Data Observability: Definition, Key Elements, and Business Benefits
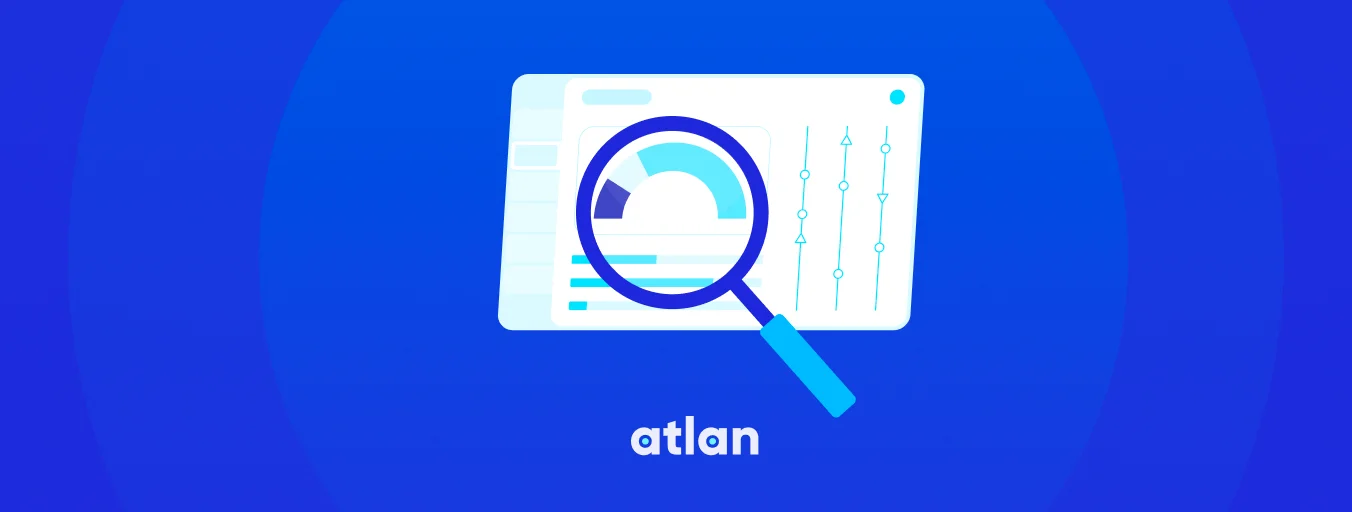
Share this article
What is data observability? #
Data observability is a holistic practice that refers to the ongoing management of data health and usability.
As organizations increasingly rely on data for virtually every aspect of their operations, maintaining data quality and reducing downtime has become a key initiative. Businesses simply can’t afford to let bad data run amuck; MIT estimates the cost of data issues to be 15-25% of revenue for most companies.
Modern data problems require modern solutions - Try Atlan, the data catalog of choice for forward-looking data teams! 👉 Book your demo today
Why is data observability important? #
Data observability is essential for effective DataOps, which is the practice of bringing together people, processes, and technology to enable the agile, automated, and secure management of data.
It’s important to understand that data observability is not a single activity or technology, but rather a collection of activities and technologies that combine to drive operations. Core data observability activities include:
- Data monitoring
- Data alerting
- Data tracking
- Data comparisons
- Data logging
1. Data monitoring #
Data monitoring is the practice of continuously surveilling an organization’s data instances to maintain data quality standards over time. Many businesses use data monitoring software to automate parts of the process and measure data quality KPIs.
Data monitoring vs data observability
While these terms are sometimes used interchangeably, they are not one and the same. Data monitoring focuses on identifying pre-defined issues and “representing data in aggregates and averages.” Data observability is about adding additional contextual information that can be used to make process changes so the issues don’t occur in the first place.
2. Data alerting #
Data alerting goes hand in hand with data monitoring — data alerts notify users when a data asset falls outside the established metrics or parameters. For example, your monitoring tool might alert you when a table with millions of rows suddenly shrinks by 90%.
3. Data tracking #
Data tracking is the process of choosing specific metrics and events, then collecting, categorizing, and analyzing those data points throughout the data pipeline for the purpose of analysis.
4. Data comparison #
Data comparison involves analyzing related data to identify differences and similarities. Combined with monitoring and alerting, comparisons can be used to notify data users about anomalies across data sets.
5. Data logging #
Data logging is the practice of capturing and storing data over a period of time so it can be analyzed to identify patterns and predict future events.
Data observability vs software observability #
Software observability was designed as a way for DevOps teams to keep their finger on the pulse of the health of various systems - in the interest of preventing downtime and predicting future behavior. Similarly, data observability helps data teams understand the health of data throughout their systems, reduce data downtime, and maintain the integrity of increasingly complex data pipelines over time. The foundation of data observability is also related to the foundation of software observability in that they both aim to create systems that prevent issues that can have compounding negative effects over time.
What are the pillars of data observability? #
The pillars of data observability are related to the pillars of software observability (metrics, logs, and traces), but differ slightly. The pillars of data observability are metrics, metadata, lineage, and logs.
1. Metrics #
Metrics are internal characteristics about the quality of the data (completeness, accuracy, consistency, etc.) that can be used to measure both data at rest and data in transit.
2. Metadata #
Metadata refers to external characteristics about data (schema, freshness, volume) that, in tandem with metrics, can be used to identify data quality problems.
Metadata and the hierarchy of data observability
The hierarchy of data observability is a concept coined by Databand: “The level of context you are able to achieve depends on what metadata you are able to collect and provide visibility on … Each level acts as a foundation for the next and allows you to attain a finer grain of observability.” In other words, you must have essential metadata elements in place in order to advance the highest, most valuable level of data observability. Databand defines three levels of the hierarchy of observability:
- Level 1: Operational Health and Dataset Monitoring —This encompasses baseline metadata requirements about data at rest (to determine if it arrived in a timely manner, frequency of updates, etc.) and data in transit (to determine how pipeline performance affects quality, what operations transform the dataset, etc.) that provide visibility into operational and dataset health.
- Level 2: Column-level Profiling — Entails auditing the structure of data tables to solidify rules and create new ones, as needed, at the column level. Investigating the column level is the key to ensuring the data is dependable and consistent.
- Level 3: Row-level Validation — With a solid foundation in place, you can examine the values in each row and validate accuracy to ensure that business rules are being met so desired outcomes are achieved. Many organizations focus myopically on this area, but without working on the first two levels, the results can be muddy.
3. Data Lineage #
Data lineage shows where data comes from and how it evolves throughout its lifecycle. Because datasets within a business are interconnected, lineage is crucial for understanding various data dependencies and the ripple effects that making changes will cause.
4. Data Logs #
Logs capture information about how data interacts with external environments. This includes both machine-machine interactions (such as data replication or transformation) and machine-human interactions (such as the creation of a new data model or consumption of data via a business intelligence dashboard).
Data observability benefits #
Processes, technologies, and frameworks that improve data team productivity are no longer a nice-to-have in today’s business landscape — they are a must-have for accelerating innovation and achieving a competitive advantage. By reducing data downtime and keeping data pipelines running smoothly, data observability enables more productive teams. Additional benefits of data observability include:
- Discovery of data problems before they negatively affect the business
- Timely delivery of high-quality data for business workloads
- Increased trust in data for key business decisions
- Efficient monitoring and alerting that can scale as the business grows
- Improved collaboration among data engineers, scientists, and analysts
It’s important to note that, in order to unlock the full benefits of data observability, all of the components and activities listed throughout this article must be unified. Some organizations practice a siloed version of data observability — individual teams collect and share metadata only on the pipelines they own. A single metadata repository, such as the metadata lake featured in an active metadata platform, is key for giving the entire organization visibility into data and system health.
How does data observability fit into the modern data stack? #
Data observability is vital for keeping the enterprise data stack running as a well-oiled machine rather than a clunky collection of poorly integrated tools. As the modern data stack continues to grow and become more complex, data observability is essential for maintaining quality and keeping a steady flow of data throughout daily business operations.
In the coming years, it will be interesting to see if data observability will continue to be its own category or if it will merge into a broader category, like active metadata. As mentioned above, keeping all your metadata in one open platform enables a variety of use cases, such as data observability, cataloging, lineage, and more. Additionally, an active metadata framework allows data teams to design programmable-intelligence bots that can be used with open-source data observability algorithms to automate advanced DataOps processes for individual data ecosystems and use cases.
Data observability can also be categorized under the burgeoning discipline of data reliability engineering. Similar to data observability’s roots in software observability, data reliability engineering was inspired by the DevOps concept of site reliability engineering. Top proponents of data reliability believe that, in addition to improving data observability, businesses should establish clear service level objectives (SLOs) — as site reliability engineers do — that define target ranges of values a data team aims to achieve. For example, 30 minutes or less of downtime per 500 hours of uptime.
Conclusion #
Data observability is fundamental to data team agility and productivity. The activities and technologies included in data observability (monitoring, alerting, tracking, comparison, and logging) are necessary to help data users understand and maintain the health of their data. As such, a rapidly growing number of data teams are integrating data observability into their strategies and data stacks because it enables optimal performance of DataOps workflows.
Learn more about the other core concepts that make up the future of the modern data stack.
Photo by ThisIsEngineering from Pexels
Share this article