Data Governance in Manufacturing: Steps, Challenges, and Practical Examples
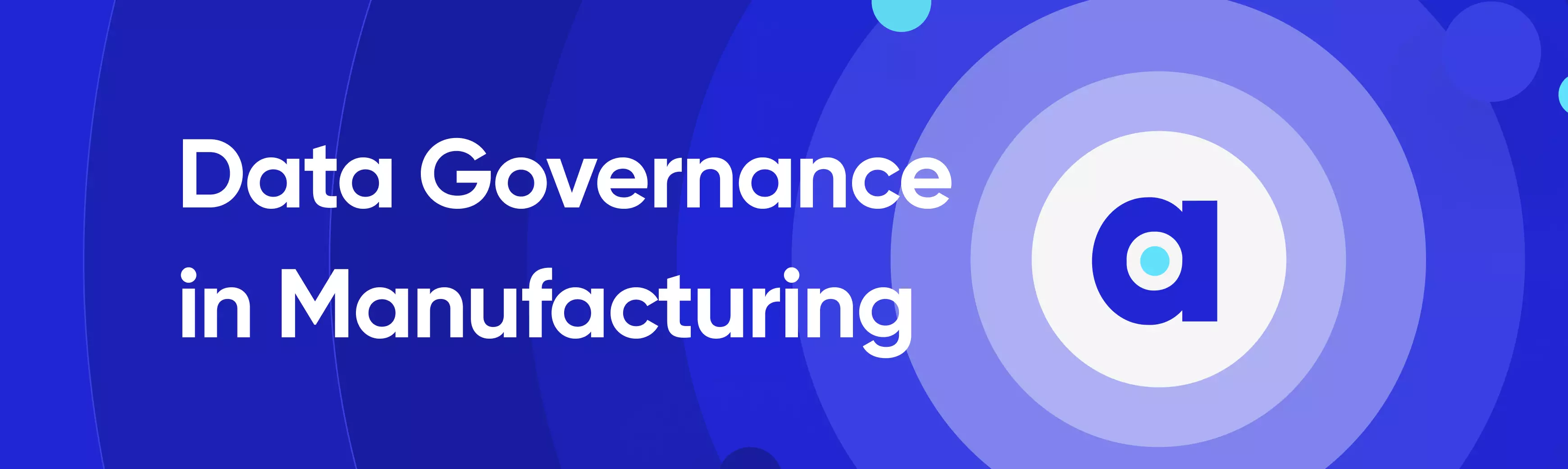
Share this article
Data governance is crucial in the manufacturing industry for several reasons. As manufacturing processes become increasingly data-driven, effective data governance ensures data accuracy, reliability, and consistency, which are essential for driving critical outcomes.
Table of contents #
- How data governance can improve manufacturing operations: 6 Key aspects
- Practical applications of data governance in manufacturing industry
- Implementing a data governance strategy in manufacturing: A step-by-step guide
- 10 Common challenges faced in implementing data governance in the manufacturing industry
- Rounding it all up
- Data governance in manufacturing: Related reads
How data governance can improve manufacturing operations: 6 Key aspects #
Here are some key aspects of data governance in the manufacturing industry:
- Quality control and assurance
- Supply chain management
- Regulatory compliance
- Operational efficiency
- Decision-making and innovation
- Data security and privacy
Let us look into each of the above aspects in detail:
1. Quality control and assurance #
Data governance helps to maintain high-quality data, enabling manufacturing companies to identify and address issues in production processes quickly. Accurate data allows for better monitoring, leading to improved quality control and assurance, reducing the number of defects and waste.
2. Supply chain management #
Manufacturing industries rely heavily on complex supply chains. Effective data governance ensures that data is consistently and accurately shared among different partners, allowing for better visibility, forecasting, and coordination. This helps to reduce lead times, optimize inventory levels, and improve overall supply chain efficiency.
3. Regulatory compliance #
Manufacturing industries are subject to strict regulations, especially when it comes to product safety and environmental standards. Robust data governance helps organizations maintain compliance by tracking and reporting the necessary data and ensuring that data is accurate and up-to-date.
4. Operational efficiency #
Data governance enables the streamlining of manufacturing processes by providing a single source of truth for all data. This helps to reduce data silos, improve collaboration, and automate manual tasks. As a result, companies can reduce downtime, improve productivity, and minimize operational costs.
5. Decision-making and innovation #
With a strong data governance framework in place, manufacturing companies can gain valuable insights from their data, leading to better decision-making and increased innovation. This includes identifying areas for process improvements, product development, and new market opportunities.
6. Data security and privacy #
Manufacturing companies often deal with sensitive data, such as intellectual property and customer information. Effective data governance helps to protect this data by implementing strict security measures, access controls, and privacy policies, reducing the risk of data breaches and ensuring compliance with data protection regulations.
In summary, data governance plays a critical role in the manufacturing industry by ensuring data quality, enabling better decision-making, improving operational efficiency, and maintaining regulatory compliance. As a result, manufacturing companies with robust data governance structures can drive better outcomes, reduce costs, and gain a competitive edge in the market.
Practical applications of data governance in manufacturing industry #
In this section, we explore six practical examples of how manufacturing companies can benefit from data governance, ranging from quality control and assurance to data security and privacy. These examples demonstrate the importance of a comprehensive data governance strategy in driving operational efficiency, regulatory compliance, and innovation.
Let us look into each of the above key aspects with practical examples in detail:
1. Quality control and assurance #
A manufacturing company uses a data governance framework to ensure that data collected from production lines is accurate, consistent, and up-to-date. This allows them to identify patterns and trends in real-time, enabling them to address quality issues quickly.
For example, they may discover that a particular machine is producing a higher-than-normal rate of defects. By addressing this issue promptly, they can reduce waste, improve product quality, and enhance customer satisfaction.
2. Supply chain management #
A manufacturer with strong data governance practices can accurately track inventory levels and share data seamlessly with suppliers and distributors. This ensures that all parties have a clear understanding of the current supply and demand, enabling them to make better decisions about production levels and distribution routes. As a result, the company can optimize its inventory levels, reduce stockouts and overstocks, and improve overall supply chain efficiency.
3. Regulatory compliance #
A pharmaceutical manufacturer implements a data governance program to manage their clinical trial data, ensuring it is accurate, complete, and stored securely. By doing so, they can easily provide regulators with the required data and documentation for approval processes. This not only helps them maintain compliance but also accelerates the time-to-market for new drugs, benefiting both the company and patients.
4. Operational efficiency #
An automotive manufacturer uses a data governance framework to consolidate and streamline data from various sources, such as design, production, and sales departments. By creating a single source of truth, they can eliminate data silos, reduce manual tasks, and improve collaboration between departments. This results in reduced downtime, increased productivity, and more efficient use of resources.
5. Decision-making and innovation #
A food and beverage manufacturer with a robust data governance program can analyze data on consumer preferences, market trends, and competitor products. This enables them to identify opportunities for product development or process improvements. For example, they may discover a growing trend for plant-based protein alternatives, leading them to invest in research and development for new product lines, helping them stay ahead of the competition.
6. Data security and privacy #
A consumer electronics manufacturer implements a data governance strategy that includes strong security measures, access controls, and privacy policies. As a result, they can better protect sensitive data, such as intellectual property and customer information. This reduces the risk of data breaches, maintains trust with customers and partners, and ensures compliance with data protection regulations.
In each of these hypothetical examples, data governance plays a crucial role in ensuring positive outcomes for the manufacturing company, ranging from improved product quality to better decision-making and enhanced data security.
Implementing a data governance strategy in manufacturing: A step-by-step guide #
Implementing a data governance strategy in a manufacturing organization involves several steps.
Here’s a recommended approach to ensure a successful implementation:
- Secure executive sponsorship
- Establish clear goals and objectives
- Create a data governance team
- Develop a data governance framework
- Conduct a data inventory and assessment
- Implement data governance tools and technologies
- Develop a training and education program
- Monitor and measure progress
- Foster a data-driven culture
- Continuously improve
Let us look into each of the above steps in detail:
1. Secure executive sponsorship #
Gaining support from top-level management is crucial for the success of any data governance initiative. Executive sponsorship ensures the necessary resources, budget, and authority to drive the program forward.
2. Establish clear goals and objectives #
Define the specific outcomes your organization aims to achieve with data governance. These goals should align with the organization’s overall business strategy and address the most pressing data-related challenges.
3. Create a data governance team #
Assemble a cross-functional team with representatives from various departments, such as IT, operations, quality control, and finance. This team will be responsible for driving the data governance program, ensuring collaboration and coordination across the organization.
4. Develop a data governance framework #
Create a comprehensive data governance framework that outlines the policies, procedures, roles, and responsibilities for data governance. This should include elements such as data quality standards, data lineage, data access and security policies, and data stewardship roles.
5. Conduct a data inventory and assessment #
Identify the critical data assets within your organization and evaluate their current state. This assessment will help you understand the gaps and issues that need to be addressed in your data governance strategy.
6. Implement data governance tools and technologies #
Invest in appropriate tools and technologies to support data governance efforts, such as data cataloging, data quality management, and data lineage tracking tools. These tools can help automate processes and improve overall data governance efficiency.
7. Develop a training and education program #
Educate employees on the importance of data governance, their roles and responsibilities, and the processes and tools involved. Regular training and communication will help ensure adherence to the data governance policies and procedures.
8. Monitor and measure progress #
Establish key performance indicators (KPIs) to track the effectiveness of your data governance program. Regularly monitor and evaluate these KPIs to identify areas for improvement and to demonstrate the value of data governance to stakeholders.
9. Foster a data-driven culture #
Encourage a mindset that values data quality, accuracy, and consistency throughout the organization. This will help embed data governance practices into the day-to-day operations and decision-making processes.
10. Continuously improve #
Data governance is an ongoing process. Regularly review and update your data governance strategy to ensure it remains aligned with evolving business needs, industry standards, and regulatory requirements.
By following these steps, you can implement a successful data governance strategy in a manufacturing organization, ensuring better data quality, improved decision-making, and enhanced compliance with industry regulations.
10 Common challenges faced in implementing data governance in the manufacturing industry #
Implementing data governance in a manufacturing organization can present several challenges. Anticipating and addressing these challenges is crucial for a successful data governance program.
Here are some common challenges and ways to mitigate them:
- Lack of executive support
- Organizational resistance
- Data silos
- Inadequate data quality
- Insufficient resources
- Undefined roles and responsibilities
- Regulatory compliance
- Technology integration
- Scalability
- Continuous improvement
Let us look into each of the above challenges in detail:
1. Lack of executive support #
Without a commitment from top-level management, data governance initiatives may struggle to secure the necessary resources and authority. To mitigate this, present a clear business case outlining the benefits of data governance and how it aligns with the organization’s strategic goals.
2. Organizational resistance #
Implementing data governance can lead to resistance from employees who may perceive it as additional work or a threat to their autonomy. To address this, communicate the benefits of data governance to all stakeholders and involve them in the planning and implementation process. Provide adequate training and support to help employees adapt to the new processes.
3. Data silos #
Manufacturing organizations often have data stored in disparate systems and formats, leading to data silos and inconsistencies. To tackle this issue, establish a centralized data repository or implement data integration tools to consolidate and standardize data across the organization.
4. Inadequate data quality #
Poor data quality can undermine the effectiveness of a data governance program. Address this by implementing data quality management processes, such as data validation, cleansing, and enrichment. Regularly monitor data quality metrics to identify and rectify issues.
5. Insufficient resources #
Implementing data governance may require additional resources, such as personnel, technology, and budget. To overcome this, prioritize data governance initiatives based on their potential impact and allocate resources accordingly. Consider leveraging external expertise or outsourcing certain tasks if necessary.
6. Undefined roles and responsibilities #
Ambiguity in roles and responsibilities can hinder data governance efforts. Clearly define the roles, responsibilities, and accountabilities of various stakeholders involved in the data governance program, including data stewards, data owners, and data custodians.
7. Regulatory compliance #
Keeping up with changing regulatory requirements can be challenging. Establish a robust compliance management process that monitors regulatory changes and ensures timely updates to your data governance policies and procedures.
8. Technology integration #
Integrating data governance tools with existing systems can be complex. Collaborate with IT teams to ensure seamless integration and compatibility with existing systems. Test the tools thoroughly before deployment to avoid disruptions to ongoing operations.
9. Scalability #
As the organization grows and evolves, so will its data governance needs. Design your data governance framework to be scalable and flexible, allowing for adjustments and expansions as required.
10. Continuous improvement #
Data governance is an ongoing process that requires regular monitoring and adjustment. Establish a feedback loop and mechanisms for continuous improvements, such as periodic reviews, audits, and stakeholder feedback.
By anticipating these challenges and implementing strategies to address them, you can increase the likelihood of a successful data governance program in a manufacturing organization.
Rounding it all up #
The manufacturing industry is increasingly data-driven, and data governance is essential to ensure accuracy, reliability, consistency, and compliance with regulations.
Effective data governance can improve manufacturing operations in six key aspects: quality control, supply chain management, regulatory compliance, operational efficiency, decision-making and innovation, and data security and privacy.
Data governance ensures that data is consistent, accurate, and up-to-date, enabling manufacturing companies to optimize inventory levels, reduce waste, streamline processes, and make better decisions.
If you are evaluating and looking to deploy best-in-class data access governance for the modern data stack without compromising on data democratization, do give Atlan a spin.
Atlan is a third-generation data catalog built on the premise of embedded collaboration that is key in today’s modern workplace, borrowing principles from GitHub, Figma, Slack, Notion, Superhuman, and other modern tools that are commonplace today.
Data governance in manufacturing: Related reads #
- What is Data Governance? Its Importance, Principles & How to Get Started?
- Key Objectives of Data Governance: How Should You Think About Them?
- Data Governance Framework — Examples, Templates, Standards, Best Practices & How to Create One?
- Data Governance and Compliance: Act of Checks & Balances
- How to implement data governance? Steps, Prerequisites, Essential Factors & Business Case
- How to Improve Data Governance? Steps, Tips & Template
- 7 Steps to Simplify Data Governance for Your Entire Organization
- Snowflake Data Governance — Features, Frameworks & Best Practices
- Automated Data Governance: How Does It Help You Manage Access, Security & More at Scale?
- Enterprise Data Governance — Basics, Strategy, Key Challenges, Benefits & Best Practices
- Data Governance in Insurance: 7 Ways It Impacts Insurers
Share this article