Data Catalog Platform: The Key To Future-Proofing Your Data Stack
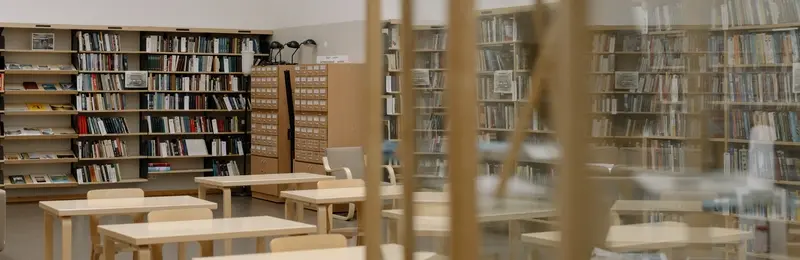
Share this article
Data catalog platforms have become an essential technology for modern enterprises due to their ability to increase data user productivity and connect disparate data elements. Let’s take a closer look at data catalog platforms and why they are the key to future-proofing your data stack.
What is a data catalog platform? #
A data catalog platform is a technology that has the functionality of data catalog software and integrates with other data tools for more effective and efficient data management. It is a type of modern data platform, which MongoDB defines as, “an integrated set of technologies that collectively meet an organization’s end-to-end data needs.” A data catalog platform provides context and trust to end-users and is thus a key driver of data democratization.
What is data democratization? #
Data democratization means that everyone across the organization has the ability to access, understand, and use data to inform decisions. Traditionally IT departments were responsible for data management and governance. Modern workflows require a way for business users to uncover insights without relying on engineering teams.
Furthermore, they need a way to gain context and trust in the data they’re using to drive decisions. The modern data catalog platform provides true data democratization by allowing all users to swiftly and easily access the data they need through features such as Google-like search, quick filters, and data profiling.
What is a modern data catalog? #
Modern data catalogs are the evolution of data catalogs in response to data democratization and other trends. According to Gartner, “The stand-alone metadata management platform will be refocused from augmented data catalogs to a metadata ‘anywhere’ orchestration platform.” Earlier data catalogs required stewards to own and govern data, while modern data catalogs are designed so users can understand data context from within their usual workflow.
Data trends and user desires that fueled the modern data catalog platform #
Cutting-edge tools for data warehousing, data lake storage, data ingestion, and more, all make it very easy to set up and scale up a robust data stack with minimal overhead. However, when it comes to bringing governance, trust, and context to data, the modern data stack is severely lacking. That’s where data catalog platforms come in. Here are some of the key factors that caused this technology to emerge as a solution for uniting these must-have data tools.
Factor #1: The creation of the modern data stack #
Around 2016 the modern data stack — characterized by self-service, agile data management, and cloud-first and cloud-native design — became mainstream. Tools like Fivetran and Snowflake now allow users to set up pipelines and warehouses in under 30 minutes. In this fast-paced world, traditional data catalogs become a bottleneck, with a significant setup time and the need for stewards to own and govern data. This created the demand for next-generation platforms to bring data catalogs up to speed with the rest of the data stack.
Factor #2: The diverse humans of data #
Data teams encompass data engineers, analysts, scientists, product managers, and more. Each of these people has their own unique “data DNA,” with different preferred tools, skills sets, tech stacks, and ways of approaching problems. This diversity brings creative ways of developing solutions but makes collaboration difficult. Modern data catalog platforms need to be intuitive and simple to use so everyone can use them to understand data on their own terms. Data user diversity also means that self-service is no longer optional, it is an essential feature.
Factor #3: The new vision for data governance #
Stakeholders traditionally have seen data governance as a bureaucratic process that hinders their day-to-day work. Modern, collaborative governance requires a new type of data catalog built from the bottom up and needs a reframing as “data and analytics governance” to highlight its importance for bringing clarity and transparency to data analytics.
Factor #4: The rise of the metadata lake #
Big data is exploding: According to G2, businesses generate around two billion gigabytes of data every day. But let’s not forget that an equally vast and rapidly growing amount of metadata accompanies all of this information. To get the most out of metadata, businesses need to store all types of data in a unified repository that is accessible, connected, and usable by both humans and machines.
A metadata lake uses a data lake architecture to build a storage repository for a metadata catalog, expanding the possible uses for metadata beyond today’s use cases like data cataloging, lineage, and observability, to future use cases like automatically fine-tuning data pipelines.
Factor #5: The birth of active metadata #
In August 2021, Gartner scrapped its Magic Quadrant for Metadata Management and replaced it with the Market Guide for Active Metadata Management, signaling a new way of thinking about metadata.
Traditional catalogs are passive, focused on documenting what happened in the past, and rely on human effort to curate and document data. Modern data catalog platforms instead serve as active metadata platforms, continuously collecting metadata from logs and other sources, processing metadata to derive intelligence (such as by automatically creating lineages by parsing through query logs), and transforming passive metadata into active metadata that drives insights like alerts and recommendations in real-time.
Four pillars of the modern data catalog platform that will help future-proof your data stack #
These driving factors are making data scientists think hard about what will define the new generation of data catalog platforms. Here are four elements that have been proven to help organizations unite and future-proof their data stacks. Together, these elements make up the foundation of Data Catalog 3.0, our vision of the modern data catalog platform.
Programmable bots #
Augmented data catalogs that use machine learning to automate manual tasks have become increasingly popular in the past few years. This is a positive trend, but no single machine learning algorithm can magically solve all the data management problems in the world, like creating context, uncovering anomalies, and building intelligent data management.
Data Catalog 3.0 platforms instead rely on programmable bots which allow teams to create their own machine learning or data science algorithms for specific use cases such as security, classification, and observability.
Embedded collaboration #
The diversity of data teams means data catalogs need to integrate seamlessly with the tools stakeholders already use. Embedded collaboration is about work happening where data users already are, such as requesting access to a data asset through a link (as with Google Docs), approving or rejecting a request inside Slack, or triggering a support request on Jira without leaving a data asset. This unifies disparate micro-workflows, making these tasks seamless, efficient, and (ideally) delightful.
End-to-end visibility #
Data Catalog 2.0 tools made significant improvements in data discovery, but they didn’t allow for a single source of truth for data, resulting in frustrating back-and-forths with engineers or executives.
Data Catalog 3.0 tools give a full picture of all the information users need for a given data asset, including information about the ownership (generated from query history), where it comes from (via automated lineage), whether it is trustworthy (based on quality scores and how recently it was updated), which columns are used the most, how people use them, and most importantly, a preview of data itself.
Rather than relying on old-school, top-down governance, this visibility allows organizations to practice federated data governance where standards are defined centrally but individual teams are able to execute them in a way they believe is appropriate for their particular environments.
Open by default #
To better understand and trust data, users need a way to integrate metadata with the rest of their data toolkit. Data catalog platforms should leverage open APIs to connect with the rest of the data stack and maximize the potential of active metadata. By connecting to all other parts of the modern data stack, Data Catalog 3.0 tools will go from passive metadata stores to active tools for improving daily data work. New superpowers like automatically creating column-level lineage from query logs will emerge as a result of this openness.
Employing a data catalog platform to connect the data stack #
This new generation of data catalog tools represents a fundamental jump in how users can understand the context of the data they work with — and trust the insights they gain — in a self-service manner. A big part of this shift is the modern data experience Data Catalog 3.0 tools deliver: Users are able to leverage metadata from within their usual workflows without needing to rely on data stewards working behind the scenes.
With Atlan, all of the essential functionalities of the modern data catalog come together in a single platform that integrates seamlessly with the rest of the modern data stack.
Data catalog platform: Related reads #
- What is a modern data catalog?
- What Is a Data Catalog? & Do You Need One?
- Best Alation Alternative: 5 Reasons Why Customers Choose Atlan
- Data catalog benefits: 5 key reasons why you need one
- What is the difference between data catalog and metadata management?
- Data catalog software: Why it’s essential and how to pick one for your business in 2022
- Enterprise data catalog(EDC): Definition, importance & benefits
Evaluating a data catalog platform for your organization. Do take Atlan for a spin. Atlan is a third-generation modern data catalog built on the framework of embedded collaboration that is key in today’s modern workplace, borrowing principles from GitHub, Figma, Slack, Notion, Superhuman, and other modern tools that are commonplace today.
Share this article