Quantitative vs Qualitative Data: Differences & Benefits
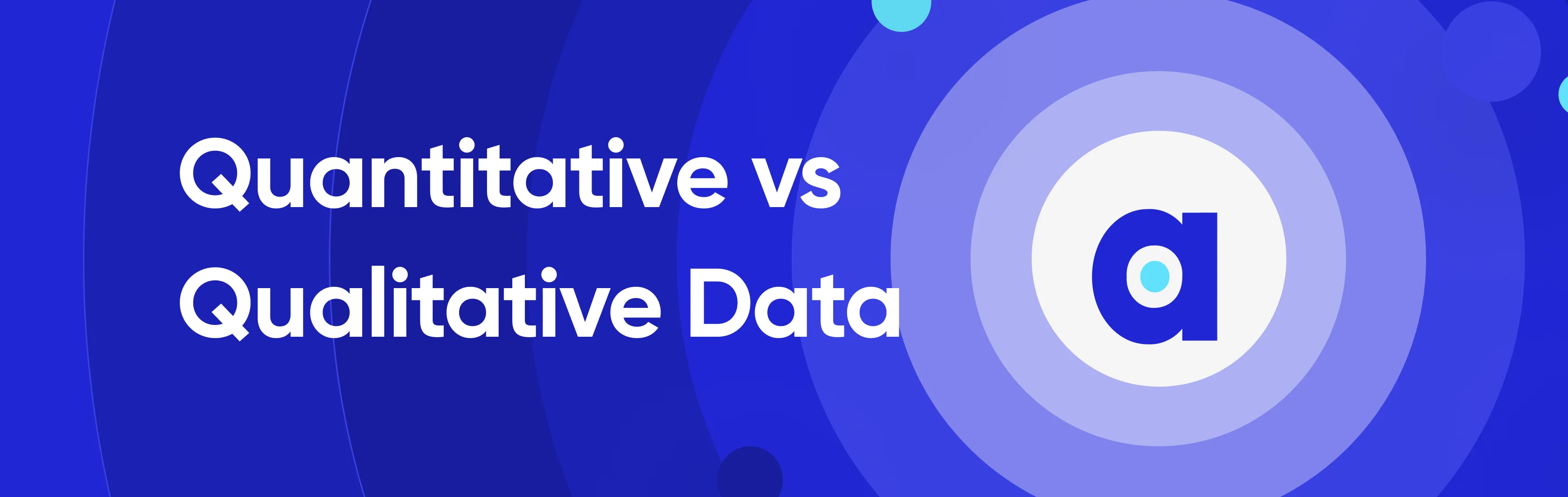
Share this article
Quantitative vs qualitative data #
Quantitative data provides measurable metrics and helps track performance, while qualitative data adds context and depth to the numbers. Both qualitative and quantitative data are valuable for businesses.
By using both types of data together, businesses can gain a more comprehensive understanding of their customers, make well-informed decisions, and develop effective strategies that consider both objective measurements and subjective experiences.
Modern data problems require modern solutions - Try Atlan, the data catalog of choice for forward-looking data teams! 👉 Book your demo today
In this blog, we will discuss the differences between qualitative vs quantitative data and their combined roles which can benefit your business.
Let’s dive in!
Table of contents #
- Quantitative vs qualitative data
- Understanding them better
- Difference between qualitative and quantitative data with examples
- How do businesses generally collect, manage, and utilize qualitative vs quantitative data?
- What system is required to handle both quantitative and qualitative data?
- Data team composition for effective data utilization
- How to ensure maximum utilization of both qualitative and quantitative data?
- What else should you know about the use of quantitative and qualitative data in business?
- Qualitative vs quantitative data - A tabular view
- Summary
- Related reads
Quantitative vs qualitative data: Understanding them better #
Let us first understand the basics of both quantitative vs qualitative data:
What is quantitative data? #
It is numerical, measurable, and can be used for statistical analysis. It answers the questions “how much,” “how many,” “how often,” or “to what extent.”
In the business context, this could include sales data, financial metrics, customer usage data, or employee performance data. It can be graphically represented and is typically subject to various forms of statistical analysis to detect trends, correlations, and other patterns.
For example, a company might use quantitative data to measure the growth rate of users, the conversion rate of a sales process, or the trend of monthly revenue.
What is qualitative data? #
Qualitative data, on the other hand, is non-numerical, interpretive, and exploratory. It provides insights into why certain behaviors occur and adds context to quantitative findings.
This kind of data can be derived from:
- Interviews
- Open-ended survey responses
- Customer reviews
- Observational notes
It provides a deeper understanding of the motivations, opinions, and drivers behind the numbers. For instance, a business might use qualitative data to understand customer preferences, perceptions of a brand, or reasons for product dissatisfaction.
The importance for a business executive lies in the combination of both these data types. Quantitative data can provide a broad, macro view of what is happening in the business, while qualitative data can help drill down to understand why it’s happening.
The numbers can tell you that sales are down, but it might take some qualitative investigation to understand that it’s because customers find your website difficult to navigate.
The integration of qualitative and quantitative data can lead to a more comprehensive view of business performance, better decision-making, and ultimately, more positive outcomes.
Difference between qualitative and quantitative data with examples #
Qualitative and quantitative data are two different types of information collected in research. They serve unique purposes and are often used in different types of research methodologies. Both types of data have strengths and limitations, and they provide different insights into the phenomena being studied.
Quantitative data #
- Quantitative data is numerical, structured, and statistical. It is used to quantify the problem by generating numerical data that can be transformed into statistics.
- This data type is usually collected through measurements, tests, structured questionnaires, and rating scales.
- The key aim is to determine the relationship between an independent variable and a dependent or outcome variable in a population.
What is an example of quantitative data? #
If a company is trying to determine the average number of hours its employees spend at work, it would collect quantitative data like the number of hours each employee logged at work during a particular period. This type of data can be easily counted, measured, and expressed using numbers. It allows researchers or analysts to make precise measurements and perform statistical analysis.
Qualitative data #
- On the other hand, qualitative data is non-numerical, unstructured, and detailed. This type of data is used to uncover trends in thoughts and opinions and dive deeper into the problem.
- It provides insights into the problem or helps to develop ideas or hypotheses for potential quantitative research.
- Qualitative data is typically collected through interviews, focus groups, observations, and open-ended questionnaires.
What is an example of qualitative data? #
If a company wants to understand its employees’ feelings and perceptions about the workplace environment, it may conduct interviews or focus groups. Employees might describe the environment as “stressful,” “supportive,” “competitive,” etc. These descriptions are examples of qualitative data because they provide context, depth, and detail about the workplace environment.
In both examples, the combination of quantitative and qualitative data provides a more comprehensive picture of the situation. By measuring the average number of hours worked (quantitative) and understanding employee perceptions (qualitative), the company gains a better understanding of its employees’ experiences.
It’s important to remember that neither type is inherently better or more valuable than the other; they simply provide different types of information and serve different purposes in the research process.
How do businesses generally collect, manage, and utilize qualitative vs quantitative data? #
Businesses have a multitude of methods to collect, manage, and utilize both qualitative and quantitative data. Let’s break it down for each type:
Here are some of the methods to collect, manage and utilize quantitative data:
- Collection
- Management
- Utilization
Now, let’s look into the methods to collect, manage and utilize quantitative data in detail.
Quantitative data: #
1. Collection #
Quantitative data is usually collected through instruments like:
- Surveys with close-ended questions
- Website analytics tools
- Financial systems
- Customer databases
- Point-of-sale systems
Companies can also purchase quantitative data from third-party sources, such as market research firms or public databases.
2. Management #
Quantitative data is typically structured and stored in databases or data warehouses. Given its numerical nature, it lends itself well to the organization in spreadsheets or specialized data visualization and analytics tools like Tableau, PowerBI, and Excel.
Effective management often involves data cleaning to ensure accuracy and consistency, as well as proper security measures to ensure data privacy and compliance with regulations.
3. Utilization #
Businesses often use quantitative data to measure performance against key performance indicators (KPIs), identify trends, and make forecasts. Data analysis and visualization tools can help to illustrate these insights in a way that’s easily understandable.
Advanced modeling techniques like the below-mentioned ones can be used to make predictions and inform strategic decisions:
- Data mining
- Machine learning
- Statistical.
Qualitative data #
Here are some of the methods to collect, manage and utilize qualitative data:
- Collection
- Management
- Utilization
Now, let’s look into the methods to collect, manage and utilize qualitative data in detail.
1. Collection #
Qualitative data is often collected through methods like:
- Interviews
- Focus groups
- Open-ended survey questions
- Customer feedback
- Social media monitoring
- Observational research
2. Management #
Unlike quantitative data, qualitative data is typically unstructured or semi-structured, making it more challenging to manage. It often needs to be categorized or coded in some way to allow for analysis.
- This might involve tagging comments or feedback with relevant themes or categories, or transcribing and annotating interviews or focus group recordings.
- Qualitative data analysis tools like NVivo can help with this process.
- Like quantitative data, qualitative data also needs to be stored securely and handled in a way that complies with privacy regulations.
3. Utilization #
Qualitative data is invaluable for gaining a deeper understanding of customer needs, motivations, and perceptions. It can be used to inform:
- Product development
- Improve customer experience
- Shape marketing messages
- Address specific issues or challenges identified through quantitative data.
Qualitative data often provide the context and narrative that helps to explain the ‘why’ behind the numbers.
A business that effectively integrates both quantitative and qualitative data in its decision-making processes is likely to have a well-rounded understanding of its operations, customers, and market, enabling it to make informed strategic decisions.
What system is required to handle both quantitative and qualitative data? #
Handling data at scale requires robust systems for data collection, storage, management, and analysis. Here are some systems and practices an organization should consider:
- Data collection systems
- Data storage systems
- Data management and governance systems
- Data analysis and visualization tools
- Data integration and ETL tools
- Advanced analytics and AI
- Data security and privacy tools
Let us understand these systems in a bit more detail:
1. Data collection systems #
For quantitative data, automated data collection systems such as the ones below are a necessity:
- Customer relationship management (CRM) systems like Salesforce or HubSpot can track sales, customer interactions, and other valuable metrics.
- Enterprise resource planning (ERP) systems manage and integrate important business functions, providing a wealth of operational and financial data.
- Digital analytics tools like Google Analytics, Mixpanel, or Adobe Analytics can provide data on website or app usage.
- IoT devices and sensors can collect data in real time on a variety of metrics, particularly for manufacturing or logistics businesses.
For qualitative data:
- Customer feedback tools like Uservoice or Medallia can gather user feedback and reviews.
- Social listening tools like Brandwatch or Hootsuite can track mentions and sentiments on social media.
- Focus group software or online survey tools like SurveyMonkey or Qualtrics can collect qualitative data on a large scale.
- Customer support systems like Zendesk can provide a wealth of qualitative data on customer queries and complaints.
2. Data storage systems #
A robust database or data warehouse is needed to securely store data at scale:
- SQL-based systems like MySQL or PostgreSQL are widely used for structured data.
- NoSQL databases like MongoDB can handle unstructured or semi-structured data.
- Data warehousing solutions like Google BigQuery, Amazon Redshift, or Snowflake are designed for storing and analyzing large volumes of data.
3. Data management and governance systems #
Data quality and governance are crucial when dealing with data at scale.
- Master data management (MDM) solutions ensure data consistency and accuracy across the organization.
- Data cataloging tools help manage data assets and maintain a metadata repository.
- Data governance platforms ensure data is handled in a way that meets legal and ethical requirements.
4. Data analysis and visualization tools #
Tools that can analyze and present data in an understandable format are key:
- For quantitative data, Business Intelligence (BI) tools like Tableau, PowerBI, Looker, or Qlik can analyze and visualize data.
- Statistical software like SPSS or R can be used for more advanced quantitative analysis.
- For qualitative data, text analysis tools like NVivo, Atlas.ti, or Leximancer can help categorize and understand text-based data.
5. Data integration and ETL tools #
To work with both qualitative and quantitative data, tools are needed to integrate, transform, and load data from a variety of sources.
- ETL (Extract, Transform, Load) tools like Talend, Informatica, or Google Cloud Dataflow can automate the process of consolidating data from multiple sources and preparing it for analysis.
6. Advanced analytics and AI #
To derive deeper insights from large volumes of data, AI and machine learning tools can be invaluable.
- Tools like TensorFlow, PyTorch, or Azure Machine Learning can be used to develop and implement machine learning models.
- Natural language processing (NLP) tools can help analyze and understand qualitative data at scale.
7. Data security and privacy tools #
When handling data at scale, privacy and security are paramount. Therefore,
- Encryption tools can protect data both at rest and in transit.
- Access management systems can control who has access to different types of data.
- Privacy management tools can ensure compliance with regulations like GDPR or CCPA.
A comprehensive system needs to consider all these areas. The precise mix will depend on the specific needs and context of the business, including the type of data they work with, their industry, and their regulatory environment. Integration of these systems is key - data needs to flow seamlessly between.
Data team composition for effective data utilization #
To effectively collect, manage, and utilize both qualitative and quantitative data, an organization should consider assembling a diverse and modern data team with a range of skill sets.
Here’s a possible composition for a data team:
- Data engineers
- Data analysts
- Data scientists
- Business intelligence (BI) analysts/developers
- Database administrators (DBAs)
- Data quality managers
- Data governance officers
- Data strategists/Data product managers
- User researchers
- Data visualization experts
Let us understand each of their roles in brief:
1. Data engineers #
They are responsible for designing, building, and managing the data infrastructure. This includes the data collection systems, databases, data warehouses, and ETL processes. Data engineers ensure that data is available, reliable, and accessible for analysis.
2. Data analysts #
Data analysts work with both qualitative and quantitative data to provide insights that inform business decisions. They need strong statistical skills to work with quantitative data, as well as strong interpretive skills to understand and analyze qualitative data.
They typically work closely with business teams to understand their data needs and provide relevant insights.
3. Data scientists #
For more complex data tasks, data scientists apply machine learning models and other advanced techniques to extract insights from data. They might work on predictive modeling, customer segmentation, natural language processing for qualitative data, or other complex analyses.
4. Business intelligence (BI) analysts/developers #
They focus on designing and creating data reports and dashboards using BI tools. They should understand the business context well to present the most relevant and useful information.
5. Database administrators (DBAs) #
DBAs ensure that the databases run smoothly. They are responsible for data security, backup and recovery, performance tuning, and other maintenance tasks.
6. Data quality managers #
They ensure that the data is accurate, consistent, and reliable. This involves:
- Setting up data validation rules
- Resolving data quality issues
- Maintaining documentation on data standards and procedures.
7. Data governance officers #
They ensure compliance with relevant data regulations and best practices. This is especially important for industries like healthcare or finance that have specific data handling requirements.
8. Data strategists/Data product managers #
They work at the intersection of data and business strategy, overseeing the development and implementation of data-driven projects and ensuring alignment with business objectives.
9. User researchers #
They gather qualitative data through methods like user interviews, focus groups, and usability testing. They interpret this data to provide insights into user needs and behaviors.
10. Data visualization experts #
They specialize in representing complex data in an easily understandable and visually appealing format. This role is crucial for communicating data insights to non-technical stakeholders.
In small organizations, one person may wear multiple hats. As an organization grows, it might also add roles like a Chief Data Officer (CDO) to oversee the data strategy at an executive level, or specialized roles like text analysts or AI specialists.
Effective collaboration is key in such a team. Everyone should have a clear understanding of the data lifecycle, from collection through to decision-making, and regular communication should be encouraged to ensure that data insights are effectively integrated into business decisions.
How to ensure maximum utilization of both qualitative and quantitative data? #
Achieving maximum utilization of both qualitative and quantitative data involves careful planning, communication, and the creation of a data-driven culture within the organization. Here are some strategies to ensure that all people, processes, and tools are in place:
- Develop a clear data strategy
- Invest in the right tools and infrastructure
- Build a skilled data team
- Foster a data-driven culture
- Establish clear processes and governance
- Prioritize data security and privacy
- Encourage collaboration and communication
- Continually evaluate and improve
- Promote transparency
Now, look into the strategies to ensure maximum utilization of both qualitative and quantitative data in detail.
1. Develop a clear data strategy #
Your data strategy should align with your overall business strategy. It should outline:
- The objectives for your data
- The types of data that will be collected and used
- The tools and infrastructure needed
- How data will be managed and governed
2. Invest in the right tools and infrastructure #
As discussed previously, it’s crucial to have the right systems in place for data collection, storage, analysis, and management.
You should invest in tools that fit your needs and have the capacity to handle your data volume and complexity. These tools should be interoperable to allow for seamless data integration.
3. Build a skilled data team #
You need a diverse team with skills in data engineering, data analysis, data science, data governance, and business intelligence. Investing in training and development can help ensure your team has the latest skills.
It may also be beneficial to hire individuals with business domain expertise, who can understand and apply data in the context of your business.
4. Foster a data-driven culture #
For data to be fully utilized, it needs to be integrated into decision-making processes across the organization.
This requires fostering a culture that values data, where all employees understand the importance of data and how it can help them in their roles. Providing training and resources can help build this culture.
5. Establish clear processes and governance #
Clear processes should be established for data collection, management, and use. This includes data quality processes to ensure data is accurate and reliable, as well as data governance to ensure compliance with data regulations.
6. Prioritize data security and privacy #
Ensuring the security and privacy of your data is crucial. This involves implementing robust security measures and complying with data privacy regulations.
7. Encourage collaboration and communication #
Encourage regular communication and collaboration between your data team and other departments. This can help ensure that data insights are relevant and effectively applied to business decisions.
8. Continually evaluate and improve #
The data landscape is continually evolving, so it’s important to regularly evaluate your data practices and make improvements where necessary.
This could involve adopting new tools or techniques, updating your data strategy, or providing additional training for your team.
9. Promote transparency #
Transparency about data practices and insights can foster trust and buy-in from all stakeholders. This might involve sharing regular reports or dashboards, explaining how data is used in decision-making, or involving employees in data projects.
By implementing these strategies, you can ensure that all people, processes, and tools are in place for maximum utilization of both qualitative and quantitative data.
What else should you know about the use of quantitative and qualitative data in business? #
The use of both qualitative and quantitative data is at the core of informed decision-making in businesses.
Here are a few more points that might be useful:
- Understanding the limitations of each type
- Balancing the two types
- Incorporating data into decision-making
- Ethical considerations
- Staying current with technologies and trends
- The importance of experimentation
- Predictive and prescriptive analytics
Let’s explore the use of both qualitative and quantitative data in detail.
1. Understanding the limitations of each type #
Every type of data comes with its own set of limitations.
- For instance, quantitative data might give you hard numbers, but it won’t necessarily tell you the reasons or motivations behind those numbers.
- Conversely, qualitative data provides rich detail and context but may not be representative of the larger population or segment due to typically smaller sample sizes.
Understanding these limitations helps businesses to leverage each type effectively and interpret findings correctly.
2. Balancing the two types #
It’s essential to maintain a balance between quantitative and qualitative data. Relying too much on one can skew the insights and lead to poor decisions. The two types should complement each other and provide a more holistic view of the situation.
3. Incorporating data into decision-making #
Using data is not just about collecting and analyzing it. It’s also about incorporating the insights into decision-making processes. This involves communicating findings effectively, ensuring they’re understood by decision-makers, and fostering a data-driven culture within the organization.
4. Ethical considerations #
Businesses must handle data ethically, respecting privacy and confidentiality. This includes:
- Obtaining data through appropriate and legal means
- Informing participants (if human subjects are involved) about how their information will be used
- Ensuring that data is stored securely and used responsibly.
5. Staying current with technologies and trends #
The field of data analysis and business intelligence is continually evolving, with new tools and methodologies regularly emerging. Staying current with these developments can help businesses gain a competitive edge and maximize their data utilization.
6. The importance of experimentation #
Data can provide valuable insights, but sometimes, the best way to learn is by experimenting. Businesses can use their data to identify potential improvements or innovations, test these out on a small scale, and then use the resulting data to evaluate their effectiveness.
7. Predictive and prescriptive analytics #
Beyond understanding what has happened (descriptive analytics) or why it happened (diagnostic analytics), businesses are increasingly using data to predict what will happen (predictive analytics) and what actions should be taken (prescriptive analytics). This involves more complex data modeling and often uses machine learning techniques.
Remember that the ultimate goal is to use qualitative and quantitative data to provide valuable business insights that lead to informed decision-making and improved business outcomes.
Qualitative vs quantitative data - A tabular view #
Below is a comparison table highlighting the main differences between qualitative and quantitative data:
Qualitative data | Quantitative data | |
---|---|---|
Nature | Non-numerical, descriptive | Numerical, measurable |
Data type | Text, images, audio, video | Numbers, statistics |
Collection methods | Interviews, focus groups, observations, case studies | Surveys, experiments, counting, databases |
Analysis | Interpretive, thematic analysis | Statistical, mathematical calculations |
Examples | Customer reviews, interview transcripts | Sales figures, website traffic, customer demographics |
Strengths | Provides depth and context, explore 'why' | Provides breadth, measurable, can generalize |
Limitations | Not easily generalizable, time-consuming | Lacks context, doesn't explain 'why' |
Use aases in business | Understanding customer sentiments, product feedback | Measuring performance, forecasting, trend identification |
Data management | Coding, theme identification | Database storage, data cleaning |
Represented graphically | Word clouds, thematic maps | Bar charts, line graphs, pie charts |
Remember, qualitative and quantitative data offer different insights and are often most powerful when used together to provide a well-rounded understanding of a business situation.
Summarizing it all together #
Qualitative data is non-numerical and descriptive, providing depth and context. It helps understand the ‘why’ behind occurrences. Quantitative data, on the other hand, is numerical and measurable, providing breadth and allowing for generalization. It shows ‘what’ is happening but doesn’t explain why.
In summary, qualitative and quantitative data, when collected, managed, and utilized appropriately, can provide valuable insights that can guide effective decision-making and drive positive business outcomes. It is crucial to have the right systems, teams, and culture in place to make the most of both types of data.
Quantitative vs qualitative data: Related reads #
- What Is a Data Warehouse: Concept, Architecture & Example
- What is DataOps? — DataOps Framework & 9 Key DataOps Principles
- Data Quality Measures: Best Practices to Implement
- 18 Data Validations That Will Help You Collect Accurate Data
- Benefits of Moving On-Premise to Cloud: Empowering Your Business for Success
- The Benefits of GDPR Compliance and Data Governance: Protecting Your Data and Your Business
Share this article