How AI Data Governance Shows Potential To Help You Scale Data Security, Integrity, Privacy, and Compliance
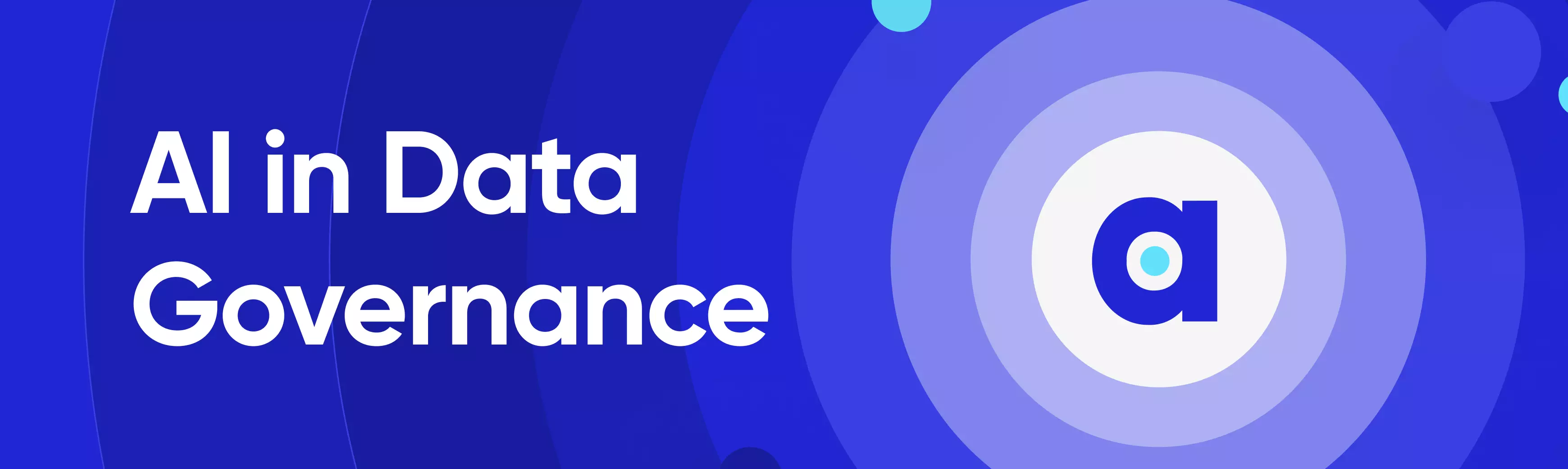
Share this article
Using AI in data governance can potentially help you scale your efforts to understand, manage, and use data while keeping it secure.
As your technological debt grows and the amount of data generated explodes, data governance becomes hard to manage without AI and automation.
Let’s explore the possibilities of AI in data governance by first grasping the concept of shifting left, followed by how artificial intelligence can catalyze this shift.
Table of contents #
- Shifting data governance to the left
- How can AI help in shifting data governance to the left?
- 5 possible AI data governance workflows
- Summing up on AI in data governance
- AI data governance: Related reads
Shifting data governance to the left #
What is the “shift left” approach? #
The “shift left” approach in DevOps is about beginning testing as early as practical in the software development lifecycle (SDLC). This helps you catch potential design defects as early as possible and fix them right away.
Similarly, the “shift left” approach in data governance is about moving the responsibility for data quality and compliance while data assets are being created, modified, or updated.
Joshua Shinavier, the senior staff engineer at LinkedIn, highlights the shift left approach in data governance by comparing it to software development:
“Software engineers have known for decades that documentation on code should live right next to the code itself, leading to solutions like Javadoc, Pydoc, etc. being embedded in the program code itself. It is not hard to apply similar ideas to the schema and data definition languages associated with SQL, Avro, Protobuf, Thrift, etc.”
Why shift data governance to the left #
Traditionally, data practitioners shipped projects as they were, then
went back later to add data governance requirements dictated by top-down mandates.
With the “shift left” approach, data governance won’t be an afterthought to data and analytics architecture and use cases.
Instead, data governance can become an integral part of the daily workflows of data practitioners. That means embedding data protection, security, and privacy into each process — moving data governance closer to data asset creation.
Schematic representation of what it means to shift data governance to the left. Image by Atlan.
So, how can you effectively realize the concept of shifting data governance to the left? An exciting way to solve that problem is with AI in data governance.
How can AI help in shifting data governance to the left? #
Shifting data governance to the left means:
- Creating data asset descriptions, READMEs, and more while curating data
- Tagging and setting policies for data assets as soon as you source them
- Generating announcements or alerts whenever you’re about to make changes to an asset
These merely scratch the surface. Undertaking these tasks manually isn’t efficient when you are an enterprise with hundreds and thousands of data sources, tools, and systems.
That’s where artificial intelligence can help.
For instance, once you connect data sources to a data catalog, you can automatically process the underlying metadata. After that, you can ask AI to spot and suggest fixes for incomplete or inaccurate assets — no ownership, missing classification tags, etc.
Another example is documenting multiple data assets in bulk. Once you’ve created the definition, description, and README for one asset, you can ask AI to automatically document the same for other similar data assets.
AI can also help you identify recurring tasks in data governance and recommend setting up rule-based automation to eliminate the manual effort involved.
So, what would the workflows for these processes look like? And how would they affect data quality, discovery, access, and compliance?
Let’s look at five data governance workflows powered by AI.
5 possible AI data governance workflows #
Leveraging AI in data governance may make it possible for you to scale the following workflows:
- Data asset quality monitoring
- Anomaly detection using automated data lineage
- Data asset discovery with complete context
- Granular, role-based access control
- Regulatory compliance with automation
Before we go further, it’s crucial to note that the first step is to compile all the metadata for your data estate in one place. Diversity and granularity of metadata are central to making the use of AI in data governance a reality.
Now, let’s explore each workflow.
1. Data asset quality monitoring #
You can only monitor data asset quality when you have a bird’s-eye view of all the metadata updates in one place.
Once you set up your dashboards, you can monitor each asset in real-time as an AI data governance platform would track everything — classifications, requests, schema changes — and update the dashboard continuously.
A modern data catalog can help you automate quality workflows and notify the right people whenever there’s a discrepancy. However, you still have to set up these data workflows manually for the catalog to spot any issues.
With AI in data governance, you can go a step further — AI can study the issues that occur, and then suggest which data pipelines have discrepancies even without a workflow in place.
2. Anomaly detection using automated data lineage #
A key capability of an active data governance platform is automated column-level lineage. Automated lineage helps you understand:
- Where a data asset came from
- How it transformed throughout the data pipeline
- Where it was ultimately consumed
AI can read your lineage and quality metadata to track anomalies in your daily workflows and communicate which downstream BI assets will be affected.
Column-level data lineage in an AI data governance platform. Image by Atlan.
Another challenge in such situations is knowing the right person to notify — is it the table owner? Is it the owner of the database system?
AI can suggest the right owner responsible for analyzing and handling the anomaly.
AI can also alert you when someone having the right access credentials makes critical changes to data assets — changing the glossary definition, asset classification, trust certificate, and more. That’s because these changes require multiple approvals from various stakeholders in your data teams.
3. Data asset discovery with complete context #
As mentioned earlier, AI in data governance will only work when you’ve connected all the data sources, apps, and systems to a central platform — a modern data catalog — so that it can compile and curate metadata automatically.
Such a connected ecosystem makes it easier for technical and business users to quickly find, explore, and use the data they need from various tools like Snowflake, Looker, or dbt.
The modern data catalog helps you by compiling all the metadata about each data asset and setting up 360-degree data asset profiles. Think of these as LinkedIn profiles, but for data assets.
With AI in data governance, you can scale asset profile creation by asking AI to automatically document the context, and suggest owners, tags, certifications, etc.
Data asset profiles for complete context. Image by Atlan.
4. Granular, role-based access control #
The best way to set up access control is to build policies around:
- Who your data users are
- Which teams do they work in
- What projects they’re working on
This requires you to personalize your approach to access control as per each user persona, business domain, and project in your organization.
With AI in data governance, you can analyze metadata to auto-populate tag suggestions for similar data assets and then propagate them via lineage.
AI would also use custom metadata to help you select which data assets should be visible to each user role in your organization while restricting visibility to those that wouldn’t find this information relevant.
For example, a finance team member won’t need access to dbt transformations and Airflow DAGs, but they may need to dig into lineage to troubleshoot an odd Salesforce report or query a Snowflake table to determine ARR.
5. Regulatory compliance with automation #
Compliance is easier when your technological debt is small. As your technology stack matures and architecture becomes more complicated, you’ll have new data sources appearing at all times.
Regulations like GDPR require you to fulfill data deletion requests from your customers and while you can automate some of it, you still have to scour through your secondary systems to find and delete data.
In such situations, rule-based automation can help. You can set up workflows to bulk-create tags, attach owners, propagate column descriptions, attach classifications, and more.
What does rule-based automation look like? Image by Atlan.
With AI in data governance, you can rely on AI to identify sensitive assets and tag them as PII even without rule-based automation in place. It will also propagate the masking and encryption policies for this asset across all downstream applications via lineage.
What shifting data governance to the left means for your organization #
Summing up on AI in data governance #
The possibilities of AI in data governance are endless. Think of it as the iPhone moment for the modern data stack.
For instance, since ChatGPT’s launch in November 2022, it has set records for the fastest-growing user base with 100 million monthly active users in January 2023. Today it’s being used to draw up legal contracts, offer financial advice, write haikus, translate content, and more.
The potential use cases keep growing as our understanding of the application as well as the chatbot itself continues to evolve.
The same applies to AI in data governance. What we’re seeing so far is just the tip of the iceberg and we cannot wait to see what else is in store for data teams and their efforts in scaling data governance.
Share this article