privacy regulations, such as HIPAA or GDPR. In short, each group has their own language for exchanging information about data.
Data needs a common language that can unify the organization. This common language needs to be understood by both technical and non-technical users and act as the connective tissue across diverse tools, personas, and outcomes. For many organizations today, data products act as the common language — acting as a curated, reusable, reproducible, and trusted interface between diverse data personas, tools, and outcomes.
- Governance adapts to the people, people don’t adapt to governance.
Governance is not one-size-fits-all. Every organization is unique, with unique teams, operating processes, cultures, personas, and outcomes they want to drive with data at different times in their journey.
Ownership, stewardship, and responsibility are more than likely completely separate (or non-existent) things when you’re just getting started. Some domains might have a data steward, but that person isn’t responsible for the quality of the data. Some data teams might own the management of a particular application’s data, but they are not stewarding how the data is used. Passive governance fails in today’s data landscape because it takes a one-size-fits-all approach, expecting organizations and their people to conform to rigid processes and technology.
Instead, governance needs to be flexible and adaptive. Whether it’s centralized, decentralized, or federated, governance processes should be flexible enough to adapt to different structures and governance technology should be configurable enough to adapt in the same direction.
- Governance embeds as code, it’s not lost as documentation.
Governance is often lost in forgotten PDFs, Excel spreadsheets, and Confluence files. This means that, no matter how well-intentioned a company and its employees are, data governance policies and rules are often not actually put into practice. When these guardrails aren’t built into routines, they’re often missed and forgotten.
Governance needs to be embedded into the way data teams work — across diverse personas, tools, and outcomes. This involves modern technology that automates these policies within daily data work, through embedded code rather than stale, static documentation gathering digital dust.
Governance can be embedded in 4 ways:
- Shift-right: Data consumers at the end of the data pipeline use Business Intelligence tools for data analysis and reporting. Governance needs to be embedded into these tools, so data consumers don’t have to go searching for context, asking if they can trust the data or if the data is sensitive and private.
- Shift-left: Data producers at the beginning of the data pipeline are tasked with delivering data that is trusted, high-quality, and contextual, but manual documentation interrupts their workflows. Governance should be embedded into data producer workflows, like in dbt YAML files as data contracts or through CI/CD, to ensure data producers can deliver on their promise of trusted data with ease.
- Shift-down: The data stack is increasingly becoming fragmented and disconnected. Active governance connects these fragmented tools through metadata, like tags, that are standardized and synchronized across the data stack.
- Shift-up: Stewardship is often disconnected from data, leading to policies becoming stale and ineffective at actually governing the data estate. Shifting governance up means connecting the data layer with the policy layer, ensuring that policies are embedded into the diverse workflows across the data estate.
- Governance changes and evolves, it’s never done.
In data, change is the constant. We live in a world that is constantly innovating, with technology shifts happening more frequently. With every new innovation, from Teradata making big data possible, to Redshift introducing cloud computing, to Snowflake giving rise to the Modern Data Stack, to generative AI, there are new tools, teams, and outcomes that governance needs to enable.
For example, with Generative AI, every Chief Data Officer is now approaching the realm of unstructured data, vector databases, LLMs, and AI applications to empower teams like Customer Success, Marketing, and Sales to leverage AI through unprecedented use cases like RAG and fine-tuning. None of these were a priority for CDOs two years ago.
Governance, in its approach to process and technology, fundamentally needs to be open and extensible to change. It needs to build the foundation for today and for the future.
About Atlan
Atlan is the active metadata platform for data and AI governance, enabling data and business teams to easily find, trust, and govern AI-ready data. Backed by top investors including GIC, Insight Partners, Sequoia, and Salesforce Ventures, Atlan features deep integrations across the modern data stack and is trusted by the likes of Ingersoll Rand, Cisco, Autodesk, Unilever, Ralph Lauren, FOX, News Corp, Nasdaq, NextGen, Plaid, and HubSpot to enable major AI and data democratization initiatives. To learn more about Atlan, please visit our website and follow us on X (@AtlanHQ) and LinkedIn.
The Active Governance Manifesto: Related reads #
- How Autodesk Democratizes Data with an Adaptive, Federated Approach
- How Nasdaq Drives a Common Language for Data
- How Elastic Embeds Governance into Daily Workflows
- Active Data Governance: What It Is and How to Get Started
Share this article