10 Steps to Create an Effective Data Foundation
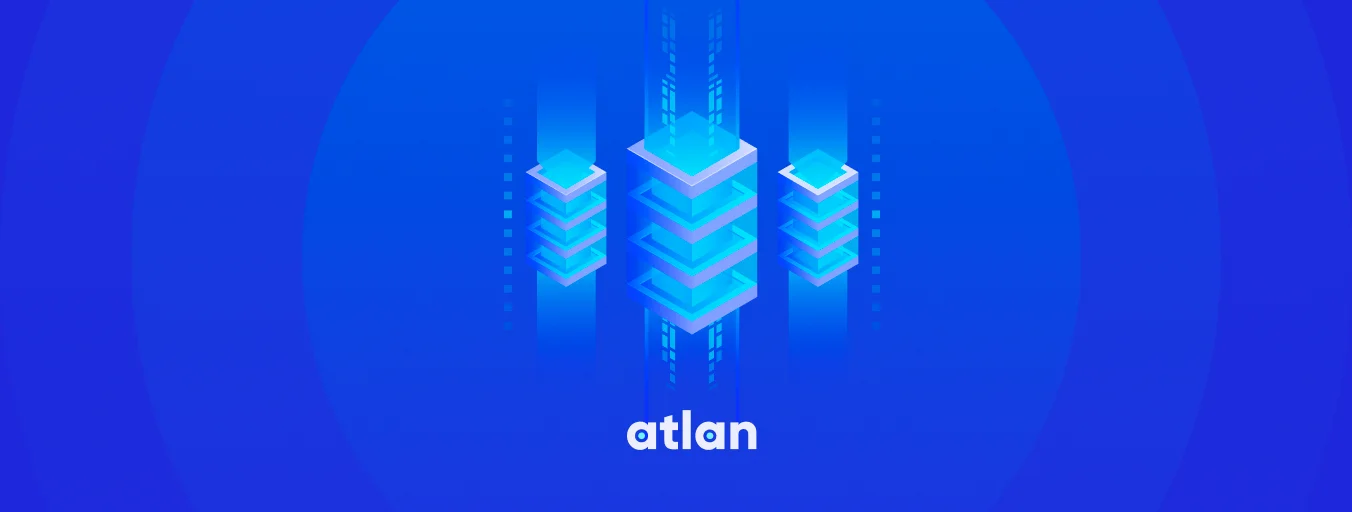
Share this article
At its core, a data foundation encompasses the fundamental elements and structures that provide the groundwork for effective data management, analysis, and utilization. It involves the seamless integration of data from various sources, ensuring its quality, consistency, and accessibility.
This architecture enables data to be collected, stored, processed, and distributed in a manner that supports both immediate operational needs and future strategic initiatives.
Modern data problems require modern solutions - Try Atlan, the data catalog of choice for forward-looking data teams! 👉 Book your demo today
In this article, we will learn about the data foundation architechture, uncover its transformative potential, and equip enterprises with the knowledge to unlock lasting value from their most valuable resource — data.
Let’s dive in!
Table of contents #
- What is data foundation?
- 10 Steps to create an effective data foundation
- Data foundation architecture
- Data foundation layers
- Purpose of an intelligent data foundation
- Benefits of building a strong data foundation
- Challenges of building a strong data foundation
- Summary
- Data foundation: Related reads
What is data foundation? #
A data foundation refers to the fundamental infrastructure, processes, and strategies that lay the groundwork for effectively collecting, managing, storing, organizing, and leveraging enterprise data.
It encompasses a set of principles, technologies, and practices designed to ensure data quality, accessibility, security, and usability. This enables organizations to derive valuable insights and make informed decisions from their data assets.
According to the IDC, the global data sphere will grow to 175 zettabytes by 2025, emphasizing the critical need for scalable, flexible, and agile data foundations.
Organizations that strategically implement future-proof data foundations are better positioned to exploit emerging technologies, maintain data integrity and security, and adapt to the continuously changing business landscape, ensuring long-term sustainability and progress.
Key features of a data foundation:
- Data strategy and governance
- Data integration and accessibility
- Data quality and cleansing
- Data storage and architecture
- Master data management (MDM)
- Accessibility and usability
- Flexibility for data types
- Future-proofing for innovation
Let us understand each of them in detail:
1. Data strategy and governance #
A data foundation begins with a well-defined data strategy that aligns with business goals.
- Data governance policies are established to guide how data is collected, stored, accessed, and maintained.
- It involves defining roles, responsibilities, and processes to ensure data accuracy, compliance with regulations, and protection against breaches.
- A robust data governance framework provides transparency and accountability while fostering trust among stakeholders.
2. Data integration and accessibility #
The foundation integrates data from various sources, whether internal or external, structured or unstructured.
- Data integration involves the seamless aggregation of data from various sources, both internal and external, into a unified platform.
- This process ensures that data can be accessed and analyzed without hindrance, breaking down silos that often inhibit cross-functional collaboration.
- It involves the use of ETL (Extract, Transform, Load) processes, APIs, and connectors to bring data from diverse systems together.
- It ensures seamless connectivity and accessibility for authorized users across the organization, breaking down data silos and promoting a unified view of data.
3. Data quality and cleansing #
To maximize the value of data, a data foundation focuses on maintaining data quality.
- Data quality is paramount for any successful data foundation.
- Ensuring that the data is accurate, complete, consistent, and up-to-date is essential for making informed decisions.
- Data quality assurance involves processes like data cleansing, validation, and enrichment.
- This step helps in minimizing errors and inconsistencies that can arise due to human input, system errors, or data migrations.
- This involves thorough data cleansing, validation, and enrichment processes to ensure accuracy and reliability.
4. Data storage and architecture #
An optimized data storage architecture is a cornerstone of the data foundation.
- The architecture design of a data foundation needs to be scalable to accommodate the increasing volume, velocity, and variety of data.
- This often involves choosing appropriate database systems, data warehousing solutions, cloud services, and frameworks that can handle the data growth over time.
- A well-designed architecture ensures that the data foundation remains responsive and adaptable to changing business needs.
- It defines where and how data is stored, considering factors like scalability, performance, security, and cost-effectiveness.
- This could involve a combination of on-premises and cloud-based solutions.
5. Metadata management #
Metadata — data about data — is crucial for understanding and managing data assets.
- Metadata, which provides context and information about the data, is a vital aspect of a data foundation.
- Effective metadata management helps users understand the origin, meaning, and relationships of data elements.
- It aids in discovering relevant data, understanding its lineage, and maintaining a clear data dictionary, enhancing overall data comprehension.
- A data foundation establishes metadata management practices to capture information about data sources, definitions, transformations, and usage.
6. Accessibility and usability #
A data foundation should make data easily accessible to authorized users across the organization.
- User-friendly interfaces and tools facilitate data exploration, analysis, and reporting, even for those without advanced technical skills.
- Self-service analytics empower business users to retrieve insights on their own, reducing dependency on IT teams for data access.
7. Flexibility for data types #
Modern data foundations must be adaptable to various data types, including structured, semi-structured, and unstructured data.
- This versatility enables organizations to harness insights from sources like text, images, videos, social media posts, sensor data, and more.
- A flexible foundation accommodates diverse data formats.
- This enables advanced analytics techniques like natural language processing and machine learning.
8. Future-proofing for innovation #
A future-proof data foundation not only ensures that an organization’s current data needs are met but also that it’s poised to seamlessly integrate and leverage future advancements in the data and tech landscape.
- It anticipates technological advancements, changing data landscapes, and evolving business requirements.
- By incorporating open standards, modular components, and flexible architectures.
- The foundation can readily embrace new data sources, tools, and technologies as they emerge.
In essence, a data foundation serves as the bedrock for data-driven decision-making. By establishing a well-structured, well-governed, and well-managed data environment, organizations can significantly reduce the challenges associated with data utilization.
10 Steps to create an effective data foundation #
Creating a data foundation involves establishing a comprehensive framework for managing an organization’s data assets. This process is fundamental for organizations to leverage their data effectively for decision-making, insights, and maintaining competitive advantage.
Here’s a detailed explanation of how to create a robust data foundation:
- Understanding business objectives
- Data governance
- Data architecture and infrastructure
- Data integration and warehousing
- Data quality management
- Data accessibility and democratization
- Analytics and business intelligence (BI)
- Scalability and flexibility
- Backup and disaster recovery
- Continuous improvement and innovation
Let us understand each of them in detail.
1. Understanding business objectives #
- Align with business goals: Identify and understand the business goals that the data foundation will support. This alignment ensures that the data strategy is relevant and adds value to the organization.
- Stakeholder engagement: Involve stakeholders from different departments to understand their data needs and challenges.
2. Data governance #
- Establish data governance framework: Implement policies, procedures, and standards for data management. This includes defining roles and responsibilities for data ownership, quality, security, and compliance.
- Compliance and security: Ensure adherence to legal and regulatory requirements related to data, such as GDPR, HIPAA, etc.
3. Data architecture and infrastructure #
- Design data architecture: Develop a scalable and flexible data architecture that supports current and future needs. This includes choosing between cloud-based or on-premises solutions, data warehouses, data lakes, etc.
- Invest in infrastructure: Select and implement the necessary hardware and software infrastructure that aligns with the designed data architecture.
4. Data integration and warehousing #
- Integrate data sources: Consolidate data from various sources, ensuring it can be accessed and used effectively. This may involve ETL (Extract, Transform, Load) processes.
- Data warehousing: Create a centralized repository (data warehouse) where integrated data can be stored and accessed.
5. Data quality management #
- Implement data quality measures: Establish processes for ongoing data quality management, including data cleaning, deduplication, and validation.
- Continuous monitoring: Regularly monitor and audit data quality, making improvements as needed.
6. Data accessibility and democratization #
- User access controls: Define who has access to what data and under what circumstances, ensuring data security while making data accessible to those who need it.
- Promote data literacy: Educate and train staff on data concepts and the importance of data-driven decision-making.
7. Analytics and business intelligence (BI) #
- Implement BI tools: Deploy tools that allow for data analysis, reporting, and visualization to support decision-making processes.
- Support advanced analytics: Prepare the foundation for more sophisticated analytics, such as predictive analytics and machine learning.
8. Scalability and flexibility #
- Plan for growth: Ensure that the data foundation can scale with the growth of the business. This includes scalable infrastructure and flexible data models.
9. Backup and disaster recovery #
- Implement robust backup solutions: Regularly back up data to protect against data loss.
- Disaster recovery planning: Develop and maintain a disaster recovery plan to ensure data availability and business continuity in case of emergencies.
10. Continuous improvement and innovation #
- Iterative approach: Continuously evaluate and improve the data foundation. Stay abreast of emerging technologies and practices in data management.
Creating a data foundation is not a one-time project but an ongoing process. It requires a strategic approach, investment in technology and people, and a commitment to maintaining high-quality data practices.
As technology and business needs evolve, so should the data foundation, making it a dynamic and integral part of the organization’s strategy.
Data foundation architecture: Establishing a solid data infrastructure #
Data foundation architecture refers to the structured framework that outlines the design, components, and interactions necessary to create a robust infrastructure for collecting, storing, managing, processing, and analyzing enterprise data.
This architecture serves as the blueprint for building a strong data foundation that supports data-driven decision-making and business growth.
Steps to build a data foundation architecture:
- Identify business goals
- Assess data assets
- Define data governance
- Plan data integration
- Architecture design
- Develop ETL processes
- Build data storage
- Implement data governance tools
- Establish security measures
- Set up analytics tools
- Monitor and optimize
- Educate and train
- Evolve and adapt
Let us understand each oh the steps in detail:
1. Identify business goals #
Before delving into technical details, it’s essential to understand the organization’s business goals and align the data foundation with these objectives.
- Identify what insights you need to derive from the data, how it will impact decision-making, and what challenges you aim to address.
- This strategic vision will guide the architecture’s design.
- Define clear business objectives that the data foundation should support.
- This could range from improving decision-making to enhancing customer experiences.
2. Assess data assets #
Conduct a comprehensive assessment of existing data sources, formats, quality, and accessibility.
- Identify gaps and opportunities for improvement. Identify all the data sources across the organization.
- These could include databases, applications, spreadsheets, IoT devices, and more.
- Create a comprehensive inventory to understand the scope and variety of data you’ll be working with.
3. Define data governance #
Develop data governance policies, including data ownership, access rights, data quality standards, and compliance procedures.
- Implement data governance policies and procedures to ensure data security, compliance, and access control.
- Define roles and responsibilities, establish data ownership, and set up mechanisms to monitor and audit data usage.
4. Plan data integration #
Determine data sources, integration methods, and data flow architecture.
- Create a roadmap for extracting, transforming, and loading data into the foundation.
- Integrate data from disparate sources into a centralized repository or data lake.
- This step involves data extraction, transformation, and loading (ETL) processes to ensure consistency and compatibility.
- Modern architectures often utilize data integration platforms and tools to streamline this process.
5. Architecture design #
Design the architecture based on the types of data you’re working with and your business requirements.
- Consider aspects like data storage, data processing, data access, scalability, and security.
- Common architectural options include data warehouses, data lakes, and hybrid solutions.
6. Develop ETL processes #
Design and implement ETL processes that transform and load data into the foundation. Focus on data cleansing, transformation, and validation.
-
Extract:
- The primary step involves pulling data from diverse sources, which can range from databases, cloud platforms, APIs, logs, web scrapers, or even flat files.
- Ensure that the extraction process can handle connectivity issues, source changes, or unexpected data without failing outright.
-
Transform:
- Once extracted, the data might not be in a suitable or consistent format.
- Transformation processes make the data cohesive, clean, and compatible for analytics.
- Ensure that data from diverse sources aligns to a consistent schema or structure.
-
Load:
- This phase involves moving the transformed data into a final destination, such as a data warehouse, where it’s ready for analysis.
- Depending on timeliness requirements, decide between batch loading (all at once) or stream loading (continuous).
7. Build data storage #
Create a data storage infrastructure that aligns with scalability, performance, and security requirements.
- Select appropriate data storage solutions based on the nature of your data.
- For structured data, relational databases might be suitable, while unstructured or semi-structured data could be stored in NoSQL databases or object storage systems.
8. Implement data governance tools #
Utilize tools for data quality monitoring, metadata management, access controls, and data lineage tracking.
- Implement data governance policies and procedures to ensure data security, compliance, and access control.
- Define roles and responsibilities, establish data ownership, and set up mechanisms to monitor and audit data usage.
9. Establish security measures #
Implement security measures such as encryption, access controls, and data masking to safeguard sensitive data.
- Integrate robust security measures to protect sensitive data from breaches and unauthorized access.
- Encryption, authentication, and access controls are vital components of a secure data foundation.
10. Set up analytics tools #
Deploy analytics and visualization tools that enable data exploration and insights generation.
- Beyond simple visualizations, modern analytics tools offer predictive modeling, machine learning, and more.
- If leveraging predictive analytics, ensure there’s a mechanism to train, test, and refine models.
- Implement ways to gather feedback on analytics predictions or outcomes to improve accuracy over time.
11. Monitor and optimize #
Regularly monitor data quality, system performance, and user feedback. Optimize the architecture based on insights gathered.
- Building a data foundation is an ongoing process.
- Implement monitoring and performance tracking mechanisms to identify bottlenecks, anomalies, and areas for improvement.
- Regularly assess the architecture against changing business needs.
12. Educate and train #
- Provide training to users, data scientists, and analysts on how to effectively use the data foundation for insights and decision-making.
- Educate your team about the newly established data foundation.
- Provide training on data tools, analytics platforms, and best practices to ensure effective utilization.
13. Evolve and adapt #
As business needs change and technology evolves, continuously assess and update the data foundation architecture to ensure its relevance and effectiveness.
- As your business evolves, so should your data foundation.
- Regularly reassess your architecture to accommodate new data sources, technologies, and business objectives.
By meticulously following these steps, organizations can construct a robust data foundation architecture that empowers them to unlock the true value of their data assets.
Data foundation layers: Structuring data for success #
A well-structured data foundation comprises several layers, each serving a specific purpose in managing, processing, and extracting value from data.
These layers work cohesively to create a robust data ecosystem that supports effective data management and utilization.
Let’s delve into the layers:
- Data collection layer
- Data storage layer
- Data processing layer
- Data integration and master data management (MDM) layer
- Data governance and security layer
- Metadata management layer
- Analytics and visualization layer
- Data access and API layer
- Continuous monitoring and improvement layer
Let us understand each layer in detail:
1. Data collection layer #
At the foundation of the architecture lies the data collection layer. This layer focuses on gathering data from various sources, whether internal or external, structured or unstructured. It involves the following key aspects:
- Data sources: Identify and integrate relevant data sources, such as databases, APIs, sensors, and third-party data providers.
- Data ingestion: Establish mechanisms to capture and ingest data in real-time or batch mode, ensuring seamless integration into the foundation.
- Data transformation: Perform initial data transformations to convert raw data into a consistent format suitable for further processing.
2. Data storage layer #
This layer is responsible for storing the ingested data in a way that ensures accessibility, scalability, and data integrity. Key considerations include:
- Storage technologies: Select appropriate storage technologies such as relational databases, NoSQL databases, data lakes, and cloud-based solutions.
- Data partitioning: Organize data into partitions or segments to optimize retrieval and storage efficiency.
- Data replication and backup: Implement redundancy and backup strategies to ensure data availability and disaster recovery.
3. Data processing layer #
The data processing layer focuses on transforming, enriching, and aggregating data to make it ready for analysis. Core components include:
- ETL (Extract, Transform, Load): Design and deploy ETL processes to clean, transform, and consolidate data from various sources.
- Data enrichment: Enhance data by adding context, metadata, and additional attributes to provide a more comprehensive view.
- Data validation: Apply validation rules to identify and rectify data quality issues during processing.
4. Data integration and master data management (MDM) layer #
This layer ensures consistency and coherence across data sources and domains. It involves:
- Data integration: Integrate data from different sources, synchronizing them to provide a unified view of relevant information.
- Master data management (MDM): Identify and manage master data entities such as customers, products, and locations to maintain consistency and accuracy.
5. Data governance and security layer #
The data governance and security layer ensures data compliance, security, and access control. It encompasses:
- Data governance policies: Define rules, policies, and ownership responsibilities for data quality, data usage, and compliance with regulations.
- Access control: Implement role-based access controls (RBAC) to restrict data access based on user roles and responsibilities.
- Data security: Employ encryption, masking, and anonymization techniques to safeguard sensitive data.
6. Metadata management layer #
Metadata provides context about the data, aiding understanding and management. This layer involves:
- Metadata capture: Document metadata elements that describe data sources, transformations, and relationships.
- Metadata repository: Create a centralized repository to store and manage metadata, enabling data discovery and lineage tracking.
7. Analytics and visualization layer #
At the top of the architecture, the analytics and visualization layer enables data exploration, analysis, and insights generation:
- Analytics tools: Employ tools like data warehouses, big data platforms, and machine learning frameworks for advanced analysis.
- Data visualization: Utilize visualization tools to present data insights in understandable and actionable formats.
8. Data access and API layer #
This layer facilitates data consumption by various applications and users:
- APIs and data services: Develop APIs to expose data to internal systems, third-party applications, and external stakeholders.
- Data access control: Enforce access controls at this layer to ensure data security and compliance.
9. Continuous monitoring and improvement layer #
This ongoing layer involves continuous monitoring, optimization, and adaptation of the data foundation:
- Data quality monitoring: Set up automated checks to monitor data quality and trigger alerts in case of anomalies.
- Performance optimization: Continuously monitor system performance, resource utilization, and query efficiency.
By carefully constructing each of these layers within your data foundation architecture, organizations can establish a well-organized, secure, and scalable environment.
What is the purpose of an intelligent data foundation? #
An intelligent data foundation represents an advanced evolution of traditional data management practices. It leverages cutting-edge technologies and methodologies to not only manage data efficiently but also to extract insights, predict trends, and enable proactive decision-making.
Let us look at the different purposes of data foundation:
- Comprehensive data management
- Real-time data processing
- Advanced analytics and insights
- Predictive capabilities
- Personalization and customer insights
- Proactive decision-making
- Agility and adaptability
- Cost efficiency
- Competitive edge
- Data monetization
Let us understand each of the above aspects in detail:
1. Comprehensive data management #
At its core, an intelligent data foundation is built to manage vast and diverse data sets.
It provides the infrastructure to :
- Collect
- Store
- Organize
- Process data from a multitude of sources, regardless of its volume, velocity, or variety.
2. Real-time data processing #
An intelligent data foundation enables real-time data processing, allowing organizations to respond swiftly to changing conditions and emerging opportunities.
This is especially crucial in industries where milliseconds matter, such as financial services, supply chain management, and IoT applications.
3. Advanced analytics and insights #
Beyond basic data storage, an intelligent data foundation empowers organizations to perform advanced analytics.
It facilitates the application of:
- Machine learning algorithms
- Predictive modeling
- Artificial intelligence techniques to uncover hidden patterns, correlations, and insights within the data.
4. Predictive capabilities #
By analyzing historical data, an intelligent data foundation can predict future trends, behaviors, and outcomes.
This predictive capability enhances organizations’ ability to plan, strategize, and allocate resources effectively.
5. Personalization and customer insights #
In customer-centric industries, an intelligent data foundation enables personalized experiences by analyzing customer data to understand preferences, behaviors, and needs.
This allows organizations to tailor offerings and interactions, enhancing customer satisfaction and loyalty.
6. Proactive decision-making #
Equipped with real-time insights and predictive analytics, an intelligent data foundation empowers organizations to make proactive decisions.
Instead of reacting to events, they can anticipate trends, mitigate risks, and capitalize on opportunities before they fully manifest.
7. Agility and adaptability #
An intelligent data foundation is designed for agility and adaptability.
It can accommodate:
- Changing data requirements
- New sources of information
- Evolving technologies without requiring a complete overhaul of the infrastructure.
8. Cost efficiency #
While implementing an intelligent data foundation involves investments in technology and resources, the long-term benefits outweigh the costs.
Organizations save time and resources by automating data processing, reducing manual efforts, and avoiding the consequences of poor decision-making due to incomplete or outdated data.
9. Competitive edge #
In a data-driven world, an intelligent data foundation confers a significant competitive edge.
Organizations that harness their data to derive insights and drive innovation are better positioned to outperform competitors, capture market share, and stay relevant.
10. Data monetization #
For many organizations, data has become a valuable asset in its own right.
An intelligent data foundation enables data monetization by identifying opportunities to sell or share data, creating new revenue streams, and fostering partnerships.
In summary, the purpose of an intelligent data foundation is to empower organizations with the tools and capabilities needed to turn raw data into actionable insights.
10 Benefits of building a strong data foundation #
Let us understand the benefits:
- Informed decision-making
- Data-driven culture
- Improved operational efficiency
- Enhanced customer experiences
- Competitive advantage
- Quick and accurate reporting
- Proactive issue detection
- Predictive analytics
- Effective resource allocation
- Scalability and growth
Let us understand each of them in detail:
1. Informed decision-making #
A robust data foundation provides reliable, accurate, and up-to-date information. This empowers decision-makers at all levels with the insights they need to make informed choices, resulting in better outcomes and improved strategic planning.
2. Data-driven culture #
A strong data foundation promotes a data-driven culture within the organization. When data is readily available and trustworthy, employees are more likely to base their decisions on evidence rather than intuition.
3. Improved operational efficiency #
Organizations with a solid data foundation can streamline operations by automating processes, identifying bottlenecks, and optimizing workflows. This efficiency leads to cost savings and increased productivity.
4. Enhanced customer experiences #
Accurate customer data allows organizations to personalize interactions, understand preferences, and address needs effectively. This leads to improved customer satisfaction and loyalty.
5. Competitive advantage #
Having a strong data foundation enables organizations to stay ahead of competitors by leveraging insights to identify trends, anticipate market changes, and innovate more effectively.
6. Quick and accurate reporting #
With data readily available and well-organized, generating reports becomes faster and more accurate. This is crucial for compliance, financial reporting, and performance tracking.
7. Proactive issue detection #
By analyzing historical and real-time data, organizations can detect anomalies and issues early on, allowing them to take corrective actions before problems escalate.
8. Predictive analytics #
A strong data foundation enables the application of predictive analytics, helping organizations forecast trends, outcomes, and customer behaviors. This informs strategic planning and resource allocation.
9. Effective resource allocation #
Insights derived from a solid data foundation guide organizations in allocating resources, both human and financial, to areas that offer the greatest returns.
10. Scalability and growth #
As organizations expand, a strong data foundation accommodates increasing data volumes and complexity. It supports growth without sacrificing data quality or accessibility.
It elevates an organization’s ability to make informed decisions, drive operational efficiency, and foster innovation, ultimately leading to improved customer satisfaction, competitive advantage, and sustained growth.
8 Challenges of building a strong data foundation #
Certainly, building a strong data foundation comes with its own set of challenges that organizations need to address.
These are as follows:
- Data quality and cleansing
- Data integration complexity
- Data governance
- Legacy systems integration
- Scalability
- Cost considerations
- Change management
- Data retention and lifecycles
Let’s delve into these challenges in detail:
1. Data quality and cleansing #
Maintaining data quality is a major challenge. Inaccurate, incomplete, or inconsistent data can lead to flawed insights and decisions. Cleaning and ensuring data accuracy requires dedicated efforts, automated processes, and ongoing monitoring.
2. Data integration complexity #
Integrating data from various sources with different formats, structures, and systems can be complex. Overcoming integration challenges demands careful planning, standardized data formats, and robust ETL processes.
3. Data governance #
Implementing effective data governance practices is challenging. Organizations need to define:
- Roles
- Responsibilities
- Policies for data ownership, security, privacy, and compliance.
Lack of proper governance can lead to data silos, security breaches, and regulatory issues.
4. Legacy systems integration #
Integrating a strong data foundation with existing legacy systems can be difficult. Compatibility issues, data migration complexities, and the need for modernization can pose significant challenges.
5. Scalability #
As data volumes grow, scaling the infrastructure to accommodate increased storage and processing requirements becomes a challenge. Scalability demands careful architecture planning, efficient resource allocation, and technology selection.
6. Cost considerations #
Investing in technology, tools, training, and skilled personnel can be expensive. Organizations must balance the cost of building a data foundation with the potential long-term benefits.
7. Change management #
Adopting a data-driven culture and getting employees to embrace new processes and technologies can be met with resistance. Change management strategies are necessary to ensure successful adoption.
8. Data retention and lifecycles #
Managing data throughout its lifecycle, including archiving, retention, and disposal, can be complex. Organizations need to determine data retention policies and processes compliant with regulatory requirements.
Addressing these challenges demands a comprehensive approach, a commitment to ongoing improvement, and collaboration across different teams and departments.
Summarizing it all together #
As data continues to proliferate and evolve, a solid data foundation becomes not just an advantage, but a necessity. It becomes the solid ground on which organizations can stand confidently amidst the shifting sands of the business landscape.
In an era where data has emerged as the lifeblood of modern enterprises, building and nurturing a data foundation is akin to investing in the very essence of growth and sustainability.
Data foundation: Related reads #
- Data Governance Policy — Examples & Templates
- What is a data lakehouse: Definition, architecture, components, and use cases.
- What is a data lake: Definition, examples, architecture, and solutions.
- Data mesh vs data lake: What are the differences in architecture, use cases, and benefits?
- Data Warehouse vs Data Lake vs Data Lakehouse: What are the key differences?
- Top data catalog use cases
Share this article