What Is Dark Data and How Can You Make Sense of It?
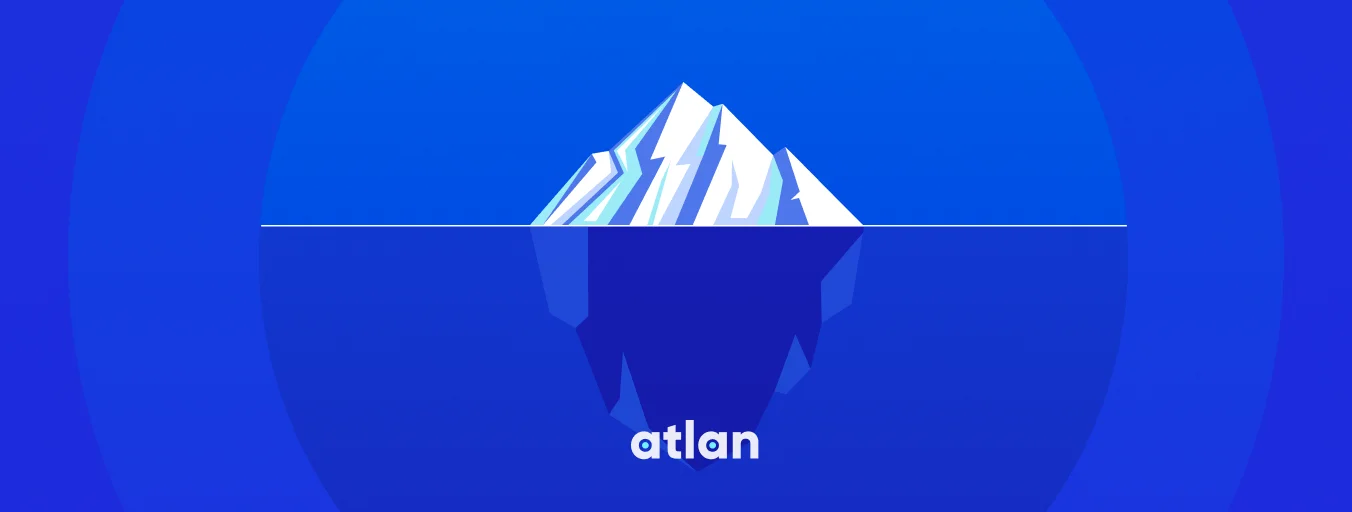
Share this article
Dark data is data that is untapped. Gartner equates it to dark matter in physics — as dark data often makes up most of the information assets belonging to organizations.
While organizations have become adept at collecting large volumes of data, they still struggle with leveraging all that data. On average, more than 50% of a company’s data is considered “dark” — i.e. with no value or meaning assigned to it.
Here we will explore the concept of dark data, its cost, and how to handle it.
Modern data problems require modern solutions - Try Atlan, the data catalog of choice for forward-looking data teams! 👉 Book your demo today
What is dark data? #
Dark data refers to the type of data that was not fully used in a timely manner for its intended purpose. As a result, organizations forget that it’s still a part of their data ecosystem.
Gartner defines dark data as, “the information assets organizations collect, process, and store during regular business activities, but generally fail to use for other purposes (for example, analytics, business relationships, and direct monetizing).”
A large portion of data ends up as dark data because many organizations collect, tag, bookmark, and store data for the purpose of gathering insights. However, they leave it unused, and so it becomes stale over a period of time.
This is similar to a situation that arises while moving houses. You pack all your stuff in boxes. But, even after you’ve moved and set up your new place, there are several unopened boxes that continue to stay in the attic and over a period of time, are forgotten.
Maybe there was something of relevance in those boxes, however, it ends up staying lost in a dark corner of your house.
How is dark data created? #
According to Seagate’s “Rethink Data Report”, only 32% of data available to enterprises is put to work, whereas the remaining 68% goes unleveraged.
The top reasons for this trend include:
- Compliance requirements
- Marketing and sales campaigns
- Customer data from various platforms
Compliance requirements #
Many compliance and governing standards like HIPAA and GDPR force organizations to follow strict regulations for protecting sensitive data.
There’s a cost and time period associated with encrypting or masking, and then storing sensitive data. However, many organizations end up storing them long after the mandatory period and fail to keep track of which data is sensitive or expired.
Campaigns #
Marketing departments, analysts, and data scientists collect and store data for various analyses. This data is supposed to inform strategies for effective outreach, such as branding, sales, or marketing.
This data is not reused in the future or by other departments, thus resulting in data silos. In these scenarios, it’s not uncommon for organizations to forget about these silos and duplicate their efforts in a quest to find other actionable data.
Customer data #
Customer call records, video presentations, and any digital content related to customers are stored in CRMs, sales intelligence platforms, and more. Product teams tend to use this information to refine roadmap decisions, priorities, and even architecture and design decisions.
However, the lifespan of such recordings, emails, and issues from customers is short-lived. Often, they aren’t cleaned up even after consumption, thus resulting in digital hoarding.
What is the cost of dark data? #
When over 68% of the data collected goes unused, that comes at a cost in terms of storage, regulatory risks, and security threats. Let’s look at the cost of dark data along these parameters:
- Data storage: In 2019, a company like Netflix was spending $9.6 million per month for storing its data on AWS. If a large portion of that data gets unused, then organizations are spending millions merely to store dark data.
- Data breach: When you don’t have end-to-end visibility of where your data resides and what it contains, it’s possible to inadvertently make copies in non-secure devices and expose them to security threats. Besides the reputational damage, you also have to pay a fine for exposing customer information to a breach. In 2020, Equifax had to pay $1.38 billion as a settlement for a class action lawsuit due to a data breach incident.
- Data regulation: According to a PWC report, large banks spend around $88 million to collect and store customer data for compliance and regulatory purpose. Most of the data is probably never used but will have to be stored with proper security procedures to comply with data laws and regulations.
- Data R.O.T: With dark data, inaccuracies can become commonplace. Redundant, Obsolete, and Trivial data results in productivity loss whenever wrong data sets get shared for downstream consumption. According to Gartner research, the average financial impact of inaccurate data on organizations was $15 million per year in 2018.
How to discover dark data? #
There are six effective factors for evaluating your data and identifying dark data:
- Staleness
- Popularity score
- Data provenance
- Quality
- Redundancy
- Classification
Here are some questions you should be asking for each of these factors.
Data staleness #
If data has not been modified over a period of time, it is an indication that the data is becoming stale. So, go through your assets and ask yourself, “when was the dataset last updated or modified?”
Low popularity score #
A low popularity score indicates that it is not a widely used or trusted source of information. So, it’s best to evaluate whether any pipelines, models, and BI systems are using an asset.
Missing data provenance #
If there are no data pipelines writing to an asset or reading from it, then it is no longer in mainstream consumption. So, take stock of your assets and ask these questions:
- Is this a siloed dataset?
- How are the upstream and downstream applications using it?
Poor data quality #
Datasets with poor quality (null or duplicate values, incorrect patterns, missing data) will result in providing incomplete or inaccurate insights.
So, they’re candidates for either getting fixed or discarded.
Data redundancy #
Copies of data in multiple systems can be detected using ML techniques like data similarity discovery. You should employ these techniques periodically to get rid of redundant data.
Unclassified data #
Take stock of your unclassified, untagged, and unlabeled data and find out if any of it is sensitive. Not identifying sensitive data or missing data policies can result in data breaches.
How to handle dark data #
Before we get into understanding how to handle dark data, we need to remember that ‘dark’ does not necessarily equate to bad. Dark data is merely data that’s not immediately visible or in a format that is readily consumable.
So, the best way to deal with dark data is to find ways to manage it and reduce the amount of unknown, unclassified data in your ecosystem.
According to Alan Dayley, the research director at Gartner:
“No matter which types of dark data your organization collects, or how it is stored, the key to keeping data out of the dark is to ensure that you have a means of translating it from one form to another and ingesting it easily into whichever analytics platform you use.”
Consider this three-pronged approach to start managing dark data:
- People: Addressing the data culture in your organization
- Processes: Reviewing the current process in place for data management
- Products: Getting technology in place, wherever possible, to scale data management
People #
The first step is to build a strong data culture within your organization. You should:
- Establish a DACI/RACI model to call out the stakeholders and their responsibilities
- Evangelize the need to periodically purge unwanted or stale data, and avoid data duplication
- Onboard new hires and set goals and expectations on data hygiene best practices
Processes #
The next step is to have clear procedures and guidelines for collecting, storing, and using data. You should:
- Set up clear policies on data reuse, data retention, data classification, and sensitive data proliferation
- Establish approval workflows on data usage and track audit and activity details to prevent data spillage
- Set up a cadence of periodic data review (for instance, a Data Week) for everyone in the organization to review usage, and consumption and identify data assets that can be discarded
The best way to establish proper processes is by introducing a solid data governance program.
Products #
The last step is to invest in technology wherever possible to scale your data management practices. You should:
- Adopt and leverage data management tools to collect, organize, classify and identify data and provide point-in-time options to consume this information
- Invest in technology solutions that have the ability to identify and tag R.O.T data and suggest appropriate deletion policies
- Integrate your data stack so that the appropriate data with metrics, such as data freshness, provenance, and quality, are visible across data consumer’s tools of choice
Dark data: Recap #
As mentioned earlier, dark data is the forgotten treasure trove of information in your data ecosystem. Dark data is costly for organizations as it incurs huge storage costs and can attract hefty non-compliance fees.
However, dark data also holds a vast, untapped potential that can give you the edge you need to grow your business.
That’s why it’s crucial to discover whether you have dark data and then find ways to manage it so that you can unlock its full value.
If you’re looking to invest in technology that keeps your data from going dark, you may want to check out Atlan, an active metadata platform.
Some examples of how Atlan can help manage dark data include:
- Reduce pipeline costs by reducing unnecessary data processing and improving resource utilization
- Clean up the data landscape by removing duplicate assets thus reducing costs
- Automatically assign freshness status to assets thus helping identify stale ones
The possibilities are endless. Check out more here.
Atlan Case study: Metadata Management at Wework
Dark data: Related Resources #
- What is modern data stack: History, components, platforms, and the future
- What is a modern data platform: components, capabilities, and tools
- What is data fabric: definition, components, benefits & use cases
- What is Data Mesh?
Share this article