Boost Data Analyst Productivity With These Hacks in 2024
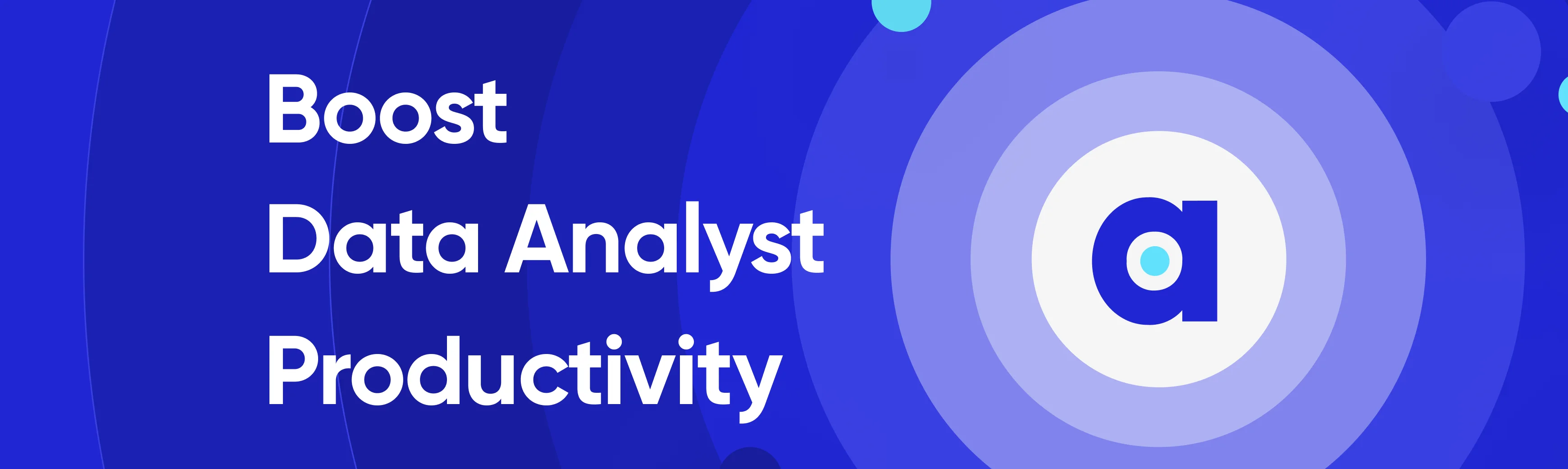
Share this article
As a data analyst, how can you boost your productivity?
Well, the confluence of Big Data and logistics presents an unprecedented opportunity to optimize business processes and make informed strategic decisions. As such, the role of a data analyst in a logistics company becomes crucial. They are tasked with extracting, cleaning, and interpreting vast amounts of data.
However, data analysts often find themselves battling against an endless barrage of data-related issues. From leakages and system malfunctions to general data retrieval and cleansing, the sheer volume of tasks can severely hamper their productivity. The end result is a reactive rather than a proactive use of your data resources.
If the potential of your data analysts is to be fully harnessed, it’s essential to shift the narrative from merely fixing problems to utilizing data for insightful analysis and business optimization. Let’s discuss some strategies to boost data analyst productivity in a logistics environment.
Table of contents
- Stellar strategies to boost your productivity as a data analyst
- Personal productivity hacks for data analysts
- Essential qualities to be a high-performing data analyst
- Related reads on data analyst productivity
7 Stellar strategies to boost your productivity as a data analyst
For your data analysts, you know that every minute counts when it comes to extracting valuable insights from complex datasets. Here’s how you can catalyze your team’s productivity and efficiency to new heights with the following strategies.
- Streamlining data governance
- Leveraging data management tools
- Emphasizing on training and skill development
- Encouraging collaboration and cross-functional interaction
- Fostering a data-driven culture
- Building a data catalog
- Setting clear objectives and KPIs
Let’s understand them in detail.
1. Streamlining data governance
Your data is only as reliable as the protocols governing it. Implement a robust data governance policy to ensure data quality, privacy, and compliance across all territories. Clear guidelines on data ownership, accessibility, and quality control will reduce the time spent handling data inconsistencies and disputes.
2. Leveraging data management tools
The use of advanced data management tools can significantly reduce time spent on mundane tasks like data cleaning and retrieval. Machine learning algorithms can automate these processes and offer analysts more time to focus on data interpretation and strategic tasks.
Implement tools like Alteryx, Trifacta, or Talend, that help in data preparation and data quality management.
3. Emphasizing on training and skill development
Continuous training and upskilling should be a core aspect of your data team’s growth strategy. Equip your analysts with skills to leverage advanced data manipulation and data visualization tools such as Tableau, Power BI, Python, or R. This will not only make them more productive but also provide them with a broader range of analytical techniques.
4. Encouraging collaboration and cross-functional interaction
Analysts working in silos can lead to duplications and inefficient use of resources. Promote a culture of collaboration where analysts, data engineers, and business teams work together to share insights and develop best practices. This can be facilitated through regular meetings, collaboration platforms, and cross-functional projects.
5. Fostering a data-driven culture
To reduce the load on your data team, instigate a data-driven culture throughout your organization. Train non-technical staff on the basics of data retrieval and interpretation so they can handle simple data tasks independently. This also empowers them to make informed decisions without always relying on your data team.
6. Building a data catalog
A well-organized data catalog can significantly speed up data discovery and accessibility. It provides a centralized repository with metadata information about all data assets, which makes it easier for analysts to find the data they need and understand its context.
7. Setting clear objectives and KPIs
Ensure your analysts are working toward clearly defined goals and KPIs. This will give them a better understanding of what they need to achieve and how their work contributes to the overall business objectives.
These strategies are designed to transform your data analysts from firefighters to strategic assets, allowing them to drive business value through insightful data analysis. However, successful implementation of these strategies requires strong leadership and commitment from the top. It’s vital for your managers, CIOs, and Chief Data Officers to champion these changes and lead by example.
Personal productivity hacks for data analysts in a logistics setting
In the realm of data analytics, particularly in complex industries like logistics, the challenge is twofold. On the one hand, there is the need to glean insights from vast data sets to facilitate strategic decision-making.
On the other hand, there’s the practical aspect of dealing with data—organizing it, cleaning it, and preparing it for analysis. This can often involve repetitive, time-consuming tasks that can hinder your productivity and limit the time you have for more valuable analytical work.
The good news is that there are numerous strategies that can help streamline your workflow, making you more efficient and productive as a data analyst. Here are some tactics you can apply:
- Refining workflow
- Saving and reusing code snippets
- Automation tools and ETL processes
- Mastery of tools Version control
- Maintaining a data dictionary
- Project management tools
- Continuous learning and upskilling
Let’s understand how to apply these tactics effectively.
1. Refining workflow
As a data analyst, having a well-structured workflow is crucial to your productivity. This means breaking down your tasks into manageable parts and tackling them systematically.
For example, you might start with data collection, followed by cleaning and preprocessing, then exploratory data analysis, modeling, and finally, interpretation and presentation of results.
Having this structure in place can help you stay organized and focused, reducing wasted time from switching between unrelated tasks.
2. Saving and reusing code snippets
Coding is a significant part of a data analyst’s role, and you often find yourself writing similar lines of code across different projects. Saving frequently used code snippets (for example, data cleaning scripts or commonly used SQL queries) in a dedicated repository can be a game-changer. Reusing these snippets not only saves you coding time but also reduces the chance of errors that may arise when coding from scratch.
3. Automation tools and ETL processes
Consider utilizing automation tools for repetitive tasks. For instance, if you find yourself extracting data from databases or APIs frequently, you could use an ETL tool or write a script to automate this process. Python, with libraries like pandas, offers a robust environment for creating such automation scripts.
4. Mastery of tools
The more proficient you are with your data analysis tools, the quicker you can get things done. Be it SQL for database queries, Python or R for data cleaning and analysis, or Tableau for data visualization, mastering these tools can significantly enhance your productivity. Attend webinars, take online courses, read books, or simply practice on new datasets to hone your skills.
5. Version control
Using version control systems like Git can make managing your codebase much easier, especially if you are collaborating with others. It keeps track of all changes, lets you revert to previous versions of your code, and helps resolve conflicts when merging code from different sources.
6. Maintaining a data dictionary
A data dictionary is a centralized source of information about your data, containing details like what each column represents, the type of data it contains, possible values, and where the data comes from. This can be incredibly helpful when dealing with large, complex datasets, allowing you to quickly understand your data without having to dive into the code.
7. Project management tools
Keeping track of your tasks, deadlines, and progress can be streamlined using project management tools like Trello, Jira, or Asana. They let you visualize your workflow, making it easier to manage and prioritize tasks.
8. Continuous learning and upskilling
Lastly, never stop learning. The field of data analysis is continually evolving, with new tools, techniques, and best practices being developed regularly. Regularly dedicating time to learning new skills, either through online courses, reading research papers, or participating in data analysis forums and communities, can help you stay at the forefront of your field.
These strategies will not only make you more efficient at your work but also make your job more satisfying, as you’ll be able to spend more time doing meaningful analysis and less time on tedious, repetitive tasks.
8 Essential qualities to be a high-performing data analyst
A data analyst’s role is crucial in making sense of the vast amounts of data modern businesses collect, helping organizations make informed decisions. But what differentiates an exceptional data analyst from the rest? Here are some qualities that set a strong data analyst apart.
- Analytical mindset
- Proficiency in tools and technologies
- Attention to detail
- Strong mathematical skills
- Problem-solving skills
- Communication skills
- Business acumen
- Continuous learner
Why are they important? Let’s dive right into them.
1. Analytical mindset
One of the most important characteristics of a successful data analyst is an analytical mindset. This means having a natural curiosity to explore data and derive insights. An analytical mindset enables a data analyst to question assumptions, identify patterns, and create hypotheses to test against the data.
2. Proficiency in tools and technologies
Data analysts need to be proficient in a variety of tools and technologies. This includes programming languages like SQL for database queries, Python or R for data cleaning and statistical analysis, and data visualization tools like Tableau or Power BI. A strong data analyst is always looking for ways to expand their toolkit and keep up-to-date with emerging technologies.
3. Attention to detail
Data analysis often involves dealing with complex and large datasets. Attention to detail is key in ensuring the accuracy of the work. It helps identify errors, inconsistencies, or anomalies in the data that could lead to misleading results.
4. Strong mathematical skills
A strong foundation in mathematics, particularly statistics, is vital. From understanding distributions and correlations to hypothesis testing and regression analysis, data analysts use statistical concepts regularly to draw insights from data.
5. Problem-solving skills
Data analysts often encounter challenges that require creative solutions, such as data quality issues or complex business problems. Having strong problem-solving skills allows a data analyst to come up with innovative solutions and make informed decisions.
6. Communication skills
Data analysts don’t work in a vacuum. They often need to present their findings to stakeholders who may not have a technical background. Therefore, strong communication skills, both written and verbal, are essential to explaining complex data insights in a clear and concise way that everyone can understand.
7. Business acumen
Data analysts work with data to solve business problems, so understanding the business context is crucial. This includes knowing the industry, the company’s goals, and how data can be used to support those goals. Good business acumen allows a data analyst to focus on the most relevant insights and make recommendations that have a real impact on the business.
8. Continuous learner
The field of data analysis is continually evolving, with new methodologies, tools, and best practices constantly emerging. A strong data analyst is a lifelong learner who seeks to continuously update their skills and knowledge.
In short, a strong data analyst possesses a mix of technical skills, analytical capabilities, and soft skills. They can not only analyze and interpret complex datasets but also communicate their findings effectively to drive business decisions. By continually improving these qualities, you can thrive and excel in your role as a data analyst.
Recap: What have we learnt so far?
Enhancing data analyst productivity involves refining workflow processes, reusing code snippets, leveraging automation and ETL tools, mastering necessary analytical tools, and implementing version control systems.
Maintaining a data dictionary aids in data understanding, while project management tools streamline task tracking. Additionally, a continuous learning approach ensures analysts stay abreast of evolving trends and techniques.
The focus is on reducing repetitive, manual work and boosting time for in-depth analysis and deriving valuable insights, thereby maximizing the efficacy of a data analyst.
Related reads on data analyst productivity
- Data Vs Metadata
- Data Catalog 101 Guide
- AI Data Catalog
- Active Metadata: 101 Guide From Pioneers Of The Concept
- Metadata management 101: Benefits, tools, and best practices
Share this article