Data Quality in Data Governance: The Crucial Link That Ensures Data Accuracy and Integrity
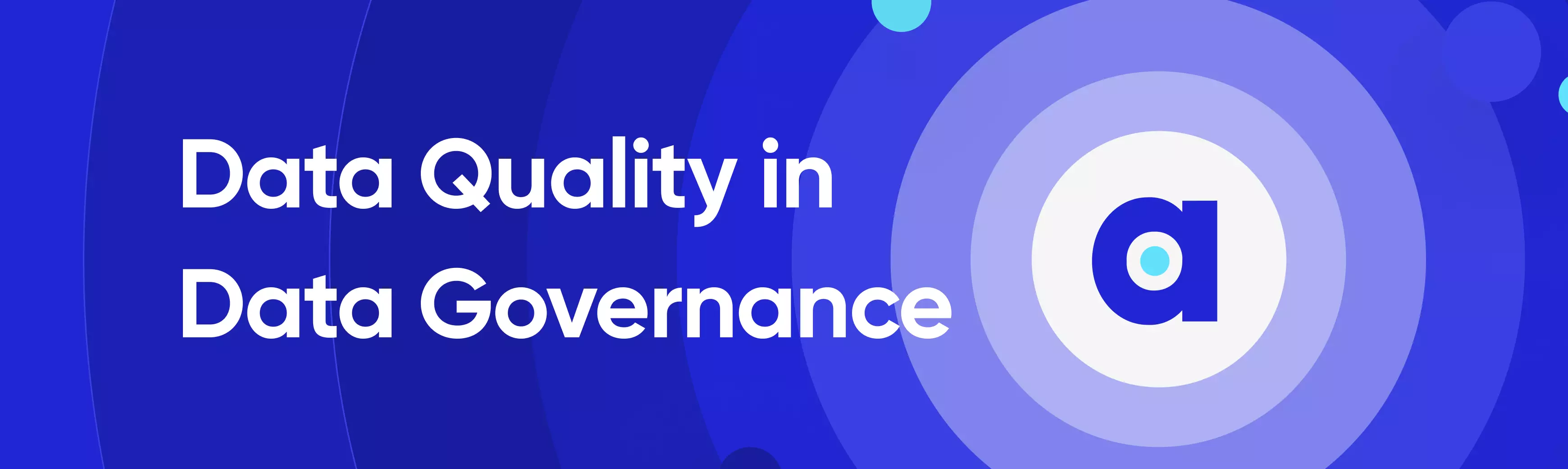
Share this article
Data quality in data governance means the degree to which data in a system is accurate, consistent, reliable, and relevant for a particular use. It is one of the key aspects of effective data governance. It’s about ensuring that the data your organization collects, stores, and uses is of high integrity and can be trusted for decision-making. Various aspects of data quality include accuracy, validity, reliability, timeliness, relevance, and completeness.
Data governance, on the other hand, is the overall management of the availability, usability, integrity, and security of the data employed in an enterprise. It’s a set of principles and practices that ensure high quality throughout the complete lifecycle of your data.
In this article, we will learn how data quality and data governance are interconnected, its importance, and the different data quality tools needed for effective data governance.
Let dive in!
Table of contents #
- Is data quality a part of data governance? Discovering their connection
- The interconnection between data quality and data governance: A tabular view
- Deciphering the interrelationship between data governance and data quality: A detailed view
- The importance of ensuring data quality in data governance
- 7 Crucial data quality rules in data governance
- Implementing data quality tools for effective data governance: A step-by-step guide
- Bringing it all together
- Data quality in data governance: Related reads
Is quality a part of data governance? Discovering their connection #
Imagine data as a key ingredient in a recipe. Just as the quality of ingredients determines the taste of the dish, the quality of data influences the effectiveness of decisions made by a business.
Data quality in data governance, simply put, refers to the ‘goodness’ of the data being used. Good data is accurate, complete, consistent, relevant, and timely. When your data meets these standards, you can trust the insights derived from it and make reliable decisions.
In other words, data governance is the strategy and data quality is a key tactical goal of that strategy. They’re deeply interconnected in several ways:
- Establishing standards
- Responsibility
- Processes and procedures
- Monitoring and improvement
- Data literacy
- Technology and tools
Let us look into each of the above interconnected ways in brief:
1. Establishing standards #
A good data governance program sets the standards for data quality within the organization. It defines what ‘good’ data quality looks like, which metrics to use, and how to measure them.
2. Responsibility #
Data governance assigns roles and responsibilities for maintaining data quality. This includes data owners, stewards, and custodians, who are responsible for various aspects of data quality in their respective domains.
3. Processes and procedures #
Data governance lays down the processes and procedures for maintaining data quality. This could include data cleansing, de-duplication, integration, and validation routines.
4. Monitoring and improvement #
Data governance also involves monitoring data quality levels, auditing for compliance with standards, and driving continuous improvement initiatives.
5. Data literacy #
Data governance ensures that the organization understands the importance of data quality and is capable of maintaining it. This includes training and education, communication, and advocacy efforts.
6. Technology and tools #
Data governance involves selecting and implementing the right technology and tools to support data quality. This includes database management systems, data integration tools, data quality tools, and business intelligence tools.
In summary, without a strong data governance program, maintaining high data quality becomes a challenge. And without high-quality data, the insights and decisions derived from that data may be flawed, potentially leading to suboptimal or even harmful outcomes. Therefore, your initiative to develop a data governance strategy is a crucial step toward ensuring data quality in your organization.
The interconnection between data quality and data governance: A tabular view #
In this section of the blog, we’ll explore the relationship between data governance and data quality in a simple table:
Data Governance | Data Quality |
---|---|
Sets the overall strategy for managing data within the organization | A key tactical goal within that strategy |
Establishes standards for data quality | The degree to which data meets those standards |
Assigns roles and responsibilities for managing data | Managed by the roles defined in the governance plan |
Defines processes and procedures for data management | Maintained through those processes and procedures |
Involves monitoring, auditing, and improvement of data management practices | Quality levels are monitored and improved as part of these practices |
Ensures data literacy within the organization | The importance of high-quality data is understood as part of this literacy |
Selects and implements technology and tools to support data management | The quality of data is supported and improved by these tools and technologies |
Remember that while the table provides a simplified view, data governance and data quality are complex, interrelated concepts that require careful planning, execution, and ongoing management.
As you develop your data governance strategy, it’s important to keep data quality in mind as a key goal and to ensure that your governance practices effectively support and enhance the quality of your data.
Deciphering the interrelationship between data governance and data quality: A detailed view #
Now, let look into each of these interrelationships in detail:
1. Setting the overall strategy for managing data vs. A key tactical goal #
Data governance is like the constitution of a country - it sets the broad framework and principles of how data should be managed within an organization. For example, a part of your governance strategy might be to centralize data management for better control and consistency.
Data quality, then, becomes a key goal within that strategy, a tactical objective that needs to be achieved - for instance, ensuring that patient records are accurate and up-to-date in your centralized database.
2. Establishing standards for data quality vs. Degree to which data meets those standards #
Data governance defines what ‘good’ data quality looks like. For instance, it might define a standard that no more than 0.5% of patient records should contain errors. Data quality is then the degree to which your data meets those standards - for example, if only 0.2% of records contain errors, your data quality is high and surpasses the standard.
3. Assigning roles and responsibilities for managing data vs. Managed by the roles defined in the governance plan #
Data governance involves defining who is responsible for what data. For instance, a governance plan might assign a ‘data steward’ role to certain employees who are responsible for maintaining the accuracy of patient records.
Data quality is then managed by those data stewards - for instance, they might regularly review and correct any errors in the patient records they are responsible for.
4. Defining processes and procedures for data management vs. Maintained through those processes and procedures #
Data governance sets out the processes for managing data - for example, how new patient records should be entered into the system, how changes should be tracked, and how errors should be corrected.
Data quality is maintained through these processes - for instance, by following the defined process, employees can ensure that new records are accurate, changes are correctly recorded, and errors are promptly fixed.
4. Monitoring, auditing, and improvement of data management practices vs. Quality levels are monitored and improved #
Data governance involves regularly checking that data management practices are being followed and looking for ways to improve them - for example, you might conduct audits to ensure that patient records are being correctly maintained and look for any common issues that need to be addressed.
Data quality is what you monitor and aim to improve through these activities - for instance, if an audit finds that a significant number of records contain errors, you would look to improve your data quality by addressing the underlying issues.
5. Ensuring data literacy vs. Understanding the importance of high-quality data #
Data governance includes promoting data literacy throughout the organization - for example, by providing training on how to handle data correctly and why it’s important.
Data quality is a key part of what employees need to understand. For instance, employees in healthcare organizations need to know why accurate patient records are critical for providing good healthcare and how their actions impact data quality.
6. Selecting and implementing technology and tools vs. Quality of data supported and improved by these tools #
Data governance involves choosing the right systems and tools for managing data - for example, a database management system that provides robust data validation features.
Data quality is then supported and enhanced by these tools - for example, the validation features of the database system could automatically check that new patient records meet certain criteria, helping to prevent errors and improve data quality.
The importance of ensuring data quality in data governance: Two inseparable aspects for modern organizations #
The relationship between data quality and data governance is symbiotic. While governance policies and procedures set the framework for maintaining and improving data quality, the quality of data itself directly influences the effectiveness of these governance initiatives. Here’s why:
- Accelerating informed decision-making
- Ensuring regulatory compliance
- Boosting operational efficiency
- Enhancing customer satisfaction
- Facilitating risk management
Let us look into each of the above aspects in brief:
1. Accelerating informed decision-making #
High-quality data is a prerequisite for generating accurate and valuable insights, which drive informed decision-making. Through effective data governance, organizations can maintain the consistency and accuracy of their data, ensuring that the data-driven insights and subsequent decisions are trustworthy.
2. Ensuring regulatory compliance #
Maintaining high data quality is essential to comply with various industry-specific regulations and laws. Regulatory bodies often require organizations to demonstrate the accuracy and reliability of their data. Effective data governance allows organizations to establish and follow protocols that help in maintaining and documenting data quality, thus avoiding legal and compliance issues.
3. Boosting operational efficiency #
Quality data reduces ambiguities and errors in business processes, leading to enhanced operational efficiency and productivity. Data governance provides the standards and procedures that are essential for preserving and improving data quality, and therefore, streamlining operations.
4. Enhancing customer satisfaction #
Quality data allows for better understanding and segmentation of customers, which in turn leads to more effective marketing strategies and superior customer service. An integral part of data governance is protecting the integrity and confidentiality of customer data, which helps improve customer trust and satisfaction.
5. Facilitating risk management #
Accurate, reliable, and timely data is essential for effective risk management. An organization can anticipate and respond to potential threats more efficiently if its risk assessment is based on high-quality data. Through sound data governance, organizations can ensure the availability of quality data for risk management purposes.
Quality data is not just about accuracy; it’s about bringing trustworthiness and reliability to the table when driving business decisions. A robust data governance framework, in turn, ensures this quality is maintained and leveraged effectively to realize organizational goals.
As the volume and complexity of data continue to increase, a focus on maintaining data quality within data governance initiatives is not just recommended; it’s a business imperative.
7 Crucial data quality rules in data governance #
Data quality in the realm of data governance refers to implementing set standards or rules that help ensure data is not only accurate and reliable, but also fit for its intended use.
While these rules may vary depending on an organization’s specific needs and industry requirements, there are several common key principles that form the backbone of maintaining high-quality data, which are:
- Validity
- Accuracy
- Completeness
- Consistency
- Uniqueness
- Timeliness
- Relevance
Let us look into each of the above aspects in brief:
1. Validity #
The principle of validity emphasizes that data should adhere to a specified format and should fall within acceptable value ranges. For instance, a date of birth field shouldn’t contain text or a negative number.
2. Accuracy #
This rule underscores the need for data to accurately reflect the real-world entity or situation they represent. An error in a single data entry, such as a mistyped email address, could lead to significant miscommunication or lost opportunities.
3. Completeness #
Each required data field should contain a value, ensuring there are no gaps in information. For example, a patient’s medical record might be deemed incomplete if it lacks information about their medical history or allergies.
4. Consistency #
Consistency requires that data across all systems and platforms maintain a uniform format and definition. Discrepancies in data representation can lead to misinterpretations and incorrect analyses.
5. Uniqueness #
This rule highlights the importance of eliminating redundancies to ensure each record in the database is unique. Duplicate entries not only waste storage resources but can also distort analytical results.
6. Timeliness #
Data must be recorded and updated promptly to retain its relevance and utility. Data that is outdated can be misleading and result in erroneous decision-making.
7. Relevance #
This principle mandates that data should be pertinent and applicable to the task at hand. Extraneous data, while might seem harmless, can clutter databases and complicate the process of data analysis.
Enforcing these data quality rules is a pivotal part of a comprehensive data governance strategy. It involves setting clear policies about who is accountable for maintaining data quality, defining how data quality will be measured and tracked, and establishing procedures to correct data quality issues. By prioritizing these rules, organizations can better leverage their data, ultimately driving more accurate insights, better decision-making, and improved overall business performance.
Implementing data quality tools for effective data governance: A step-by-step guide #
Creating a comprehensive data governance program with the goal of quality data can be quite challenging. That’s why we put together a step-by-step guide on what to consider, what to implement, and how to make a business case for it:
- Step 1: Define the problem and goals
- Step 2: Identify the required tools and technologies
- Step 3: Evaluate and select tools and technologies
- Step 4: Make a business case
- Step 5: Implement the tools and technologies
- Step 6: Monitor and improve
Now, let us look into each of the above steps in detail:
Step 1: Define the problem and goals #
The first step in any data governance initiative is to clearly define what problems you’re trying to solve and what your goals are. In your case, the goal is to improve data quality. It’s also helpful to quantify the problem and the goal as much as possible.
For instance, you might say, “We have identified that 5% of our patient records contain errors. Our goal is to reduce this to less than 1%.”
Step 2: Identify the required tools and technologies #
Based on the problems and goals you’ve identified, you can start researching the tools and technologies that can help. You’ll likely need a combination of data quality tools (for data cleansing, validation, etc.), data integration tools (for combining data from different sources), and possibly also data governance tools (for managing metadata, tracking data lineage, etc.).
Step 3: Evaluate and select tools and technologies #
Now you’ll need to evaluate the different options based on their features, ease of use, compatibility with your existing systems, cost, and other factors. This may involve requesting demos or trial versions, reading reviews, and speaking with other users.
Step 4: Make a business case #
Once you’ve identified the best tools and technologies for your needs, you’ll need to make a business case for implementing them. Here are some points to include:
- Cost of poor data quality
Start by quantifying the cost of poor data quality in terms of wasted time, inaccurate reporting, poor decision-making, compliance risks, etc. This will help decision-makers understand why the status quo is not acceptable.
- Benefits of improved data quality
Then, articulate the benefits of improved data quality. This could include more accurate reporting, better decision-making, reduced compliance risk, improved patient care, and others.
- ROI of the proposed tools and technologies
Now present the return on investment (ROI) of the proposed tools and technologies. This should include both the costs (purchase price, implementation costs, ongoing costs) and the expected benefits (time saved, improved decision-making, reduced risk, etc.). Make sure to show how the benefits outweigh the costs.
- Risk mitigation
Discuss how the proposed tools and technologies can mitigate the risk of poor data quality, such as compliance risks, reputational risks, and operational risks. This can be especially persuasive for decision-makers in healthcare, where data errors can have serious consequences.
Step 5: Implement the tools and technologies #
Once you’ve got approval, you can start implementing the chosen tools and technologies. This will likely involve a project plan, resource allocation, and a roll-out strategy.
Step 6: Monitor and improve #
Finally, once the tools and technologies are in place, you’ll need to monitor their effectiveness and make improvements as needed. This could involve tracking key metrics, conducting regular audits, and seeking feedback from users.
Remember, data governance and data quality are ongoing efforts, not one-time projects. You’ll need to keep monitoring and improving over time to maintain high data quality and adjust to changing needs and circumstances.
Bringing it all together #
We hope this guide helped you gain a comprehensive understanding of the significance of data quality within the framework of data governance.
By following these steps and principles, your organization can ensure that its data governance program effectively supports and enhances data quality.
This, in turn, can lead to more accurate reporting, better decision-making, reduced compliance risk, improved patient care, and other benefits.
Data quality in data governance: Related reads #
- What is Data Governance? Its Importance, Principles & How to Get Started?
- How to Improve Data Quality: Strategies and Techniques to Make Your Organization’s Data Pipeline Effective
- Data Quality Measures: A Step-by-Step Implementation Guide
- The Benefits of GDPR Compliance and Data Governance: Protecting Your Data and Your Business
- Key Objectives of Data Governance: How Should You Think About Them?
- Data Governance Framework — Examples, Templates, Standards, Best Practices & How to Create One?
- Data Governance and Compliance: Act of Checks & Balances
- How to implement data governance? Steps, Prerequisites, Essential Factors & Business Case
- How to Improve Data Governance? Steps, Tips & Template
- 7 Steps to Simplify Data Governance for Your Entire Organization
- Snowflake Data Governance — Features, Frameworks & Best Practices
- Automated Data Governance: How Does It Help You Manage Access, Security & More at Scale?
- Enterprise Data Governance — Basics, Strategy, Key Challenges, Benefits & Best Practices
- Data Governance in Manufacturing: Steps, Challenges, and Practical Examples
- Data Governance in Retail: Best Practices, Challenges, and Viable Solutions
- Data Governance in Insurance: Why is it Important and How it Drives Positive Business Outcomes
Share this article