How to Improve the Reliability of Your Data in 11 Easy Steps
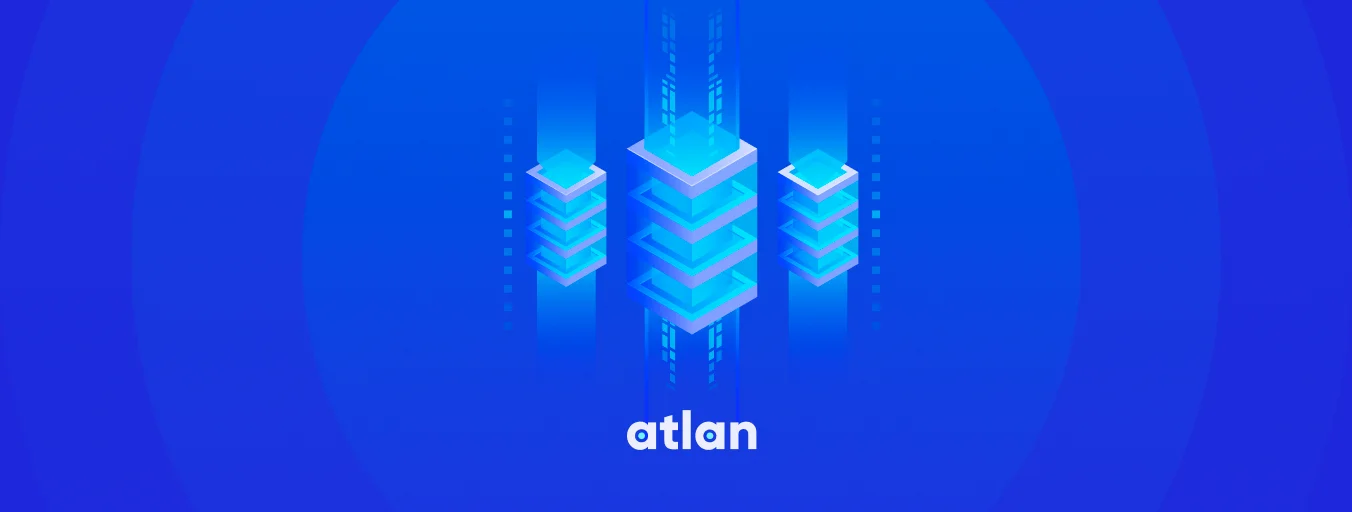
Share this article
Data reliability is a term that encapsulates the trustworthiness and consistency of a dataset. It’s a measure of the dataset’s ability to produce the same results under the same conditions over time. This concept is crucial as organizations increasingly depend on data for strategic decision-making and operational efficiency.
Reliable data is the bedrock of sound decision-making. Organizations today rely heavily on data to guide strategy, allocate resources, and drive growth.
However, data is only as good as its source. Malicious or careless practices can undermine data integrity, leaving businesses vulnerable to risky or unethical choices.
In this article, we will explore the critical factors that determine data reliability and provide best practices to safeguard accuracy across the data lifecycle.
Let’s dive in!
Table of contents #
- 9 Factors that influence data reliability
- 11 Easy steps to ensure validity and reliability of your data
- Summing up
- Related reads
9 Factors that influence data reliability #
Reliability is key to any data-driven decision-making process. Before drawing insights or taking actions based on data, it’s crucial to understand the factors that influence its reliability, which are:
- Data source
- Comprehensive coverage
- Data collection methods
- Data integrity
- Time sensitivity
- Consistency and repeatability
- Data cleaning and preprocessing
- Standardized metrics
- Error management
Let us understand each of these points in detail:
1. Credible data source #
A reliable dataset usually originates from a credible, verified source with a reputation for producing accurate information. Credibility is often established through peer reviews, expert validations, or adherence to industry standards.
Information from government agencies, academic institutions, or reputable private organizations is generally considered more reliable. Verify the credibility by cross-referencing information or through triangulation methods. A credible source substantially increases the likelihood that a dataset is reliable.
Data source quality is pivotal for reliability, opt for sources with a proven track record in credibility and accuracy. A poorly chosen source can introduce errors or biases. Ensure the source’s data is well-maintained and secure to preserve data integrity. Validate the source’s methodology for data collection.
2. Comprehensive coverage #
A reliable dataset should comprehensively cover the scope and dimensions of the phenomenon being studied. Comprehensive datasets offer balanced representation, minimizing biases and ensuring all key variables are included.
The dataset should be large enough to be statistically significant, improving the generalizability of findings. Small or selective datasets risk introducing biases that undermine reliability.
3. Data collection methods #
The method by which you collect data has a direct impact on its reliability. Be wary of sampling biases, especially in surveys or observational studies. The tools and instruments used should be regularly calibrated to ensure their accuracy.
Poorly designed collection methods can contaminate even the best data sources. Always opt for methods that have been rigorously tested.
4. Data integrity #
Data integrity refers to the accuracy and consistency of data over its entire lifecycle. This is ensured through robust security measures, regular audits, and checksum validations. Data integrity guarantees that the data hasn’t been tampered with or altered in unauthorized ways.
A compromise in data integrity significantly affects its reliability. Use cryptographic hashing or digital fingerprinting to maintain integrity.
5. Time sensitivity #
Data often has a temporal component that influences its reliability. Outdated data might no longer be relevant for current decision-making. On the other hand, some data might have seasonal fluctuations that can mislead if not properly accounted for. Always check the timestamp and consider the data’s shelf life for your specific use case.
6. Consistency and repeatability #
In a reliable dataset, similar measurements under similar conditions should produce similar outcomes. Consistency can be verified using statistical methods such as test-retest reliability or intra-class correlation.
Repeated measurements should yield results within a narrow, acceptable range of variance. Inconsistent data points can significantly distort findings and conclusions. Frequent inconsistencies across observations are red flags signaling unreliability.
Consistency in data collection methods is crucial for longitudinal studies and time-series analysis. Develop Standard Operating Procedures (SOPs) for data collection to ensure uniformity. Staff involved in data collection should be trained to adhere to these standards. Consistency makes your data repeatable and therefore more reliable.
7. Data cleaning and preprocessing #
Garbage in, garbage out: unreliable data stays unreliable if not properly cleaned and preprocessed. Inconsistent or missing data should be treated with care, through removal or interpolation.
Data from different sources should be normalized to a common scale. Invest time in quality assurance steps like data validation and outlier detection. Cleaning can make or break your dataset’s reliability.
8. Standardized metrics #
A reliable dataset employs standardized units of measurement, terminologies, and data formats. Standardization ensures that data is comparable across different times, settings, or observers. Adhering to industry standards or scientific norms enhances the dataset’s reliability.
Non-standard metrics can introduce interpretational errors or inconsistencies. Always validate the metrics against known standards or benchmarks.
9. Error management #
A reliable dataset has well-documented error margins and uncertainty levels. Error management involves strategies to detect, correct, or eliminate errors and outliers during data collection and processing.
Recognizing the existence of errors and accounting for them increases the dataset’s reliability. Tools like anomaly detection algorithms can automate error management. Always document any errors found and the corrective actions taken to improve transparency and trust.
Understanding and addressing these factors will significantly enhance the reliability of your data, providing a solid foundation for decision-making and analysis. Ensuring data reliability isn’t just a one-time activity but an ongoing commitment.
A reliable dataset is not an accident but a result of careful planning, rigorous methods, and vigilant oversight. By paying attention to these six core elements—source, coverage, integrity, consistency, standardization, and error management—you’re well on your way to ensuring your dataset stands up to scrutiny and serves as a reliable foundation for decision-making.
11 Easy steps to ensure validity and reliability of your data #
Data validity and reliability are foundational elements for informed decision-making. They are not just buzzwords but crucial criteria that significantly influence the quality of your analysis and, consequently, the actions taken.
- Establish a robust data governance framework
- Implement data governance policies
- Data auditing
- Use validated data collection instruments
- Adopt robust data collection techniques
- Enhance data storage and security
- Apply statistical tests for reliability
- Leverage automation for quality control
- Continuous training and skill enhancement
- Continuous monitoring
- Establish feedback mechanisms
Let us understand each of these points in detail
Step 1: Establish a robust data governance framework #
- A data governance framework provides guidelines and protocols for data management. It standardizes the data collection, storage, and access methods, ensuring everyone follows the same procedures.
- The framework can help you maintain data lineage, allowing for traceability of how data is manipulated over time.
- Implementing strong data governance policies helps in ensuring data security, quality, and, thus, reliability. Compliance with regulatory standards like GDPR or HIPAA can also be monitored via this framework.
Step 2: Implement data governance policies #
- Data governance sets the rules and protocols for data management and usage. Well-articulated policies can establish standardized methods for data collection, storage, and retrieval.
- Data governance ensures compliance with legal regulations and ethical standards, thereby improving reliability. These policies should be revisited and updated periodically to adapt to technological advancements or regulatory changes.
- Strong governance acts as the backbone of data reliability, giving stakeholders confidence in the dataset.
Step 3: Data auditing #
- Perform regular audits to assess the quality and reliability of the data. Audits examine the data’s source, how it was collected, and how it has been used or changed over time.
- Tools like checksums and data fingerprinting can be used to ensure data hasn’t been tampered with.
- Audits often expose weak links in the data pipeline that can then be addressed. Make data audit reports accessible to decision-makers and stakeholders.
Step 4: Use validated data collection instruments #
- Using validated instruments like well-designed surveys, calibrated sensors, or trusted software is key to data validity. Validated tools have been tested for accuracy and can produce results that are both repeatable and representative.
- Reliability and validity coefficients should be publicly available for these instruments. Regularly update and recalibrate these tools to ensure they maintain their standards.
- Always pilot-test new tools before fully integrating them into your data collection process.
Step 5: Adopt robust data collection techniques #
- Data collection is where the journey to reliability begins. Adopting proven methodologies such as stratified sampling or randomized controlled trials can improve the reliability of the collected data.
- Calibration of measurement instruments ensures accuracy, thereby enhancing reliability. A pilot phase to test collection methods can identify potential errors or biases upfront.
- Cross-reference the collected data with other reliable sources to ensure validity and reliability.
Step 6: Enhance data storage and security #
- Data corruption or unauthorized alteration significantly compromise reliability. Invest in secure, redundant storage solutions that offer real-time backups.
- Data encryption and multi-factor authentication can help secure data against unauthorized access. Regular security audits can identify vulnerabilities and help prevent data breaches.
- A secure dataset is inherently more reliable because it guards against tampering and corruption.
Step 7: Apply statistical tests for reliability #
- Use statistical methods like Cronbach’s alpha, Test-Retest, or Inter-rater Reliability to quantitatively assess your data’s reliability. Statistical tests should be chosen based on the type of data and the research design.
- The results provide a measurable metric that can be tracked over time for improvements or deteriorations. These tests add a layer of empirical credibility to your data.
- Perform these tests periodically, especially after any major changes in data collection methods.
Step 8: Leverage automation for quality control #
- Automation can significantly enhance the consistency and accuracy of data.
- Automated scripts can clean and preprocess data, eliminating manual errors.
- Data integrity checks can be automated to flag discrepancies in real-time.
- Automation improves reliability by reducing the risk of human errors and biases.
- It also allows scalability, making the dataset reliable across large volumes of data.
Step 9: Continuous training and skill enhancement #
- The human element in data management cannot be ignored.
- Regular training programs can keep staff updated on best practices and new technologies.
- Training enhances awareness about the importance of data reliability and ways to maintain it.
- Skill enhancement leads to better data handling, ultimately improving reliability.
- A trained workforce is a vital asset in the quest for data reliability.
Step 10: Continuous monitoring #
- Implement real-time monitoring systems to track anomalies or inconsistencies in data. The monitoring system should automatically flag these issues for immediate investigation.
- Feedback from these monitoring systems should be integrated into the data governance policies. Immediate corrective actions based on the feedback can significantly enhance data validity and reliability.
Step 11: Establish feedback mechanisms #
- Feedback loops with end-users, data scientists, and other stakeholders can offer invaluable insights into data quality. Real-time feedback mechanisms can trigger immediate corrective actions.
- Periodic reviews with subject matter experts can identify domain-specific issues affecting reliability. Feedback helps in adapting and refining data processes over time.
- An agile approach to feedback and adaptation contributes to sustaining high levels of data reliability.
Improving data reliability is an ongoing endeavor that requires a multi-pronged approach. Besides, ensuring validity and reliability is an ongoing process, not a one-time effort. It involves a multidisciplinary approach, blending governance policies, technological tools, and statistical methods. This integrated approach will fortify the credibility and quality of your data.
Data reliability is not a fixed attribute but a dynamic quality that can be enhanced. Organizations seeking to improve their data-driven decision-making must prioritize efforts to bolster the reliability of their datasets. This improved reliability, in turn, empowers your organization to make more accurate, confident decisions.
Summing up #
Data may be the fuel powering organizational success today, but low-quality data stalls progress. Establishing rigorous protocols to ensure reliability is thus a business-critical priority. This requires a multidimensional approach spanning governance, collection, storage, auditing, automation, training, and stakeholder engagement.
Leadership must steer the adoption of best practices to safeguard their validity. Statistical testing and continuous monitoring enable ongoing enhancements. With reliable data as their compass, teams can navigate complexity with confidence, backed by credible insights.
Related reads #
- 11 Proven Strategies for Achieving Enterprise-Scale Data Reliability
- How to Choose a Data Reliability Engineer: 9 Skills to Look For!
- Data Quality Explained: Causes, Detection, and Fixes
- Data Quality Measures: Best Practices to Implement
- What Is a Data Catalog? & Why Do You Need One in 2023?
- 18 Data Validations That Will Help You Collect Accurate Data
- Benefits of Moving On-Premise to Cloud: Empowering Your Business for Success
Share this article