Data Governance Tools: Importance, Key Capabilities, Trends, and Deployment Options
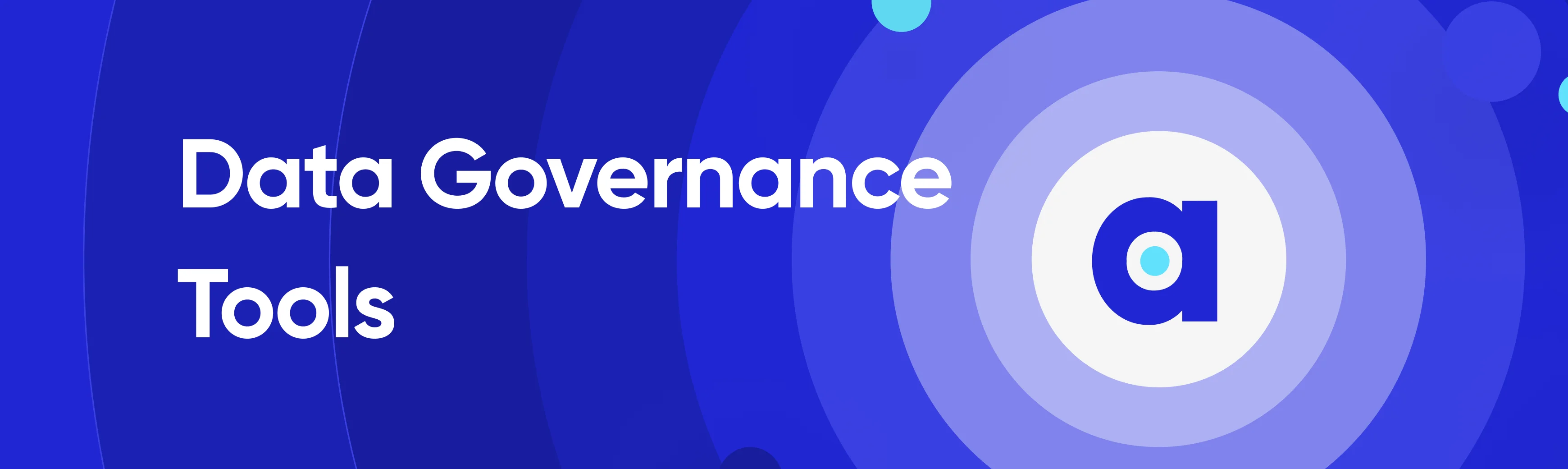
Share this article
Data governance tools are pivotal in driving strategic business decisions and shaping corporate destinies. These tools provide an efficient means to manage, control, and use the increasing volumes of data that businesses generate.
In this article, we’ll cover the importance of data governance tools, their essential components, top industry choices, and how to select and deploy them.
Let’s start by understanding the importance of data governance tools for your data estate.
Table of contents #
- Understanding data governance tools
- 5 Key capabilities of data governance tools
- Trends in data governance tools
- Top data governance tools
- Choosing the right data governance solution
- Deployment options for data governance tools
- Summary of data governance solutions
- Data governance tools: Related Reads
Understanding data governance tools in 2024 #
Data governance tools are software solutions used to streamline and automate the multifaceted process of managing, organizing, and protecting enterprise data.
Critical components of these tools include data stewardship, quality control, cataloging, lineage tracking, security, compliance, and data visualization.
Each of these elements contributes significantly to maintaining the integrity and enhancing the value of data.
In the absence of these tools, the process of data governance can be chaotic and error-prone. However, with a robust data governance tool, these burdensome tasks can be automated.
Here’s a table comparing your data ecosystem with and without data governance tools in terms of data management, quality, cataloging, compliance, and security.
Scenario | With Data Governance Tools | Without Data Governance Tools |
---|---|---|
Data Management | Data is efficiently managed with a single source of truth, enhancing consistency and reliability. | Data management can become chaotic, with multiple sources of truth and inconsistencies across the system. |
Data Quality | Automated quality checks ensure high-quality data, leading to more accurate insights and decisions. | Risk of poor data quality due to lack of automated quality checks, leading to potential inaccuracies in decision-making. |
Data Cataloging | Automated cataloging ensures accurate, consistent metadata, making data easily discoverable and usable. | Manual cataloging can lead to errors and inconsistencies, making data harder to locate and use effectively. |
Compliance | Automated compliance mechanisms ensure adherence to data regulations, reducing the risk of penalties. | Manual handling of compliance is time-consuming and risk-prone, possibly leading to non-compliance penalties. |
Data Security | Strong security features ensure data protection, minimizing the risk of breaches. | Without robust security protocols, there’s a higher risk of data breaches and unauthorized access. |
5 key capabilities of data governance tools #
Data governance tools can have a diverse range of functionalities that cater to the unique needs of managing data in a business environment. However, the five most important capabilities include:
- Data quality management
- Master data management (MDM)
- Data privacy and compliance
- Data cataloging and metadata management
- Data security
Let’s delve into the nuances of each capability further.
Data quality management #
Data quality management is the bedrock upon which reliable decisions and strategies are built. Effective data governance tools provide features that enforce data quality standards, ensuring that data is not just correct, but also consistent and useful.
Read more → How Contentsquare ensured data governance and quality with Atlan
Master data management (MDM) #
Master data management (MDM) is about creating a ‘single source of truth’ for the company’s data. MDM functionality within data governance tools helps eliminate data silos, reduces redundancy, and ensures that all data-related decisions are made using the most accurate and updated data.
Read more → How Nasdaq built a Google for its data to drive a common understanding
Data privacy and compliance #
With data regulations like GDPR and CCPA, data privacy and compliance have become more critical than ever.
Modern data governance tools equipped with robust privacy and compliance features can automate the process of adherence to these regulations, reducing the risk of non-compliance.
Read more → How a UK-based digital bank improved GDPR compliance with an active metadata platform
Data cataloging and metadata management #
Data cataloging and metadata management are vital to enhancing data accessibility and contextualization.
With a comprehensive data catalog, data practitioners can locate and understand the information they need without requiring extensive technical knowledge.
Read more → How Elastic used a data catalog to improve data governance and build trust in data
Data security #
Data security is a critical component of data governance since data breaches can result in massive financial losses, non-compliance fines, and reputation damage.
Data governance tools provide a variety of security features to ensure that access to data is appropriately managed and that data is protected from both internal and external threats.
Read more → How Austin Capital Bank controls access in an easily repeatable fashion
Next, let’s look at the top trends shaping the data governance tooling space.
2024 Trends in data governance tools #
In 2024, the world of data governance tools has been profoundly influenced by several key trends, such as the cloud, the growing complexity of data, AI, and ML.
First, we see an increase in the adoption of cloud-based data governance tools, thanks to their scalability, cost-effectiveness, and ease of integration.
Second, the growing complexity of hybrid data ecosystems has led to a surge in demand for tools capable of managing both structured and unstructured data across different platforms.
One of the most transformative trends in data governance tools, however, has been the incorporation of Artificial Intelligence (AI) and Machine Learning (ML) technologies. AI and ML have brought automation and predictive analytics to data governance, fundamentally reshaping how organizations manage their data.
As the data landscape continues to evolve in complexity, the reliance on data governance tools will only increase. These tools are transforming from being a “nice-to-have” to an absolute necessity in the data strategy of any forward-thinking enterprise.
Top data governance tools #
In the realm of data governance tools, both proprietary and open-source options cater to the varied needs of businesses.
Among the proprietary solutions, Atlan emerges as a distinguished player with its comprehensive, proactive, automation capabilities that foster a collaborative data culture within organizations.
Atlan was declared a leader in the G2 Spring 2023 Grid® Reports for Data Governance, Machine Learning Data Catalog, and Data Quality categories.
Also, read → The G2 Grid® Report for Data Governance
Open-source tools also play a critical role in the market, offering customizable solutions. These include Amundsen, DataHub, Apache Atlas, Magda, and OpenMetadata.
Each provides its unique capabilities and benefits in data governance.
The choice between open-source and proprietary tools depends on a myriad of factors including your budget, in-house expertise, and specific needs.
For a more detailed exploration of these tools, including in-depth comparisons, see the recent Forrester Wave report.
Choosing the right data governance solution #
Selecting the right data governance tool is a critical decision that can significantly impact a company’s data management efficacy. Key factors to consider include:
- Scalability: Can the tool accommodate your company’s growth and handle increasing data volumes efficiently?
- Ease of use: Is the tool user-friendly, enabling non-technical staff to engage effectively?
- Integration capabilities: How well does the tool integrate with your existing data infrastructure and software ecosystem?
- Cost-effectiveness: Does the cost of the tool align with your budget, and does its value justify the investment?
Additionally, it’s essential to acknowledge that the optimal choice of a data governance tool often depends on the size of the company, the sector it operates in, and its unique needs.
For instance, a small tech startup might prioritize integration capabilities and cost-effectiveness. Conversely, a large multinational bank might focus on scalability and robust compliance features.
The implementation phase also warrants careful consideration. The data governance tool should align with the company’s data culture, ensuring a smooth transition that promotes rather than hampers daily operations.
Deployment options for data governance tools #
The way a data governance tool is deployed can significantly impact its utility and effectiveness. Here’s a brief exploration of the various deployment options available:
- On-premise deployment
- Cloud-based deployment
- Hybrid deployment
Let’s explore each deployment option for data governance tools further.
1. On-premise deployment #
This traditional deployment method relies on internal servers and in-house IT infrastructure. It offers a high level of control and may be suitable for companies with stringent data security and regulatory requirements.
However, on-premise deployment often entails manual software updates and may require significant IT resources and capital investment.
2. Cloud-based deployment #
Cloud-based data governance solutions offer scalability, cost-effectiveness, and ease of updates. They rely on internet connectivity and off-site data centers, eliminating the need for significant upfront hardware investments.
For example, Snowflake’s cloud data governance solution provides these advantages.
However, cloud deployments require stable internet connectivity as well as even stricter attention to security than on-premises deployments.
Single-tenant vs. multi-tenant architecture in cloud deployments
In a cloud deployment, companies can opt for single-tenant (dedicated) or multi-tenant (shared) architectures.
Single-tenant solutions provide higher levels of data isolation and customizability but may cost more. On the other hand, multi-tenant solutions are typically more cost-effective and scalable but may have limitations in customization and data isolation.
Transitioning between these deployment models may be necessary as a company grows or when its needs change. A smooth transition requires careful planning and execution to minimize disruption and data loss.
3. Hybrid deployment #
A hybrid approach combines elements of both on-premise and cloud-based deployments, offering flexibility and catering to a wider range of organizational needs.
Companies might choose to store sensitive data on-premise while leveraging the cloud for scalability and cost-effectiveness for less sensitive, voluminous data.
Summary of data governance solutions #
In the era of data-driven decision-making, the critical role of data governance tools cannot be overstated. The year 2024 has seen the rise of influential trends in data governance tools, including the adoption of cloud-based solutions, hybrid data ecosystems, and the integration of AI and ML technologies.
Data governance tools will continue to grow as the cornerstone of effective, efficient, and secure data management, driving strategic business decisions and shaping the future of businesses.
Data governance tools: Related Reads #
- What is Data Governance? Its Importance, Principles & How to Get Started?
- Key Objectives of Data Governance : How Should You Think About Them?
- Data Governance Framework — Examples, Templates, Standards, Best Practices & How to Create One?
- Data Governance and Compliance: Act of Checks & Balances
- How to implement data governance? Steps, Prerequisites, Essential Factors & Business Case
- How to Improve Data Governance? Steps, Tips & Template
- 7 Steps to Simplify Data Governance for Your Entire Organization
- Snowflake Data Governance — Features, Frameworks & Best Practices
- Automated Data Governance : How Does It Help You Manage Access, Security & More at Scale?
- Enterprise Data Governance — Basics, Strategy, Key Challenges, Benefits & Best Practices
Share this article