DevOps vs. Data Engineer: Clash of the Tech Titans
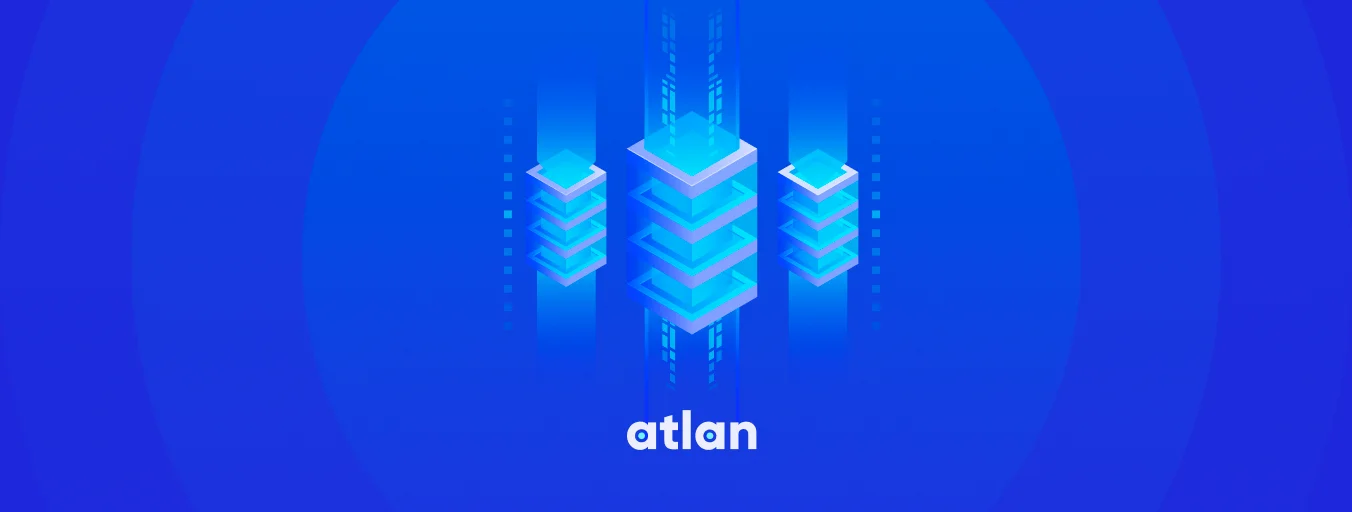
Share this article
Did you know that over 70% of companies today use both devOps and data engineering practices in some capacity? The burning question remains: “devOps vs. data engineer - which is more important?” In layman’s terms, devOps folks streamline software delivery, while data engineers sort and manage heaps of data.
The crux of the matter lies in their distinct approaches: while devOps focuses on creating seamless software delivery processes, data engineers specialize in structuring vast amounts of data for easy access and interpretation. This whole “devOps vs. data engineer” chat is more than just tech talk. It’s about finding out what’s best for businesses in our digital world.
Modern data problems require modern solutions - Try Atlan, the data catalog of choice for forward-looking data teams! 👉 Book your demo today
In this article, we will explore:
- Breakdown of devOps and data engineers.
- Insights into potential earnings.
- A glimpse at easier role to learn.
- How data engineers adopt devOps.
- More about these tech role giants.
So, let’s dive in!
Table of contents #
- What is DevOps?
- Who is a data engineer?
- DevOps vs. data engineer: 15 Core differences
- Analyzing salaries: DevOps vs. data engineer
- Which is easier to learn: DevOps or data engineering?
- DevOps vs data engineer: Which is better in the tech arena?
- How data engineers are adopting devOps strategies?
- Conclusion
- Related reads
What is DevOps? #
DevOps is a fusion of “development” and "operations”. It is a cultural and technical movement that emphasizes collaboration between software developers and IT operations teams.
It aims to bridge the traditional divide between these groups, fostering an environment where software can be designed, tested, and released more rapidly, frequently, and reliably.
At its core, devOps seeks to automate many of the manual processes involved in the software delivery lifecycle. By doing so, not only accelerates software releases but also enhances their quality and reliability.
Adopting a devOps culture means prioritizing continuous integration, continuous delivery, automation, and consistent feedback loops, ensuring that software changes are continuously moving from development to production smoothly and efficiently.
Who is a data engineer? #
A data engineer is a specialized role responsible for designing, constructing, and maintaining the architecture of large-scale processing systems, as well as the complex, large datasets used in operational and analytical applications.
Their primary goal is to provide a reliable and optimized infrastructure for data, making it easily accessible and usable for data scientists, analysts, and other stakeholders.
This often involves building and maintaining data pipelines, transforming data into a usable format, and ensuring data integrity and consistency throughout its lifecycle.
In the age of big data, data engineers play a pivotal role in a company’s ability to make data-driven decisions. Their expertise in database systems, data warehousing solutions, and big data technologies ensures that businesses can harness the full potential of their data assets.
DevOps vs. data engineer: 15 Core differences #
In the dynamic world of technology, the roles of DevOps and data engineers often intersect, yet they remain distinct in many crucial ways. Both positions serve as essential cogs in modern business machinery, particularly in the SaaS realm. But, what truly sets them apart?
Here, we’ll delve into 15 core differences that differentiate these two pivotal tech roles in a tabular format:
Aspect | DevOps | Data Engineer |
---|---|---|
Primary Objective | Ensures rapid, reliable software delivery and deployment. | Manages and optimizes the data infrastructure, ensuring data is accessible and usable. |
Main focus | Software development lifecycle, including integration, testing, and deployment. | Data lifecycle, from ingestion to storage, processing, and accessibility. |
Tool familiarity | Often works with CI/CD tools like Jenkins, Docker, and Kubernetes. | Commonly uses big data tools like Hadoop, Spark, and SQL-based systems. |
Collaboration | Works closely with both software developers and IT operations teams. | Collaborates with data scientists, analysts, and sometimes with IT operations. |
Coding | Primarily scripts for automation, infrastructure as code, and sometimes application development. | Writes complex data transformation and processing scripts, designs database systems. |
Skillset emphasis | Strong focus on systems, infrastructure, and deployment automation. | Deep understanding of databases, data modeling, and big data technologies. |
Role evolution | Emerged from the need to bridge the gap between development and operations. | Originated from the increasing need to handle, process, and make sense of large data sets. |
Main challenge | Keeping software deployable at any time and reducing software delivery time. | Ensuring data reliability, and consistency, and optimizing data processing tasks. |
Performance metrics | Often measured by deployment frequency, software stability, and recovery times. | Evaluated based on data quality, processing efficiency, and infrastructure reliability. |
Learning curve | Involves understanding both development and IT operations, alongside CI/CD principles. | Centers around mastering database systems, ETL processes, and big data frameworks. |
Future trends | Movement towards gitOps, noOps, and increased focus on security (devSecOps). | Emphasis on real-time data processing, dataOps, and integration with machine learning workflows. |
End product | Reliable software and applications, delivered quickly and efficiently. | Clean, consistent, and easily accessible datasets ready for analysis and insights. |
Career transition | Often transitions from either a software development or system admin background. | Might start as a database administrator, backend developer, or data analyst before specializing. |
Knowledge base | Needs a holistic understanding of the software development process and IT infrastructure. | Requires deep insights into data warehousing, data modeling, and the data ecosystem. |
Typical day-to-day | Involves setting up CI/CD pipelines, monitoring software deployments, and infrastructure tweaking. | Centers around constructing data pipelines, optimizing database queries, and managing data storage solutions. |
While devOps and data engineers might sometimes find their paths crossing, especially in the rapidly evolving tech landscape, their core functions and areas of expertise remain distinct.
Whether you’re a budding tech enthusiast trying to find your niche or a business leader looking to hire, understanding these differences is paramount.
Remember, in the grand theatre of technology, both devOps and data engineers have unique and essential roles to play – and recognizing their individual strengths can be the foundation to success.
Analyzing salaries: DevOps vs. data engineer #
In the rapidly evolving tech industry, where roles are continually refined and redefined, understanding the financial value associated with each position can be crucial for both professionals and employers.
Two roles that often draw curiosity in salary discussions are those of DevOps and Data Engineers. Both of these roles play instrumental parts in modern businesses, especially in the SaaS domain.
Here are the nuances of the salaries attached to these roles:
- Baseline salaries
- Geographic variations
- Experience and specialization
- Industry and sector
- Skillset and certifications
- Scope of responsibility
- Demand and supply dynamics
- Educational background
- Company size and funding
Let’s look into each of the above nuances in brief:
1. Baseline salaries #
Generally, both devOps and data engineers command impressive baseline salaries, reflective of the critical roles they play. According to various industry surveys and platforms, the average salary for devOps engineers in the U.S. tends to slightly edge out that of data engineers.
However, the difference isn’t significant and varies based on factors such as experience, location, and industry.
2. Geographic variations #
Location plays a pivotal role in determining salaries for both roles. For instance, tech hubs like Silicon Valley, Seattle, and New York often offer higher salaries compared to other regions, thanks to the concentration of tech companies and the high cost of living.
A devOps engineer in San Francisco might earn notably more than one in a mid-western city, and the same goes for data engineers.
3. Experience and specialization #
As with most professions, experience can significantly impact the earning potential.
A senior data engineer with expertise in specific big data technologies or a devOps professional with years of experience in managing complex deployment pipelines can command salaries considerably higher than their entry-level counterparts.
4. Industry and sector #
The industry or sector in which a professional works can also influence salaries.
For instance, devOps or data engineering roles in industries like finance or healthcare might offer higher salaries than, say, the education sector, due to the critical nature of data and deployment in these industries.
5. Skillset & certifications #
Beyond the foundational skills required for each role, possessing niche or highly sought-after skills can boost earning potential. For devOps, skills in newer deployment technologies or certifications from cloud providers can add a premium.
Similarly, data engineers skilled in emerging data technologies or with certifications in specific data platforms can command higher salaries.
6. Scope of responsibility #
The breadth and depth of responsibilities can influence salary. A devOps engineer responsible solely for managing deployment might earn differently than one overseeing an entire infrastructure, including security (devSecOps).
Similarly, a data engineer tasked with not just constructing data pipelines but also strategizing data storage solutions could earn more.
7. Demand and supply dynamics #
The tech industry is greatly influenced by the dynamics of demand and supply. If there’s a surge in demand for professionals skilled in a specific new technology, but a limited supply of such professionals, it can drive salaries upwards.
This dynamic can be observed in both the devOps and data engineering realms as technologies and methodologies evolve.
8. Educational background #
In the world of technology, hands-on experience often takes precedence, but one cannot undermine the importance of a solid educational foundation. Holding a degree from a reputed institution can be an added advantage during salary negotiations.
Moreover, advanced degrees such as a master’s or PhD, especially in relevant fields like computer science or data science, can provide an edge.
Additionally, continual training and certifications specific to the roles - for instance, cloud certifications for devOps or big data platform certifications for data engineers - can bolster one’s salary prospects, highlighting a commitment to professional growth and expertise in the domain.
9. Company size and funding #
The size of the company and its funding status can also play a decisive role in determining salaries. Startups, especially early-stage ones, might offer lower base salaries but compensate with stock options or other benefits, anticipating future growth.
On the contrary, established tech giants or well-funded unicorns typically have the resources to offer competitive, top-tier salaries to attract and retain talent.
For both devOps and data engineers, joining a startup might mean a trade-off between immediate monetary compensation and potential future rewards, while large corporations offer stability and immediate financial benefits.
Breaking down salaries for devOps and data engineers shows many factors at play, from education to company size. It’s essential for both tech professionals and companies to understand these to make informed decisions. In a constantly changing tech world, staying updated and adaptable is key to ensuring fair compensation and attracting the best talent.
Which is easier to learn: DevOps or data engineering? #
In the vast realm of technology, these two roles have increasingly drawn attention due to their pivotal impact on modern business processes: devOps and data engineering.
For those looking to pivot their careers or just starting in tech, a common query is which of the two is easier to learn. Determining the “ease” of learning is subjective and hinges on various aspects, from one’s foundational skills to the resources at one’s disposal.
Here are the factors that might make one seem more approachable than the other:
- Foundational knowledge
- Learning resources available
- Breadth vs. depth
- Evolving technologies
- Community and peer support
- Practical experience
- Tangibility of results
- Problem-solving approach
- Industry demand
Let’s look into each of the above factors in brief:
1. Foundational knowledge #
Diving into devOps typically requires a grounding in software development and system operations. If someone already has experience in software development, understanding the continuous integration and deployment processes might be smoother.
On the other hand, data engineering leans heavily on database management, data processing frameworks, and often a bit of programming. Hence, for those with a background in database systems, data engineering might seem more accessible.
2. Learning resources available #
The availability of learning resources can influence the ease of mastering a domain. DevOps, being a relatively newer field, might not have as extensive a range of traditional educational resources as data engineering.
However, the internet is rife with forums, online courses, and community-led initiatives that offer insights into devOps practices. Data engineering, due to its longevity, benefits from a blend of traditional academic courses and modern online resources.
3. Breadth vs. depth #
DevOps covers a broader spectrum, spanning coding, infrastructure management, integration, deployment, and sometimes security. It’s more about understanding the entire software delivery pipeline.
Data engineering, while deep, focuses more on the efficient collection, storage, and processing of data. Its depth lies in mastering specific tools and processes related to data.
4. Evolving technologies #
Both fields are continually evolving, but the pace might differ. DevOps sees frequent updates with the emergence of new tools and best practices, given the push towards automation and seamless software delivery.
Data engineering, while evolving, might be perceived as more stable in terms of the foundational technologies, though new data processing and storage methods do emerge.
5. Community and peer support #
The strength and willingness of a community to support newcomers can influence ease of learning. The devOps community is known for its collaborative spirit, with many professionals sharing their experiences, pitfalls, and best practices online.
Data engineering also has a robust community, especially around specific technologies like Apache Kafka or Hadoop.
6. Practical experience #
Gaining hands-on experience in devOps might be more straightforward for someone already in a software development role, as they can start by integrating devOps practices into their workflow.
For data engineering, practical exposure might require more specific tasks or projects, especially those centered around data pipelines and big data technologies.
7. Tangibility of results #
For many learners, seeing tangible results accelerates the learning process. In devOps, the results—like a successful deployment or a streamlined workflow—are often quickly visible.
In data engineering, the fruits of one’s labor, like a well-constructed data pipeline, might take longer to manifest or might be more abstract.
8. Problem-solving approach #
The kind of problems one prefers can guide the ease of learning. DevOps often involves troubleshooting and optimizing deployment pipelines, requiring a macro view of the software delivery process.
Data engineering leans towards addressing challenges in data collection, storage, and processing, necessitating a detail-oriented problem-solving approach.
9. Industry demand #
The demand within the industry can drive the creation of learning resources and opportunities. Both devOps and data engineering are in demand, but regional and industry-specific demands can vary.
For instance, a region with a booming tech startup culture might have a higher demand for devOps professionals, leading to more local resources and learning opportunities.
Deciding whether devOps or data engineering is easier to learn isn’t black and white; it’s a gradient colored by individual backgrounds, preferences, and goals. What might seem straightforward to one person might pose challenges to another.
Ultimately, the passion for the domain, combined with the right resources and perseverance, will define one’s learning journey. For aspirants, it’s about aligning with what resonates most with their interests and long-term career vision.
DevOps vs data engineer: Which is better in the tech arena? #
The rapid evolution of the tech industry brings forth roles that are essential to the contemporary digital ecosystem. Among these roles, devOps and data engineering consistently gather attention due to their integral part in the tech infrastructure.
A common debate that resonates among professionals and enterprises alike is: which is better, devOps or data engineering? While ‘better’ is subjective and dependent upon specific needs and contexts, this topic seeks to shed light on various areas of both domains, enabling a more informed decision-making process.
Here are the aspects to understand which is better in the tech arena:
- Role definition
- Impact on business
- Skillset and learning curve
- Evolving landscape
- Career progression
- Financial compensation
- Industry demand
- Integration with other teams
- Tools and technologies
- Business size and type
Let’s look into each of the above aspects in brief:
1. Role definition #
At its core, devOps amalgamates software development and IT operations to bolster a more efficient and streamlined software delivery process. It champions a culture of collaboration and continuous feedback.
Conversely, data engineers lay the foundation for robust data architecture, facilitating the gathering, storing, and processing of vast data quantities. Their work ensures that data scientists and analysts can efficiently derive insights from this data.
2. Impact on business #
DevOps professionals significantly influence the software delivery pipeline, ensuring products reach the market faster and updates are seamlessly integrated. This agility can be a competitive advantage for businesses.
Data engineers, on the other hand, empower organizations to harness their data’s potential, enabling informed decision-making, predictive analytics, and enhanced customer experiences.
3. Skillset and learning curve #
DevOps demands a comprehensive understanding of software development, system operations, and sometimes even a touch of security. Its practitioners often pivot between various tasks, requiring a versatile skill set.
Data engineers primarily focus on data—its collection, storage, and processing—making their skill set more specialized, revolving around database management systems, big data technologies, and programming.
4. Evolving landscape #
The world of devOps is ever-changing, with new tools, best practices, and methodologies cropping up regularly. It’s a realm of constant adaptation.
Data engineering, while certainly evolving, has established technologies that remain foundational for extended periods, though advancements in data storage and processing are perennial.
5. Career progression #
DevOps practitioners often evolve from roles in software development or system administration. Their career trajectory might lead to positions like devOps architects or even managerial roles overseeing devOps teams.
Data engineers might progress into roles such as data architects or move towards data science, given the right skill augmentation.
6. Financial compensation #
While salaries can vary based on region, experience, and individual companies, both DevOps and data engineering roles are among the higher-paying jobs in the tech industry.
Current market trends often show a competitive salary range for both, with slight variations based on the factors mentioned earlier.
7. Industry demand #
Both devOps and data engineering have robust demand. The push for digital transformation and the need for agility in software delivery drive the demand for devOps.
In contrast, the thriving realization of data as an invaluable asset fuels the need for competent data engineers.
8. Integration with other teams #
DevOps professionals frequently collaborate with development, operations, and sometimes even marketing teams, advocating a culture of shared responsibility.
Data engineers often work closely with data scientists, analysts, and occasionally IT teams, ensuring data availability and integrity.
9. Tools and technologies #
DevOps uses tools designed for continuous integration, continuous delivery, and infrastructure automation. Think about Jenkins, Docker, and Kubernetes.
Data engineers leverage technologies tailored for data storage, processing, and analytics—like Hadoop, Spark, and various database management systems.
10. Business size and type #
Startups looking to quickly launch and iterate their products might prioritize devOps to gain agility in their software delivery.
Larger enterprises, especially those dealing with vast amounts of data, might emphasize the role of data engineering to harness the full potential of their data assets.
Choosing between devOps and data engineering isn’t about which one is “better”. Both are super important in their own way. For folks thinking about their career or businesses deciding what they need more, it’s all about what fits their goals and challenges best. Remember, in tech, teamwork often wins over going solo!
How data engineers are adopting devOps strategies? #
In the tech world, different job roles sometimes overlap and borrow ideas from each other. A good example is how data engineers are using some tools and ways of working from the devOps world. This shows how tech jobs can change and adapt. It also shows that working together and mixing different skills can help businesses stay ahead.
Here’s how data engineers are adopting devOps strategies:
- Embracing automation
- Continuous Integration and Continuous Deployment (CI/CD) for data pipelines
- Collaborative work environment
- Monitoring and logging
- Infrastructure as Code (IaC)
- Scalability and flexibility
- Emphasis on testing
- Feedback loops and iterative development
Let’s take a look at each of the above aspects in brief:
1. Embracing automation #
Data engineers are steadily moving towards automating repetitive tasks. Whether it’s data ingestion, ETL processes, or even data validation, tools that cater to automation, familiar in the devOps world, are becoming indispensable.
Automation reduces errors, accelerates workflows, and ensures consistent data quality – values that resonate with both devOps and data engineering principles.
2. Continuous Integration and Continuous Deployment (CI/CD) for data pipelines #
Much like software developers employ CI/CD for swift and efficient software releases, data engineers are now using CI/CD principles for data pipelines.
This ensures that data pipeline changes are consistently tested and seamlessly integrated, allowing faster reactions to changing business requirements and data structures.
3. Collaborative work environment #
A primary tenet of devOps is fostering collaboration between operations and development. Similarly, data engineers are increasingly working in tandem with data scientists, analysts, and other stakeholders.
Tools like git, once predominantly used by developers, are now becoming prevalent among data engineers to manage version control and promote collaboration.
4. Monitoring and logging #
DevOps places a heavy emphasis on monitoring applications and infrastructure.
In the same vein, data engineers are incorporating real-time monitoring of data pipelines, ensuring they are alerted to any issues, thereby minimizing data processing downtime and maintaining data integrity.
5. Infrastructure as Code (IaC) #
DevOps champions the concept of IaC, where infrastructure is provisioned and managed using code and software development techniques.
Data engineers, managing vast data infrastructures, have begun to adopt this practice, leveraging tools like Terraform or cloud formation to ensure consistent and reproducible infrastructure deployments.
6. Scalability and flexibility #
In the devOps world, scalability ensures applications handle growth efficiently.
Data engineers, facing ever-growing data volumes, are integrating this devOps principle by designing data architectures that can effortlessly scale, often leaning on cloud solutions that can expand as data influx rises.
7. Emphasis on testing #
Robust testing frameworks are a hallmark of devOps to ensure software reliability.
Data engineers are borrowing this ethos, instituting rigorous testing for data pipelines, ensuring data accuracy, and that transformations and integrations occur without hitches.
8. Feedback loops and iterative development #
DevOps thrives on feedback, continuously iterating based on feedback loops. Data engineers, in their journey towards agility, are implementing feedback mechanisms from data consumers like data scientists and business analysts.
This ensures that the data delivered aligns with business needs and that any issues are rapidly addressed.
So, data engineers are indeed using devOps practices in their work. It’s like mixing two recipes and getting something even better. As technology changes, it’s cool to see how these roles learn from each other and improve the way they do things.
Conclusion #
In our journey through the tech landscape, we’ve navigated the nuances of both devOps and data engineering, highlighting their distinct roles, shared practices, and their overlapping realms. It’s evident that as the digital world evolves, these two fields aren’t just operating in their own bubbles. They interact, merge, and influence each other in fascinating ways.
While devOps focuses on smoothing out software development processes, and data engineering zeroes in on managing and optimizing data flow, their intersections reveal a deeper narrative. It’s about the collaborative spirit of the tech community, the shared goal of innovation, and the drive to continually refine processes for efficiency and excellence.
Wrapping things up, whether you lean towards devOps, champion data engineering, or sit somewhere in between, understanding both offers a richer perspective on the tech ecosystem. As these roles continue to weave their narratives, we’ll undoubtedly see more shared practices and innovations emerging from their combinations.
DevOps vs data engineer: Related reads #
- DataOps vs. DevOps: What are the Key Differences?
- What is DataOps? - DataOps Framework & 9 Key DataOps Principles
- What is DataOps & How Does it Help Data Management? | Atlan
- The Rise of DataOps
- 6 Tips to Choose the Best Structure for Your Data Team in 2023
- How to Choose a Data Reliability Engineer: 9 Skills to Look For!
Share this article