AI Data Steward: How Can It Empower You to Change Gears from Tactical to Strategic
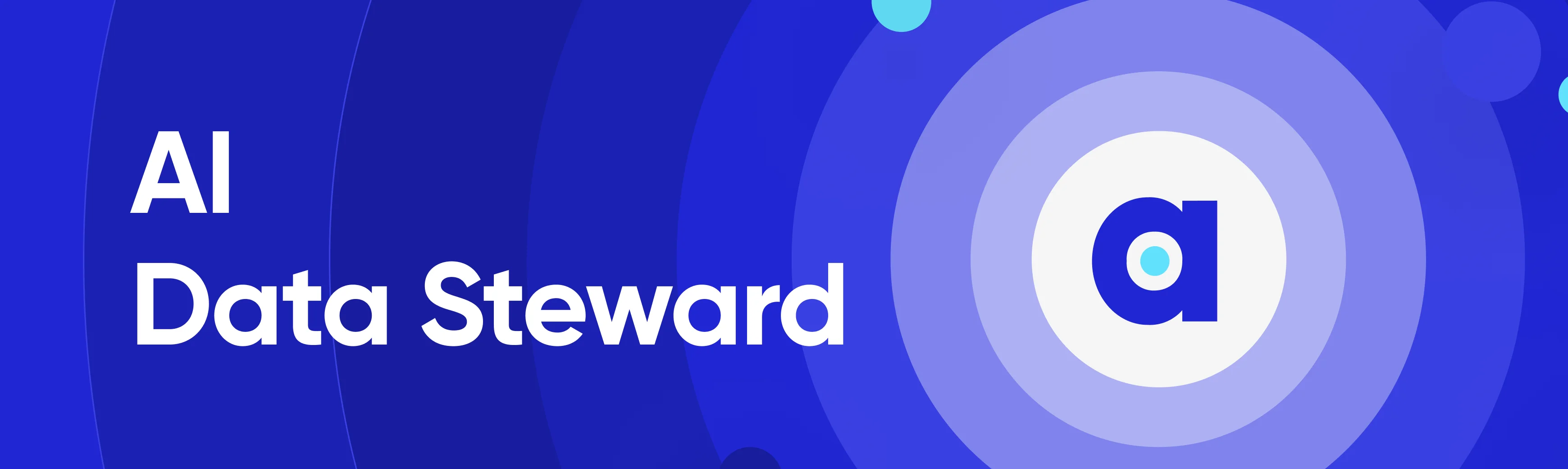
Share this article
An AI data steward would supercharge data governance, quality, and accountability with automation and generative AI.
Data stewardship is all about liaising between a company’s engineering and business sides to ensure data quality, integrity, security, and governance.
In this article, we’ll explore how AI can unburden the workload of a data steward with AI-powered metadata enrichment, automated classification, tagging, compliance, and more.
Table of contents
- What is an AI data steward?
- How can AI empower data stewards to get more done with minimal effort?
- What’s next?
- AI Data Steward: Related reads
What is an AI data steward?
An AI data steward is a copilot for data stewards that leverages automation and generative AI to scale data asset documentation, classification, quality control, and compliance.
According to a Forrester report on the role profile of a data steward:
“Data stewards ensure that data is usable, trusted, secure, and compliant with data policies and procedures across the organization. They also act as a liaison between the technology organization and business units to identify new policies, adapt existing policies to new use cases, and oversee changes that affect existing data policies.”
To see how AI can support data stewardship, let’s recap the role of a data steward. A data steward acts as a bridge between the engineering and business sides of your organization to:
- Create and enforce security and access control policies using data governance tools
- Ensure that the data is in good shape using data quality and profiling tools
Read more → Data Stewardship 101
With AI-powered technologies, data stewards can:
- Automate metadata extraction
- Analyze large volumes of real-time data to identify patterns, detect anomalies, ensure data quality, and more
- Operationalize data discovery, access control, and compliance at scale
How can AI empower data stewards to get more done with minimal effort?
Two elements that can boost the efficiency and productivity of data stewards are automation and generative AI.
So, rather than focusing on tactical, day-to-day tasks, a data steward would transition to more meaningful, strategic activities.
For instance, instead of classifying and tagging data assets themselves, data stewards would rely on AI to spot patterns and group similar data assets. While AI does the legwork, the data steward oversees by providing guidance and making critical decisions.
AI can support data stewards with several of their core responsibilities, such as:
- Metadata and documentation enrichment
- Classification and tagging for better data discovery and consistency
- Data quality control
- Certification-readiness and compliance
Let’s see how.
Metadata and documentation enrichment for better context and understanding of data
Building context from a small amount of data is generative AI’s core capability. If the context-building exercise is helped with the right prompts, it can result in you accomplishing more with less time and effort.
For instance, by understanding asset metadata, AI can generate descriptions, summaries, and READMEs. A data steward would then review this content, edit it (if required), and then publish.
AI can also help create structured metadata that describes the data asset’s content, context, and characteristics.
Moreover, using query patterns, comments, and other scattered bits of information, AI can feed information back to the metadata repository to enrich it. This saves time and effort spent in documentation, which data stewards can use to focus on more strategic tasks.
AI-driven classification and tagging for better data discovery and consistency
Effective data classification and tagging are crucial for ensuring consistency and standardization in how data is labeled and described. It also helps with data search and discovery, governance, and compliance with regulatory requirements.
That’s why classification and tagging are core responsibilities of a data steward.
Without generative AI, you can parse through data to classify and tag it, but it will be based on rules based on simple filters and regular expressions.
Generative AI can look for patterns and identify various types of data for tagging and classification.
So, data stewards can set up rule-based automation to analyze data assets, automatically classify and tag them, and even auto-suggest data asset owners at scale.
For example, imagine that you have to transfer ownership of several data assets. Instead of manually updating the ownership of each and every asset, you could create a playbook to automate this process and update the metadata of your assets at scale.
This improves consistency and accuracy in data labeling. Moreover, data that’s been well-organized help data practitioners better search, discover, and understand the available data assets.
Automated data quality control for better trust in data
Another key responsibility of a data steward is to control the quality of data flowing into your ecosystem.
For example, you can use AI to automatically detect and correct errors, remove duplicate records, fill in missing values, spot outliers, and more.
Generative AI can also help create automated tests without much engineering effort or tackling orphan records, backfills, and so on.
You can train AI to do all the legwork, detecting deviations or anomalies and triggering alerts whenever data quality thresholds are breached.
Automated certification-readiness and compliance for better governance
A sufficiently advanced AI will be able to automatically check for all the various rules and regulations a business must comply with to get a certification like GDPR, CCPA, HIPAA, etc.
For instance, let’s say you’re using an AI data catalog capable of identifying and compiling related assets under the same tag. AI can also ensure that the catalog propagates the right data encryption, masking, and anonymization policies via lineage mapping automatically.
This helps data stewards scale their efforts in data privacy and integrity, and avoid non-compliance with regulations.
AI can also do the heavy lifting by analyzing data usage patterns, access logs, and data handling practices to spot potential compliance issues or risks and recommend fixes.
AI can also give you a certification-readiness score by assessing various data quality factors, such as data accuracy, completeness, consistency, timeliness, and more. After the assessment, AI can identify actions for you to take or approve.
Read more → How AI-powered data catalogs can save time and increase productivity
What’s next?
AI-led data cataloging and governance will be a game changer for roles like data stewards and data managers.
Automating their daily workflows on metadata enrichment, documentation, quality control, and reporting and offering intelligent recommendations can increase productivity and efficiency.
Meanwhile, generative AI can provide valuable insights into data quality, usage patterns, and compliance measures, helping data stewards unearth unknown issues and find opportunities to improve.
So, data stewards can focus on developing data management strategies, ethical data practices, and more, while their AI copilot does all the legwork.
The possibilities and opportunities that AI data stewardship presents are endless, and we’re excited to see how the use cases evolve.
Share this article