11 Proven Strategies for Achieving Enterprise-Scale Data Reliability
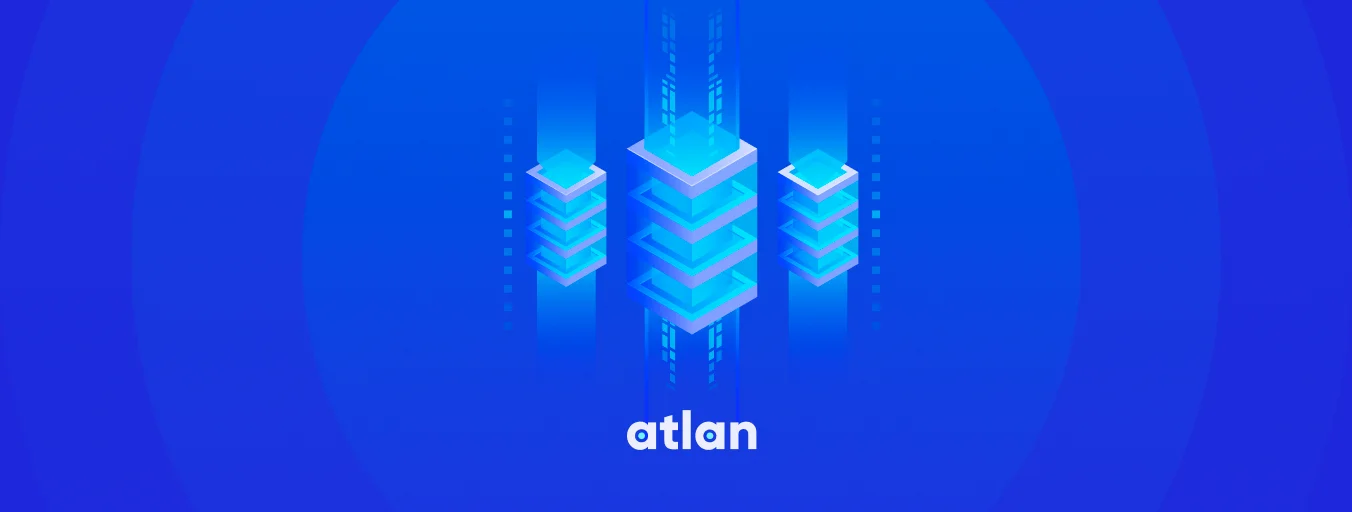
Share this article
Enterprise-scale data reliability is the consistent accuracy and availability of data in an organization, particularly one that operates at a large scale or across multiple geographies.
Reliable data isn’t just a nice-to-have; it’s a business imperative. In an enterprise context, data reliability ensures that operations run smoothly, decisions are informed, and compliance requirements are met, among other benefits.
In this article we will learn the 10 key strategies, to arm you with the knowledge you need to make your data as reliable as it is valuable.
Let us dive in!
Table of contents #
- Enterprise-scale data reliability: Why is it important for you?
- Ensuring enterprise-scale data reliability: 11 Strategies you can follow!
- Finally, what is the difference between data reliability and data quality?
- Enterprise scale data reliability: Books and resources
- Summary
- Related reads
Enterprise-scale data reliability: Why is it important for you? #
Enterprise-scale data reliability refers to the dependability and integrity of data across a large organization’s various systems, processes, and stakeholders. In simpler terms, it ensures that the data within the enterprise is accurate, consistent, and available when needed.
Enterprise-scale suggests that this isn’t just about a single database or application but encompasses a much larger scope. This could include multiple departments, geographies, data types, and data sources like CRMs, ERPs, databases, sensors, etc.
Here are a few reasons that make enterprise-scale data reliability so important for you:
1. Decision-making #
Accurate and timely data is at the heart of making informed decisions. Decision-makers, from the CEO to frontline managers, rely on data to assess performance, make forecasts, and plan future actions.
If the data is unreliable, decisions based on that data can be flawed, leading to financial losses, inefficient operations, or even legal issues. In an enterprise context, the consequences of such poor decisions can be magnified due to the scale and complexity of operations.
2. Compliance and regulatory needs #
Industries like healthcare, finance, and energy have stringent compliance requirements around data (e.g., HIPAA, GDPR, Sarbanes-Oxley Act). Non-compliance can result in hefty fines, legal actions, and reputational damage.
Reliable data helps in maintaining accurate records and traceability, which is essential for meeting these compliance and regulatory needs.
3. Customer trust #
Customer expectations have never been higher, particularly regarding how their data is handled. Mistakes like incorrect billing, unavailability of service due to data errors, or data breaches can quickly erode customer trust.
Maintaining data reliability helps in delivering consistent and accurate services that meet customer expectations, thereby nurturing trust and encouraging loyalty.
4. Operational efficiency #
Enterprise operations include multiple interlinked processes, from procurement to production to delivery. Unreliable data can create bottlenecks, delays, or inefficiencies.
For example, incorrect inventory data may result in stockouts or overstocking, both of which have cost implications. Reliable data ensures smooth operational flow.
5. Data integration #
Enterprises often operate multiple, disparate data systems (CRM, ERP, databases, etc.). Inconsistent or unreliable data can make it difficult to integrate these systems, reducing the ability to obtain a single, unified view of the business.
Data reliability ensures that integrations are meaningful, helping businesses to glean better insights.
6. Competitive advantage #
In today’s data-driven world, the quality of your data can be a significant differentiator. Enterprises with access to reliable data can gain insights more quickly and accurately than their competitors. This leads to better products, more efficient marketing, and generally smarter business decisions.
7. Scalability #
As an enterprise grows, its data needs grow exponentially. Unreliable data can severely hamper the ability to scale operations, as employees may need to manually check data for errors, new systems may not integrate well with old ones, or poor-quality data may lead to incorrect strategic decisions.
8. Data security #
Reliability is not just about data accuracy but also about data security. Data breaches can result in a loss of critical and sensitive information. A robust framework for data reliability includes measures to secure data against unauthorized access, cyber-attacks, and other security threats.
9. Innovation #
Innovation is heavily dependent on data. Whether it’s refining existing products or services, or creating new ones, reliable data is essential for R&D, market analysis, customer feedback, and more. Unreliable data can send innovation down the wrong path, wasting time and resources.
10. Cost savings #
Unreliable data comes with hidden costs. Resources have to be allocated to identify and rectify errors. There may be costs related to unsatisfied customers, operational inefficiencies, or fines for compliance lapses. Reliable data helps in avoiding these costs, offering tangible financial benefits.
11. Resource allocation #
An enterprise has various resources, including human resources, capital, and assets. Reliable data helps management to understand the efficiency and effectiveness of these resources. It enables organizations to optimize workforce deployment, investment allocation, and asset utilization, thus increasing overall productivity and profitability.
Each of these reasons emphasizes why enterprises cannot afford to overlook the reliability of their data. With the increasing role that data plays in strategic and operational decision-making, its reliability is more crucial than ever.
Ensuring enterprise-scale data reliability: 11 Strategies you can follow! #
Achieving enterprise-scale data reliability is a complex endeavor that requires a multi-faceted approach. Here are some critical strategies, along with detailed explanations for each:
- Data governance framework
- Data quality management
- Centralized data repository
- Disaster recovery and business continuity planning
- Secure access controls
- Data lineage and metadata management
- Real-time monitoring and alerts
- Compliance monitoring
- Data validation
- Employee training and awareness
Let us understand each of them in detail:
1. Data governance framework #
Instituting a data governance framework involves defining who owns the data, who is responsible for its quality, and what protocols should be followed for data management. This framework provides a foundational structure for data reliability by establishing rules and responsibilities.
A data governance committee, consisting of stakeholders from various departments, can oversee this framework to ensure adherence and periodic reviews.
2. Data quality management #
This involves regular monitoring and auditing of data to ensure it meets predefined quality standards (accuracy, completeness, timeliness, etc.). Data profiling and data cleansing techniques can be employed to check for anomalies and errors.
Regularly conducting data quality assessments helps in maintaining a high level of data reliability across the enterprise.
3. Centralized data repository #
Consolidating data into a centralized data warehouse or data lake makes it easier to manage and ensure its reliability. A centralized repository ensures that all business units access a “single source of truth,” thus eliminating discrepancies that can arise from decentralized data storage and management.
4. Disaster recovery and business continuity planning #
Data must not only be accurate but also available when needed. A robust disaster recovery plan ensures that data is regularly backed up and can be quickly restored in case of system failures, cyber-attacks, or other unforeseen events.
Business continuity planning goes hand-in-hand with this, ensuring that critical business functions can continue even when data systems encounter issues.
5. Secure access controls #
Part of data reliability is ensuring that data is secure and accessed only by authorized personnel. Implementing strict access controls and using technologies like encryption and multi-factor authentication can prevent unauthorized data access or modification, thus ensuring its integrity.
6. Data lineage and metadata management #
Understanding where the data comes from (data lineage) and what it represents (metadata) is crucial for reliability. Data lineage tools can trace the flow of data through various transformations, helping to identify any bottlenecks or errors in the pipeline.
Metadata management helps in understanding the data context, thereby aiding in its correct interpretation and use.
7. Real-time monitoring and alerts #
Real-time monitoring tools can instantly notify administrators about data issues such as inconsistencies, unauthorized access, or system failures. Immediate attention to these alerts ensures that the problem is corrected before it can significantly impact the data’s reliability.
8. Compliance monitoring #
Regular checks should be conducted to ensure that all data handling and storage procedures are compliant with relevant laws and regulations. Compliance monitoring tools can automate this process, flagging any potential issues for review, thus safeguarding against legal repercussions.
9. Data validation #
Before data enters the enterprise system, it should undergo validation checks to ensure it meets the pre-defined criteria. This can be accomplished through automated data validation workflows that screen data for inconsistencies, incompleteness, or anomalies, thereby ensuring only quality data enters the system.
10. Employee training and awareness #
Human error is often a significant contributor to data unreliability. Regular training programs aimed at making employees aware of the importance of data accuracy, security protocols, and best practices can go a long way in maintaining data reliability.
By adopting these strategies in a systematic and coordinated way, an enterprise can significantly enhance its data reliability, thus realizing all the benefits that reliable data brings in terms of operational efficiency, decision-making, compliance, and competitive advantage.
Finally, what is the difference between data reliability and data quality? #
While the terms “data reliability” and “data quality” are often used interchangeably, they have distinct meanings and implications. Here’s a tabular breakdown to differentiate the two:
Criteria | Data reliability | Data quality |
---|---|---|
Definition | Refers to the consistency and stability of data over time. | Refers to the overall condition of data based on factors like accuracy, completeness, and timeliness. |
Scope | Primarily concerned with the dependability of data across its lifecycle, including storage, retrieval, and update operations. | Covers a broad range of attributes such as accuracy, completeness, consistency, reliability, and timeliness. |
Objectives | To ensure data remains consistent and is available when needed. | To ensure data is fit for its intended uses in operations, decision-making, and planning. |
Measurement | Generally measured in terms of availability, fault tolerance, and backup recovery. | Measured using various attributes like accuracy, consistency, completeness, and relevancy. |
Importance | Vital for ensuring that data is stable and retrievable for decision-making and operational activities. | Crucial for making sure that the data can be trusted and is actually useful for analytics, reporting, and other functions. |
Concerns | Data availability, disaster recovery, fault tolerance. | Data accuracy, data integrity, data consistency, data completeness. |
Management Aspect | Managed through strategies like disaster recovery planning, backup procedures, and ensuring system uptime. | Managed through data cleaning, validation, transformation, and ongoing monitoring. |
Examples | Ensuring a database is always up and running; ensuring backups are consistently available. | Removing duplicates from a database; validating email addresses in a data set. |
Dependency | Can be viewed as a subset of data quality. Reliable data is often a pre-condition for high data quality. | Data quality is a broader concept that includes data reliability as one of its many dimensions. |
Enterprise scale data reliability: Books and resources #
Enterprise-scale data reliability is a critical concern for organizations that deal with large volumes of data, across different domains, technologies, and even geographical locations. There are a variety of books, online courses, articles, and other resources available to learn about this topic. Here’s a list broken down into different categories:
Books #
- Designing data-tntensive applications by Martin Kleppmann: This book provides an excellent foundational understanding of the architecture and technologies behind large-scale data systems.
- The data warehouse toolkit by Ralph Kimball and Margy Ross: This book is focused more on the data warehousing aspect but provides valuable insights into building reliable and scalable data systems.
- Streaming systems by Tyler Akidau, Slava Chernyak, and Reuven Lax: Focuses on data streaming architectures, which are a significant part of enterprise-scale data pipelines.
- Site reliability engineering: How Google Runs Large-Scale Systems by Niall Richard Murphy, Betsy Beyer, Chris Jones, and Jennifer Petoff: This book gives an introduction to the principles of reliability engineering, as practiced at Google.
- The Art of Scalability" by Martin L. Abbott and Michael T. Fisher: This is more of a generalist book focusing on how to scale systems, but it covers the data layer as well.
Summarizing it all together #
Achieving enterprise-scale data reliability is no small feat, but it’s an essential one in our increasingly data-dependent world. As we’ve explored, it requires a multidimensional approach—from robust data governance frameworks to employee training and awareness.
Each strategy we’ve discussed serves as a cog in the intricate machinery of enterprise data management. The 11 strategies outlined here are your toolkit for building a more reliable, efficient, and compliant data ecosystem.
In doing so, you’ll not only fortify your enterprise against operational hiccups and compliance risks but also position it for sustainable growth and competitiveness. Remember, in the age of information, data is your most valuable asset—only if you can rely on it. So, take the reins of your data destiny and steer your enterprise toward unerring reliability.
Enterprise scale data reliability: Related reads #
- Enterprise Data Governance — Basics, Strategy, Key Challenges, Benefits & Best Practices
- Enterprise Data Catalog: Definition, Importance, Architecture, Use Cases, Framework & Benefits
- What Is a Data Catalog? Do You Need One?
- Data catalog benefits: 5 key reasons why you need one
- Open Source Data Catalog Software: 5 Popular Tools to Consider in 2023
- Data Catalog Platform: The Key To Future-Proofing Your Data Stack
Share this article