How to Fix Your Data Quality Issues: 6 Proven Solutions!
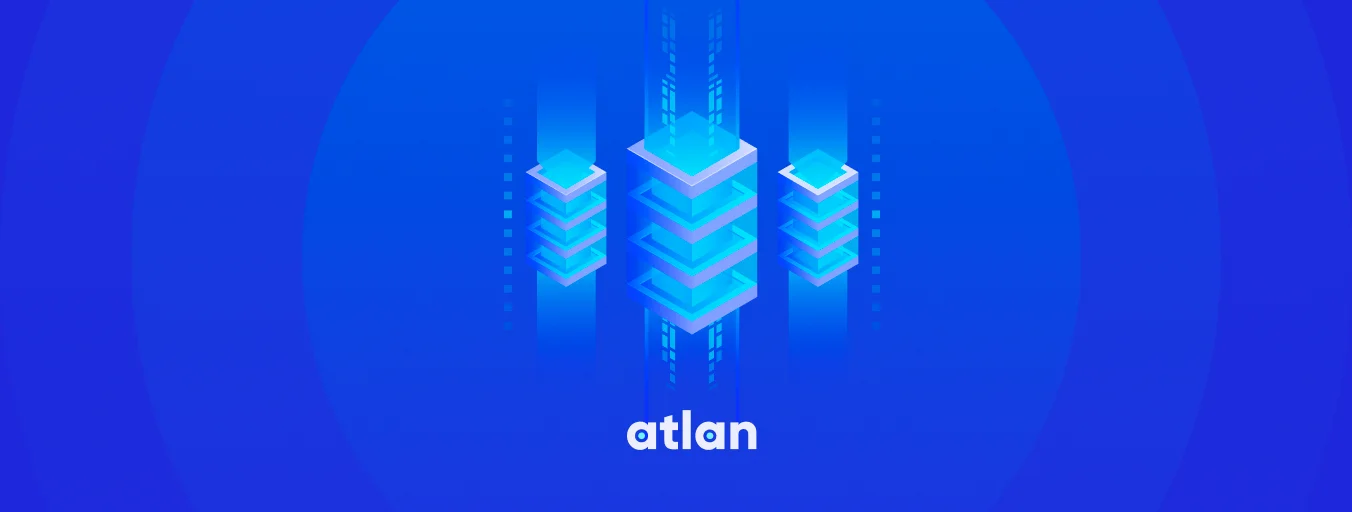
Share this article
In a data-driven world, top-quality data is key for sound decisions, insights, and optimal performance.
But the larger and more complex your data becomes, the more you’re exposed to issues that can degrade its quality. These issues can muddle your analytics, impair decision-making, and even have regulatory implications.
Modern data problems require modern solutions - Try Atlan, the data catalog of choice for forward-looking data teams! 👉 Book your demo today
In this article, we will understand:
- 6 data quality issues and how you should fix them?
- Common mistakes that trigger data quality problems
- 9 steps to fix you data quality problems
Ready? Let’s dive in!
Table of contents #
- 6 Major data quality issues and how to fix them
- 6 Common mistakes that trigger data quality issues
- How to identify data quality issues: 9 Step roadmap!
- 10 Steps for improving your data management practices
- Conclusion
- How to fix your data quality issues: Related reads
6 Major data quality issues and how to fix them #
When we use data to make decisions, it’s really important that the data is reliable and accurate. But sometimes, there are problems with the data that can cause mistakes or confusion.
These are called data quality issues. Some common issues include having the same data repeated, having wrong information, using old data, or not having all the needed information.
The good news is that there are ways to fix these problems. This helps us make better decisions and get the most out of our data.
Let’s take a look at some of these common issues and how we can solve them.
- Duplicate data
- Inaccurate data
- Outdated information
- Missing values
- Non-standardized data
- Data security and privacy
Let’s delve into these challenges and their solutions.
1. Duplicate data #
The problem: Duplicate records can occur for various reasons, including human error during data entry or system glitches. They inflate the size of your databases, wasting storage resources and skewing analytics results.
The solution: Regular database audits are crucial. Automated de-duplication tools can scan your datasets and flag duplicate entries, allowing you to merge or delete them as appropriate.
Consistent record-keeping and unique identifiers can also help in preventing duplicates.
2. Inaccurate data #
The problem: Data inaccuracies can arise from typos, misinformation, or outdated entries. These inaccuracies can lead to flawed insights and misguided decision-making.
The solution: Implement validation rules and data verification processes during data entry. For instance, if you are collecting emails, ensure that the format is checked to be valid before submission. Real-time alerts for potential errors can be helpful.
3. Outdated information #
The problem: Data can become obsolete quickly, particularly in fast-moving industries. Relying on outdated data can misguide strategic decisions and resource allocation.
The solution: Establish a data update schedule and adhere to it. Automated systems can flag old data, suggesting it for review. Retire obsolete data and refresh your databases with current information.
4. Missing values #
The problem: Gaps in your data can severely impact analyses and result in misleading insights. Sometimes missing data can be more harmful than incorrect data.
The solution: Employ imputation techniques to estimate missing values. In some cases, it might be more effective to collect the missing data directly, if possible. Flagging these gaps for future data collection can also be useful.
5. Non-standardized data #
The problem: Different formats, units, or terminologies across data sources can hamper effective data analysis, making it difficult to compare or aggregate data.
The solution: Standardization should be enforced at the point of data collection. Make sure to specify the required formats and units, and apply naming conventions consistently across datasets.
6. Data security and privacy #
The problem: Inadequate data security protocols can lead to unauthorized data access, which not only risks the data itself but can also have legal ramifications.
The solution: Ensure you’re complying with data privacy regulations like GDPR or CCPA. Implement robust encryption methods and secure data access protocols.
Regular security audits can help identify vulnerabilities. By proactively addressing these common data quality issues, organizations can significantly improve the reliability and usefulness of their data. This, in turn, enables better decision-making, more effective strategies, and ultimately, greater success in achieving business objectives.
With that being said, let’s now understand some of the root causes that trigger data quality issues.
6 Common mistakes that trigger data quality issues #
In the realm of data-driven decision-making, the significance of accurate data cannot be overstated. However, data quality issues remain a persistent challenge. These issues stem from various factors, including the following:
- Human errors
- Lack of standardization
- Data integration complexities
- Legacy systems
- Incomplete data
- Poor data governance
A closer examination of these contributing factors unveils the intricacies of maintaining data integrity in today’s information-rich landscape. So, Let’s dive in!
1. Human error #
The problem: Mistakes during data entry are common. Something as simple as a typo or choosing the wrong option from a dropdown menu can introduce errors that have ripple effects throughout your entire data set.
The impact: Take, for example, a customer’s birth year that is entered as 1992 instead of 1982. This seemingly small mistake could affect everything from targeted marketing strategies to demographic analyses, misleading businesses into tailoring products or services to the wrong age group.
2. Lack of standardization #
The problem: When data is collected from multiple sources or input by different team members, inconsistencies can occur. One department might record revenue in USD, while another uses EUR, creating confusion.
The impact: Lack of standardization can distort assessments and lead to incorrect analyses. For instance, if revenue data is provided in different currencies without clarification, your assessment of overall revenue could be drastically skewed.
3. Data integration complexities #
The problem: Many businesses find themselves needing to combine datasets, perhaps following a company merger, or when trying to offer a consolidated customer experience by linking various platforms.
The impact: The process is fraught with risk if not handled meticulously. Any discrepancies in data structure or format between the two datasets can result in duplicated entries or even data loss, potentially harming customer relationships.
4. Legacy systems #
The problem: Outdated technology platforms and legacy systems are often not equipped to handle today’s massive data volumes or complex formats.
The impact: Imagine an old inventory management system attempting to process real-time sales data from an e-commerce platform.
The result would be delays in data processing, and consequently, inaccurate inventory levels, leading to either overstocking or stockouts.
5. Incomplete data #
The problem: Sometimes, you might not have all the data you need for a comprehensive analysis. This could be because the data was never collected, or perhaps it was lost or deleted.
The impact: Incomplete data can render an analysis or report meaningless. In an HR context, for example, missing performance metrics for some employees can make an assessment of overall employee performance skewed or incomplete, thereby affecting strategic decisions.
6. Poor data governance #
The problem: Without well-defined roles and processes for data management, accountability for data quality can become unclear.
The impact: Let’s say no one is specifically tasked with updating and verifying customer contact information in your CRM.
Over time, the CRM could become filled with outdated and incorrect information, negatively impacting marketing campaigns and customer service.
By understanding these various causes in greater detail, you can take targeted steps to mitigate their impact and improve the quality of your data. Each of these issues requires a different set of strategies and tools for resolution, underscoring the need for a comprehensive and ongoing approach to data quality management.
How to identify data quality issues: 9 Step roadmap! #
Identifying data quality issues is a critical step in improving the overall quality of your data.
Here are some strategies and techniques for doing so:
- Manual inspection
- Data profiling
- Data auditing tools
- Visual analytics tools
- User feedback
- Periodic audits
- Business rule validation
- Peer reviews
- Leverage machine learning
Lets explore each step in detail.
1. Manual inspection #
- Random sampling: Take a random sample of your data and inspect it manually. Look for inconsistencies, missing values, or anomalies that stand out.
- Review metrics: Key Performance Indicators (KPIs) or other metrics may show signs of data issues. For example, a sudden drop in customer engagement could be due to data quality problems.
2. Data profiling #
- Frequency distribution: Check the frequency distribution of your variables to detect outliers or anomalies.
- Summary statistics: Basic statistical measures like mean, median, and standard deviation can offer insights into data quality.
- Cross-field validation: Validate the data in one field against another. For example, the state and ZIP code in an address should be consistent with each other.
3. Data auditing tools #
- Data quality software: These tools can automatically scan and flag potential data quality issues.
- Data validation checks: These checks can be implemented in the software and databases you use for data entry.
- Custom scripts: You can write scripts to perform complex checks that you cannot do manually or through data quality software.
4. Visual analytics tools #
- Data visualization: Use tools like Tableau or Power BI to visualize your data, which may help in identifying data quality issues that are not easily noticeable in tabular format.
- Heatmaps: Use heatmaps to find missing or inconsistent data.
5. User feedback #
- Feedback forms: Encourage users to report any errors or anomalies they find in the data.
- Error reporting: Incorporate an error reporting feature in your applications to capture instances where users encounter data quality issues.
6. Periodic audits #
- Schedule regular checks: Make it a part of your operations to check data quality at regular intervals.
- Audit logs: Use audit logs to go back and see when data was changed, who changed it, and what it was before the change.
7. Business rule validation #
- Rule-based checks: For example, if you have a rule that a customer’s age must be between 18 and 99, then you can flag any records that violate this rule.
8. Peer reviews #
- Quality assurance teams: Assign a team to review data entries or the results of data analysis tasks.
- Third-party audits: Sometimes it helps to have an external entity review your data for quality. They bring a fresh perspective and may catch issues you missed.
9. Leverage machine learning #
- Anomaly detection: Machine learning algorithms can be trained to identify outliers or anomalies in your data sets.
- Predictive analysis: Utilize machine learning to predict and flag potential future data quality issues based on historical data.
By combining these methods and continuously monitoring your data, you can successfully identify data quality issues in a timely manner.
This enables you to take corrective actions before these issues escalate into bigger problems that could jeopardize your decision-making processes. Now, let’s explore some steps to improve data management practices to evade from data quality issues.
10 Steps for improving your data management practices #
In the dynamic landscape of data utilization, ensuring that your data is reliable and valuable is paramount.
The journey from raw data to actionable insights necessitates a systematic approach to data management.
Here are the steps:
- Define data quality standards
- Data governance
- Data profiling
- Automate data validation
- Master data management
- Data integration
- Data quality tools
- Regular audits
- Training and awareness
- Continuous improvement
Let’s explore each step in detail.
1. Define data quality standards #
Defining data quality standards means setting clear rules for data accuracy, completeness, and reliability. These guidelines act as quality checkpoints, ensuring that information entered and used meets consistent and dependable criteria.
2. Data governance #
Appoint data stewards, set up data governance frameworks, and document data management processes.
3. Data profiling #
Data profiling involves systematically examining datasets on a regular basis to pinpoint irregularities and anomalies. By scrutinizing data patterns, distributions, and values, organizations can uncover inconsistencies that might otherwise go unnoticed.
This practice aids in identifying outliers, gaps, or inaccuracies that could skew analyses and decisions. Regular data profiling ensures data health and reliability, contributing to more accurate insights and informed actions.
4. Automate data validation #
Automating data validation involves setting up predefined rules that automatically check data accuracy during entry. These rules can flag or reject entries that don’t meet specified criteria, helping prevent errors before they enter the system.
For example, if a rule stipulates that email addresses must include “@” symbols, any entry without one would be flagged. By implementing automated data validation, organizations streamline the process, reduce human errors, and ensure that only accurate and consistent information makes its way into the database, ultimately improving data quality.
5. Master data management #
Master data management involves consolidating and maintaining a single, authoritative source of essential data across an organization. By centralizing master data, such as customer information or product details, businesses ensure consistency and accuracy.
This practice avoids the proliferation of duplicates and conflicting information that can arise from decentralized storage. Ensuring the quality of this master data is vital, as it forms the foundation for various operations and analyses, contributing to better decision-making and improved overall data integrity.
6. Data integration #
Data integration refers to the process of combining information from various sources into a unified and coherent dataset. Robust integration tools play a crucial role in ensuring that data from different systems, applications, or databases is accurately merged.
These tools handle complexities like differing formats, structures, and data types. By achieving seamless data integration, organizations can avoid inconsistencies and maintain data accuracy across the board.
This unified dataset provides a comprehensive view, enabling more comprehensive and precise analyses.
7. Data quality tools #
Data quality tools are valuable assets that aid in identifying and rectifying issues within datasets. These tools use various techniques like data profiling, validation, and cleansing to detect anomalies, duplicates, and inaccuracies.
By investing in such tools, organizations can automate the process of improving data accuracy and consistency. For instance, a data quality tool might identify incomplete customer addresses and suggest corrections.
This proactive approach not only enhances data reliability but also saves time and effort that would otherwise be spent manually identifying and resolving issues.
8. Regular audits #
Regular data audits involve systematically reviewing and evaluating datasets at consistent intervals. These audits ensure ongoing data quality by identifying any emerging issues, inconsistencies, or discrepancies.
By conducting routine checks, organizations can detect problems early on and take corrective actions promptly. For instance, a regular audit might uncover outdated customer contact information and prompt updates.
This proactive approach helps maintain data accuracy and prevents the accumulation of errors that could impact analyses or decision-making in the long run.
9. Training and awareness #
Training and awareness initiatives involve educating employees about the significance of data quality and their individual roles in upholding it. When staff members understand how their data-related actions impact the organization, they are more likely to prioritize accuracy and consistency.
For example, training sessions might emphasize the importance of entering correct customer details.
By fostering a culture of data quality awareness, organizations empower employees to contribute to accurate data, reducing errors at their source and enhancing the overall integrity of the data used for decision-making and analysis.
10. Continuous improvement #
Continuous improvement is a fundamental aspect of data management. Recognizing that data quality is an ongoing endeavor, organizations must consistently monitor and refine their practices.
This involves regularly assessing data quality standards, reviewing data integration processes, and updating data governance frameworks. By embracing this iterative approach, organizations ensure that their data management practices stay up-to-date with evolving needs and technologies.
Continuous improvement safeguards against complacency, allowing businesses to adapt and enhance their data quality practices over time, resulting in more reliable and valuable data for informed decision-making. By embracing these steps, businesses can navigate the evolving data landscape with confidence, efficiency, and accuracy.
Conclusion #
Data quality issues pose significant challenges in today’s data-rich landscape, affecting everything from day-to-day operations to long-term strategic decisions. With the increasing complexities and volumes of data being managed, the need for robust, reliable data has never been more critical.
However, the good news is that these challenges are not insurmountable. By understanding the types of data quality problems, their root causes, and their implications, organizations can take proactive steps to mitigate them.
So, while the journey towards impeccable data quality is ongoing, armed with the right knowledge and tools, businesses can significantly improve their data quality, paving the way for better decision-making, increased operational efficiency, and a stronger competitive edge.
How to fix your data quality issues: Related reads #
- Resolving Data Quality Issues in the Biggest Markets
- Data Quality Explained: Causes, Detection, and Fixes
- How to Improve Data Quality in 12 Actionable Steps?
- Data Quality Fundamentals: Why It Matters in 2023!
- Hidden Costs of Bad Data Explained: 12 Ways to Tackle Them
- 11 Proven Strategies for Achieving Enterprise-Scale Data Reliability
- Data Lineage & Data Observability: Why Are They Important?
Share this article