Data Fabric vs. Data Virtualization: Overview, Comparison, and Differences
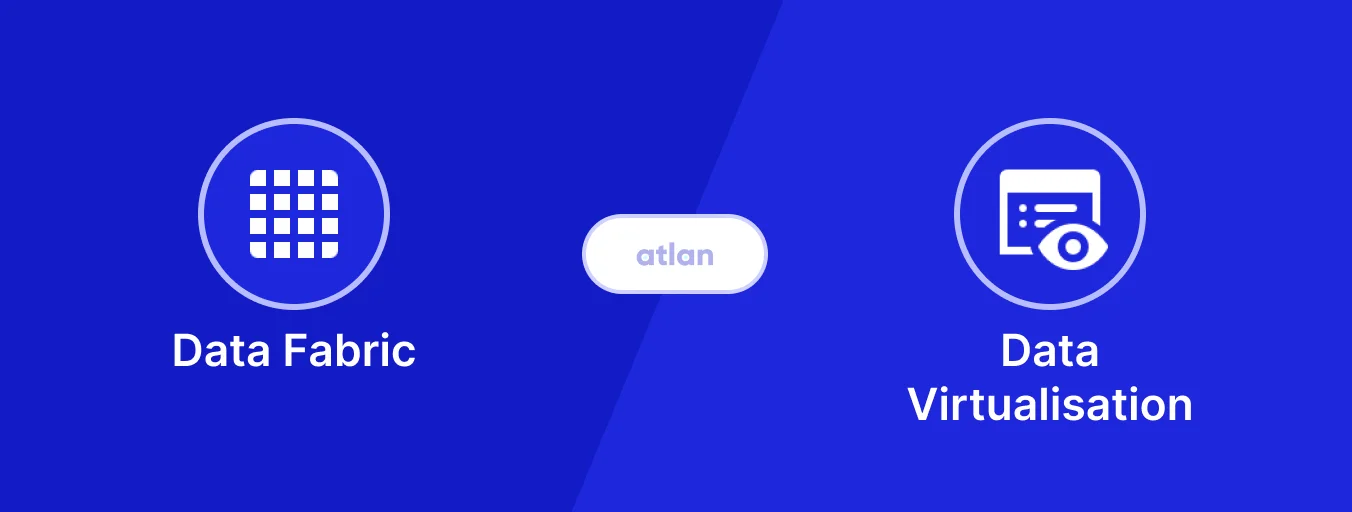
Share this article
Here’s a quick explainer if you’ve been comparing data fabric vs. data virtualization. Data fabric is a data architecture framework that simplifies data management to real-time access to all data using a virtual access layer. This layer refers to data virtualization, which is a data integration concept and the key to enabling modern data architectures like data fabric.
With several architecture frameworks and data management concepts springing up every day, telling them apart can be confusing. That leads to search strings, such as “data fabric vs. data virtualization”, “data fabric vs. data mesh” and “data virtualization vs. data federation”.
In this article, we’ll be exploring the concept of virtualization and how it fits into the data fabric architecture framework. This should help clarify the differences between the two concepts and their advantages.
[Download ebook] → Data catalogs are going through a paradigm shift. Here’s all you need to know about a 3rd Generation Data Catalog
Why should organizations modernize their data architectures? #
For instance, traditional companies hold vast amounts of data spread across several repositories and lines of business. As most of their systems are legacy IT, simply pulling together the information required for analytics could take several weeks, with intervention from engineers being a prerequisite.
Introducing new systems that run on modern technologies means significantly rethinking the entire ecosystem — a project that could take months to finish. By the time such projects go live, technology would have advanced leaps and bounds.
The key problem here is access, and that’s where virtualization can help. An architecture like data fabric can simplify data management by providing a single, unified platform compatible with all the technologies and systems across the organization.
Such a platform also eliminates silos and facilitates self-service and rapid decision-making using data.
Now let’s explore the concept of data virtualization — the key to modernizing your data architecture.
What is data virtualization? #
According to Gartner, virtualization is the abstraction of IT resources that masks their physical nature and boundaries from resource users.
Extending the definition to data, data virtualization is a concept of data integration that creates a virtual abstraction layer by eliminating data silos and connecting all data assets. It provides a universal layer across different applications within your data ecosystem.
Here’s how DAMA (Data Management Association International) defines data virtualization:
Data virtualization enables distributed databases and multiple heterogeneous data stores to be accessed and viewed as a single database. So, rather than physically performing ETL on data with transformation engines, data virtualization servers perform data extract, transform and integrate virtually.
The goal is to build a single view of all data, regardless of the source or format, without physically copying or moving that data.
A modern data architecture framework using data virtualization to simplify access. Image source: Eckerson Group
If you’re looking for an analogy, then Dataversity says it best:
Think of data virtualization as a TV guide, which includes the content of many different channels in one place.
So, you know exactly what data lives where - simplifying data discovery and access.
A Guide to Building a Business Case for a Data Catalog
Download ebook
Use cases: Data virtualization #
As we’ve mentioned earlier, most organizations have data silos spread across the cloud and disparate storage systems such as data lakes, warehouses, and data stores.
So, some of the top use cases of data virtualization are around virtual data warehouses and lakes:
- Virtual data warehouse: Virtual warehouses are faster and simpler to set up, as no physical movement of data is involved.
- Virtual data lake: Like warehouses, virtual data lakes are easier to manage, as they facilitate faster data access, seamless integration, greater accuracy, and no-code/low-code analytics for business users.
Data virtualization has two more important use cases, which simplify data discovery, facilitate governance initiatives and enable data democratization. These include:
- Data catalog: A modern data catalog with active metadata management capabilities solves the access problem. So, data gets updated in real-time, along with its context. Moreover, users can share data assets with just a link, even in multi-cloud environments.
- Self-service analytics: Empowering business users to run analytics reduces the pressure on technologists (scientists and engineers), speeding up the process of extracting value from data. This also speeds up the deployment of analytics-powered applications.
Now let’s look at data fabric — a modern data architecture framework and explore how data virtualization fits into this model.
What is data fabric? #
Here’s how Eckerson defines data fabric:
Data fabric combines architecture, technology, and services designed to ease the complexities of managing many different kinds of data, using multiple database management systems, and deployed across various platforms.
As mentioned earlier, data fabric involves setting up a single, unified layer for data management on top of distributed data. This makes accessing and sharing data assets frictionless.
Gartner provides a great analogy to envision the concept of data fabric — think of it as a self-driving car.
If the driver is active and paying full attention to the route, the car’s autonomous element has minimum or no intervention. However, if the driver is slightly lazy and loses focus, the vehicle immediately switches to a semi-autonomous mode and makes the necessary course corrections.
Similarly, data fabric observes data pipelines in the beginning. Eventually, it automates the repetitive tasks and offers recommendations to improve the outcome. This saves the time data teams spend on operations, leaving them more time for innovation and strategy.
Want to learn more about data fabrics? Read more here.
How does virtualization complement a data fabric architecture? #
According to Gartner, data fabric must have a robust data integration backbone.
Here’s why. Data fabric has to:
- Support many data sources and formats
- Catalog and manage all types of metadata
- Support active metadata management
- Be compatible with several data pipeline workflows
- Automate data orchestration
- Empower all kinds of data consumers (technologists and business users)
Enabling such capabilities requires powerful technologies for performing analytics and a solid data integration layer to access all data assets — that’s where virtualization plays a critical role.
Use cases: Data fabric #
Since data fabric centralizes access to all data across a distributed ecosystem, it makes the entire data ecosystem interoperable and reduces the turnaround time involved in finding, analyzing, and understanding data.
So, the primary use cases of data fabric are around implementing and using technology at speed. The applications include machine learning, predictive and prescriptive analytics, data discovery, and democratization.
Let’s look at some use cases:
- Data discovery: With virtualization supporting the data integration layer in the data fabric, the right people have access to the data they need, whenever they need it.
- Machine learning: A data fabric environment speeds up the process of data integration, making usable data available for further analytics. ML models are effective when they get speedy access to the right data at the right time.
- Data democratization: Central access and faster data preparation speeds make it possible to automate several aspects of data analytics. As a result, even business users can glean the insights they want, track metrics, and prepare reports without needing any help from specialists like data scientists.
How do companies implement a data fabric design? #
Data fabric is a technology-centric framework.
That’s why the design must be supported by next-gen technologies such as knowledge graphs, ML-powered modern data catalogs, data virtualization, and active metadata management.
Key pillars of a comprehensive data fabric. Image by Gartner.
The first step is to design a framework that makes sense for your organization. The next step is implementation, which involves building a platform that:
- Consolidates all metadata, with context, in a single repository
- Supports active metadata management
- Enables granular access control and governance
- Promotes open collaboration and sharing of data
That’s where a modern data catalog and governance platform like Atlan can help. We’ve also put together a comprehensive guide to help you evaluate modern data catalogs available in the market. Take a look here.
Data fabric vs. data virtualization: Summary of comparison #
As mentioned earlier, it’s easy to get confused with data fabric vs. data virtualization, especially with so many buzzwords popping up in the data management and governance ecosystem.
That’s why we’ve put together a table to explain the differences between data fabric and data virtualization:
Data fabric | Data virtualization |
---|---|
Data fabric is an end-to-end architecture for modern data management. | Data virtualization creates a data abstraction layer to integrate all data without physically moving it. |
Data fabric is used to simplify data discovery, governance and active metadata management. | Data virtualization is used when there is a need to integrate data quickly. |
Data fabric should be used when an organization requires a centralized platform to access, manage and govern all data. | Data virtualization should be viewed as one of the core elements of a data fabric architecture. |
A data fabric architecture powered by data virtualization can help organizations deliver insights at the speed of business. The centralized platform makes finding and interpreting data a breeze for technical and business users alike.
So, rather than making comparisons such as data fabric vs. data virtualization, organizations should look at building a model that exploits the benefits of both concepts.
Related Resources: #
- Data catalogs for data fabrics: 5 essential features to look for during the evaluation.
- Data fabric vs data mesh: Architecture, benefits, use cases & more.
- What is data fabric: Definition, components, and benefits.
- Data warehouse: Definition, concept, architecture, and components
- Data Catalog: Does Your Business Really Need One?
Want to understand more about the merits of data fabric and data virtualization?
Share this article