How to Achieve an Optimal Hybrid Data Governance Model?
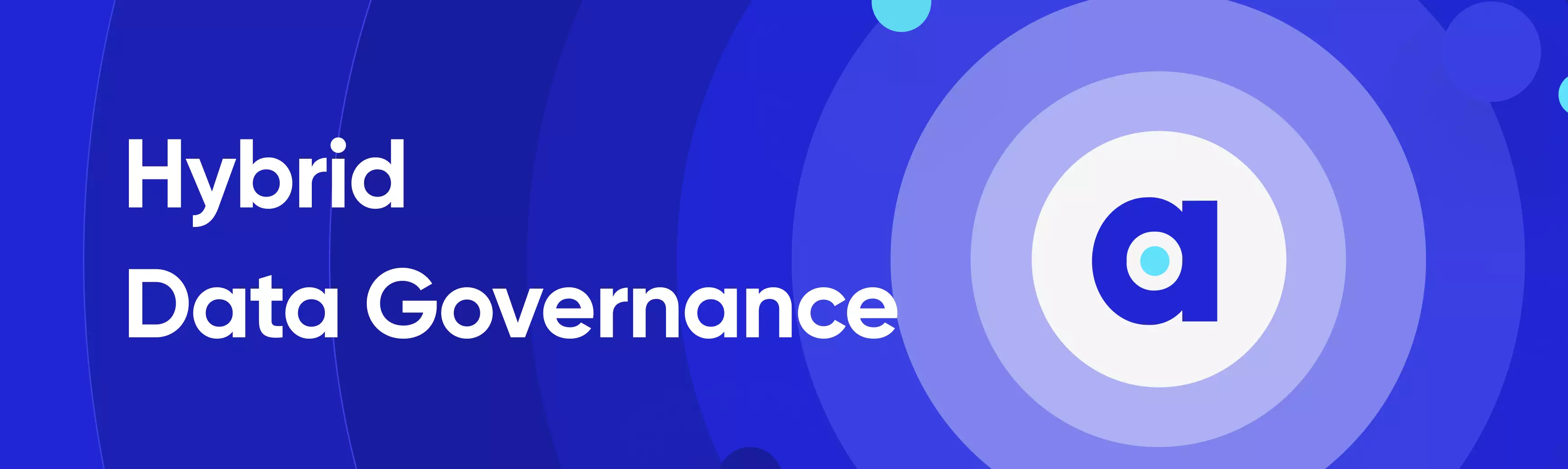
Share this article
What is hybrid data governance? #
Hybrid data governance is an approach that combines the best practices of both top-down and bottom-up data governance models. This model is designed to facilitate a balance between centralized and decentralized data governance efforts, ensuring that both the organization’s strategic objectives and the needs of individual business units are met.
In a hybrid data governance model, you will have:
1. Centralized governance #
A core data governance team or council is established at the organizational level. This team is responsible for setting the overall data governance strategy, policies, and standards, as well as monitoring compliance and ensuring data quality.
2. Decentralized governance #
Business units, departments, or teams within the organization take on the responsibility of implementing and enforcing the data governance policies and standards set by the centralized team. They also have the autonomy to create their own supplementary rules and processes to address specific data governance needs within their domain.
The hybrid model can be beneficial for a growing organization like yours, as it can provide the flexibility needed to adapt to the evolving data landscape and business requirements.
Table of contents #
- What is hybrid data governance?
- Advantages of hybrid data governance model
- The best of both worlds: How hybrid data governance can help your organization
- Creating a balanced approach: How to establish a hybrid data governance model
- Factors to consider when choosing a data governance tool for a hybrid model
- Choosing the best data governance framework model for hybrid data governance
- Rounding it all up
- Hybrid data governance: Related reads
Advantages of hybrid data governance model #
Some advantages of hybrid data governance include:
1. Agility #
The decentralized approach allows individual teams to quickly respond to their specific data governance needs, while still adhering to the overall organizational strategy.
2. Scalability #
As your company grows, the hybrid model can easily scale to accommodate more data sources, business units, and use cases.
3. Collaboration #
The centralized and decentralized components work together, fostering communication and collaboration across the organization.
4. Ownership #
Both the central governance team and individual business units have clear roles and responsibilities, ensuring that data governance is effectively managed at all levels.
5. Balance #
The hybrid model balances the need for organizational consistency with the flexibility required for individual teams to effectively manage their data.
As your company grows and looks to lead the EV charging industry, adopting a hybrid data governance model could be a strategic move. This approach can help you effectively manage your data, establish industry-wide data standards, and ultimately monetize your data assets.
The best of both worlds: How hybrid data governance can help your organization #
Now, let’s dive into the two types of data governance models: centralized and decentralized.
Centralized data governance #
In a centralized data governance model, a single, core team or council is responsible for managing and overseeing all aspects of data governance across the organization. This includes setting data policies, standards, and procedures, as well as monitoring compliance and ensuring data quality.
The centralized team usually consists of representatives from different business units and functions, such as IT, legal, and finance.
Advantages of centralized data governance:
- Consistency Provides uniform data policies and standards across the organization.
- Control Ensures a high level of control over data management, reducing the risk of data breaches and non-compliance.
- Efficiency Reduces the duplication of efforts by centralizing resources and expertise.
Disadvantages of centralized data governance:
- Rigidity May be slow to respond to the unique needs of individual business units or changing business requirements.
- Limited ownership Business units may feel less ownership and accountability for data governance, as the responsibility lies primarily with the central team.
Now, let’s look into decentralized data governance
Decentralized data governance #
In a decentralized data governance model, individual business units, departments, or teams within the organization are responsible for managing their own data governance initiatives. Each unit creates and enforces its own data governance policies, standards, and procedures, which are tailored to address their specific needs and requirements.
Advantages of decentralized data governance:
- Flexibility Provides more freedom for business units to adapt data governance practices to their unique needs and requirements.
- Responsiveness Can quickly respond to changes in business requirements or data management needs.
- Localized ownership Encourages a greater sense of ownership and accountability for data governance at the business unit level.
Disadvantages of decentralized data governance:
- Inconsistency May lead to differing data policies and standards across the organization, making it difficult to maintain a consistent data environment.
- Risk of silos Can result in isolated data management efforts, reducing collaboration and communication between business units.
Hybrid data governance combines the strengths of both centralized and decentralized models to create a more balanced and effective approach to data governance.
By blending the consistency and control of a centralized model with the flexibility and ownership of a decentralized model, a hybrid approach can help organizations better manage their data governance initiatives and adapt to evolving business needs.
Creating a balanced approach: How to establish a hybrid data governance model #
Setting up a hybrid data governance model for your organization involves several steps. Here’s a high-level overview of the process:
1. Assess your organization’s needs #
Begin by evaluating your organization’s data governance requirements, including data quality, compliance, security, and privacy needs. This will help you determine the right balance between centralized and decentralized governance.
2. Establish a central data governance team or council #
Create a cross-functional team with representatives from different departments, such as IT, legal, finance, and business units. This team will be responsible for setting overall data governance strategy, policies, and standards, as well as monitoring compliance and ensuring data quality.
3. Define roles and responsibilities #
Clearly outline the roles and responsibilities of both the central data governance team and individual business units. This includes specifying who is responsible for creating, maintaining, and enforcing data governance policies, standards, and procedures at various levels within the organization.
4. Develop a data governance framework #
Create a comprehensive data governance framework that includes policies, standards, and procedures to guide data management activities across the organization. This framework should be flexible enough to allow individual business units to adapt it to their specific needs.
5. Implement a data governance toolset #
Invest in tools and technologies that support data governance activities, such as data cataloging, data lineage tracking, data quality management, and data privacy and security management. These tools should be accessible to both the central data governance team and individual business units.
6. Communicate and train #
Communicate the data governance framework, policies, and standards to all relevant stakeholders within the organization. Provide training and resources to help employees understand their roles and responsibilities within the hybrid data governance model.
7. Foster collaboration #
Encourage collaboration and communication between the central data governance team and individual business units. This may include regular meetings, joint projects, or shared platforms for knowledge exchange.
8. Monitor and measure #
Track the effectiveness of your hybrid data governance model by establishing key performance indicators (KPIs) and monitoring them over time. Adjust the model as needed to ensure that it continues to meet your organization’s evolving data governance needs.
9. Continuously improve #
Treat data governance as an ongoing process, with regular reviews and updates to the framework, policies, and standards. Continuously seek feedback from stakeholders and make improvements based on lessons learned and changing business requirements.
By following these steps, you can successfully implement a hybrid data governance model that balances the strengths of centralized and decentralized approaches, allowing your organization to efficiently manage its data assets and adapt to the growing and dynamic EV charging industry.
Factors to consider when choosing a data governance tool for a hybrid model #
When evaluating data governance tools to support your hybrid data governance model, consider the following factors to ensure you choose the right solution for your organization:
1. Compatibility #
The tool should be compatible with your organization’s existing data infrastructure and technology stack, including databases, data warehouses, and data integration platforms.
2. Scalability #
As your organization grows, the tool should be able to scale to accommodate increasing data volumes, complexity, and governance requirements.
3. Functionality #
Look for a tool that provides a comprehensive set of data governance features, such as data cataloging, data lineage tracking, data quality management, data privacy and security management, and reporting and analytics capabilities.
4. Flexibility #
The tool should be adaptable to both centralized and decentralized governance scenarios, allowing you to implement the hybrid model effectively. It should also support customization to meet the unique needs of your organization and individual business units.
5. Ease of use #
Choose a tool that is user-friendly and intuitive, allowing both technical and non-technical users to navigate and interact with the system easily.
6. Collaboration and communication features #
The tool should support collaboration between the central data governance team and individual business units, facilitating knowledge sharing, communication, and joint decision-making.
7. Integration capabilities #
The tool should be able to integrate seamlessly with other systems and tools in your organization, such as data integration platforms, BI tools, and data management solutions.
8. Vendor support #
Consider the quality of customer support provided by the vendor, including their responsiveness, expertise, and availability of resources, such as documentation and training materials.
9. Total cost of ownership #
Assess the total cost of ownership for the tool, including licensing fees, implementation costs, maintenance fees, and any additional costs for upgrades or add-on features.
10. Security and compliance #
Ensure that the tool meets your organization’s security and compliance requirements, including data privacy regulations like GDPR or CCPA.
As you evaluate potential data governance tools, it’s essential to involve stakeholders from various departments and business units in the decision-making process. This will help ensure that the selected tool meets the needs of both the central data governance team and the individual business units, allowing you to effectively implement a hybrid data governance model.
Choosing the best data governance framework model for hybrid data governance #
There isn’t a one-size-fits-all “best” data governance framework for hybrid data governance, as the specific framework that will work best for your organization depends on your unique needs, goals, and context.
However, you can leverage widely recognized data governance frameworks and principles as a starting point, then tailor them to support your hybrid approach. Some popular data governance frameworks include DAMA-DMBOK (Data Management Body of Knowledge), COBIT (Control Objectives for Information and Related Technologies), and CMMI-DCMM (Data Management Maturity Model).
Rounding it all up #
We’ve discussed hybrid data governance, which combines the best practices of centralized and decentralized data governance models to achieve a balance between organizational consistency and flexibility for individual business units. This approach is suitable for growing organizations like yours, as it can efficiently manage data assets and adapt to the evolving needs of the EV charging industry.
To implement hybrid data governance:
- Assess your organization’s data governance needs.
- Establish a central data governance team.
- Define roles and responsibilities for the central team and business units.
- Develop a flexible data governance framework tailored to your hybrid approach.
- Implement a data governance toolset to support both centralized and decentralized efforts.
- Provide communication, training, and resources to stakeholders.
- Foster collaboration and communication between the central team and business units.
- Monitor and measure the effectiveness of the hybrid model using KPIs.
- Continuously improve the data governance framework, policies, and standards.
By following these steps, you can create a data governance strategy that enables your organization to efficiently manage its data assets, establish industry-wide data standards, and potentially monetize its data in the growing EV charging market.
If you are evaluating and looking to deploy best-in-class data access governance for your data ecosystem without compromising on data democratization? Do give Atlan a spin.
Hybrid data governance: Related reads #
- What Is Data Governance? Its Importance, Principles & How to Get Started?
- Automated Data Lineage: Benefits + Tools Evaluation Guide
- What Is a Data Catalog? & Why Do You Need One in 2023?
Share this article