Technical Metadata 101: Everything You Need To Know
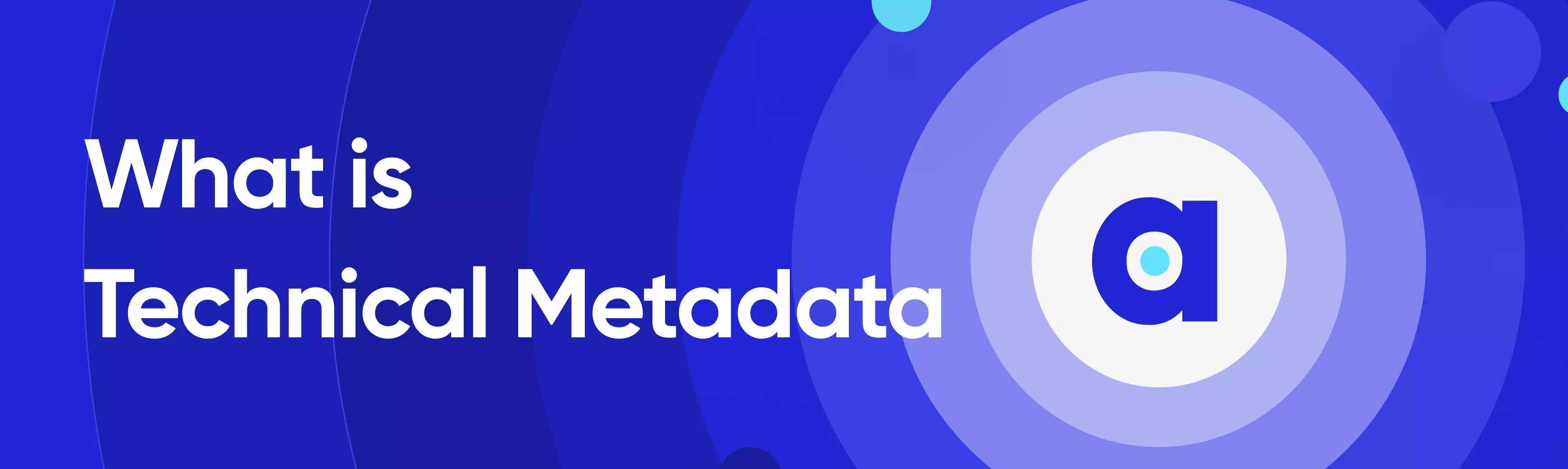
Share this article
Technical metadata refers to the information that describes the technical aspects of data, such as data structure, storage, format, and data processing.
It plays a vital role in managing, understanding, and effectively utilizing data within an organization.
In this blog we’ll understand how to:
- Boost your data management with technical metadata
- Collect and utilize technical metadata effectively by implementing strong systems and processes
- Explore hypothetical scenarios and use cases for metadata-driven automation
Let’s dive in.
Table of contents #
- 7 Ways Leveraging Technical Metadata Boosts Your Data Management
- Building a strong foundation: Systems and processes for technical metadata collection and utilization
- Applying metadata-driven automation: Hypothetical scenarios and use cases
- Rounding it all up
- Technical metadata: Related reads
7 Ways Leveraging Technical Metadata Boosts Your Data Management #
By leveraging technical metadata, your team can drive several crucial outcomes:
1. Data discovery and understanding #
Technical metadata enables data users to quickly discover and understand the data they are working with. It provides information about data formats, file types, data sources, and storage locations.
This helps users identify the right datasets for their needs and reduces the time spent on manual data exploration.
2. Data lineage and traceability #
Technical metadata helps track the data’s journey from its source to its current form, showing how it has been transformed, processed, and combined with other data sources along the way. This is crucial for understanding data quality, ensuring compliance, and identifying potential data issues.
3. Data quality and consistency #
By providing detailed information about data structures, technical metadata enables your team to identify inconsistencies, errors, or anomalies in the data. This helps maintain a high level of data quality and ensures that decision-makers can trust the data they are using.
4. Data cataloging and organization #
Technical metadata allows you to create a well-organized data catalog, making it easier for users to find and access the data they need. It helps classify data based on various attributes, such as file type, format, and usage, making it more accessible to users across the organization.
5. Data integration and transformation #
Understanding the technical aspects of your data is critical when integrating data from multiple sources or transforming it for specific use cases. Technical metadata ensures that data teams have the necessary information to perform these tasks efficiently and accurately.
6. Data governance and compliance #
Proper management of technical metadata supports data governance initiatives by providing transparency into data lineage, quality, and usage. This allows organizations to demonstrate compliance with regulations and maintain control over their data assets.
7. Reduced dependency on tribal knowledge #
With a clear understanding of technical metadata, your team can move away from relying on tribal knowledge and undocumented processes. This improves efficiency, reduces the risk of errors, and ensures that the entire organization can access and utilize data effectively.
By leveraging technical metadata, your team will be better equipped to manage and utilize data, driving informed decision-making and better business outcomes.
Building a strong foundation: Systems and processes for technical metadata collection and utilization #
To collect, automate, and utilize technical metadata effectively for driving mission-critical outcomes, you need to have several systems and processes in place:
1. Metadata management solution #
Implement a robust metadata management solution that can automatically discover, catalog, and store technical metadata from various data sources. This solution should integrate with your existing data infrastructure, such as databases, data warehouses, and data lakes, and offer capabilities like data lineage tracking, automated metadata extraction, and versioning.
2. Data catalog #
Create a centralized data catalog that organizes technical metadata in a user-friendly manner, making it easily accessible and searchable for data users. This catalog should support data classification, tagging, and indexing, allowing users to quickly find relevant datasets and understand their structure, format, and origin.
3. Data integration and ETL processes #
Ensure that your data integration and ETL (Extract, Transform, Load) processes are designed to capture and store technical metadata. This includes information about data sources, data transformations, and data quality checks, which should be stored alongside the processed data for easy access and analysis.
4. Data governance framework #
Establish a data governance framework that defines policies, procedures, and roles for managing technical metadata. This framework should outline how metadata is collected, updated, and maintained, as well as the responsibilities of different team members in ensuring metadata accuracy and completeness.
5. Data lineage and traceability tools #
Utilize tools that can automatically generate data lineage and traceability information, giving you insights into the journey of your data from source to destination. These tools should be able to visualize data flows, allowing users to understand the dependencies between datasets and track data transformations.
6. Metadata-driven automation #
Leverage metadata-driven automation to streamline data management tasks, such as data ingestion, validation, transformation, and integration. By using technical metadata to inform these processes, you can reduce manual intervention, increase efficiency, and minimize errors.
7. Training and awareness #
Provide training and resources to your data team, ensuring they understand the importance of technical metadata and how to leverage it effectively. This may include workshops, documentation, and ongoing support to help users navigate and utilize the metadata management solution and data catalog.
8. Monitoring and auditing #
Regularly monitor and audit your metadata management processes to ensure that technical metadata is up-to-date, accurate, and complete. This may involve setting up automated alerts for discrepancies or anomalies, as well as periodic manual reviews to validate metadata quality.
By implementing these systems and processes, your organization will be better equipped to collect, automate, and utilize technical metadata, driving mission-critical outcomes and improving overall data management.
Applying metadata-driven automation: Hypothetical scenarios and use cases #
Metadata-driven automation refers to using metadata to inform and control various data management processes, reducing manual intervention and increasing efficiency. By leveraging metadata, organizations can streamline data ingestion, validation, transformation, integration, and other data-related tasks.
Let us go over a few hypothetical examples of workflows and use cases for metadata-driven automation:
1. Data ingestion and validation #
A financial institution receives transaction data from various sources, such as ATMs, mobile apps, and online banking portals. Metadata-driven automation can be used to identify the format, schema, and source of each incoming dataset and automatically apply appropriate validation rules based on the metadata.
This ensures that the ingested data adheres to predefined quality standards without requiring manual intervention.
2. Data transformation and mapping #
An e-commerce company collects data from multiple vendors in different formats and structures. Metadata-driven automation can be used to dynamically generate transformation rules and mappings based on the technical metadata of each source dataset (e.g., column names, data types, etc.).
This enables the company to automatically transform and harmonize the incoming data into a standardized format, making it easier to analyze and integrate with other datasets.
3. Data lineage and impact analysis #
A healthcare organization needs to track data lineage for regulatory compliance and ensure the accuracy of its reports. By leveraging metadata-driven automation, the organization can automatically generate and maintain data lineage information, such as the origin, transformation steps, and dependencies of each dataset.
This enables users to quickly identify the impact of data changes on downstream processes and reports, allowing them to make informed decisions about data updates and modifications.
4. Data catalog maintenance #
A media company maintains a data catalog that serves as a single source of truth for all its data assets. Metadata-driven automation can be used to automatically update the catalog with new or updated datasets, as well as track changes to data schemas, formats, and structures.
This keeps the data catalog current and accurate, allowing users to find and access the most up-to-date information quickly.
5. Dataset versioning and archiving #
A research organization generates multiple versions of datasets as they update and refine their data over time. Metadata-driven automation can be used to automatically track changes to datasets, create new versions when updates are made, and archive older versions as needed.
This ensures that users can easily access the most recent version of a dataset while maintaining a historical record of changes.
These are just a few examples of how metadata-driven automation can be used to streamline data management processes and improve efficiency. By leveraging technical metadata, organizations can reduce manual intervention, minimize errors, and ensure that their data is consistently managed and maintained across the enterprise.
Rounding it all up #
In this blog, we learnt the importance of technical metadata in managing, understanding, and effectively utilizing data within an organization.
Technical metadata refers to the information that describes the technical aspects of data, such as data structure, storage, format, and data processing. There are seven ways that leveraging technical metadata boosts data management, such as data discovery, understanding, lineage, and quality.
To collect, automate, and utilize technical metadata effectively, we need to implement various systems and processes, including metadata management solutions, data catalogs, data integration, and ETL processes.
By understanding and leveraging technical metadata, organizations can better manage and utilize their data, driving informed decision-making and improved business outcomes.
Technical metadata: Related reads #
- Active Metadata: 101 Guide From Pioneers Of The Concept
- What is Metadata? - Examples, Benefits, and Use Cases
- What Is a Metadata Catalog? — Basics & Use Cases
- What is Metadata Management and Why is it So Important?
Share this article