Data Mesh Vs. Data Lake: Key Differences & Use Cases for 2025
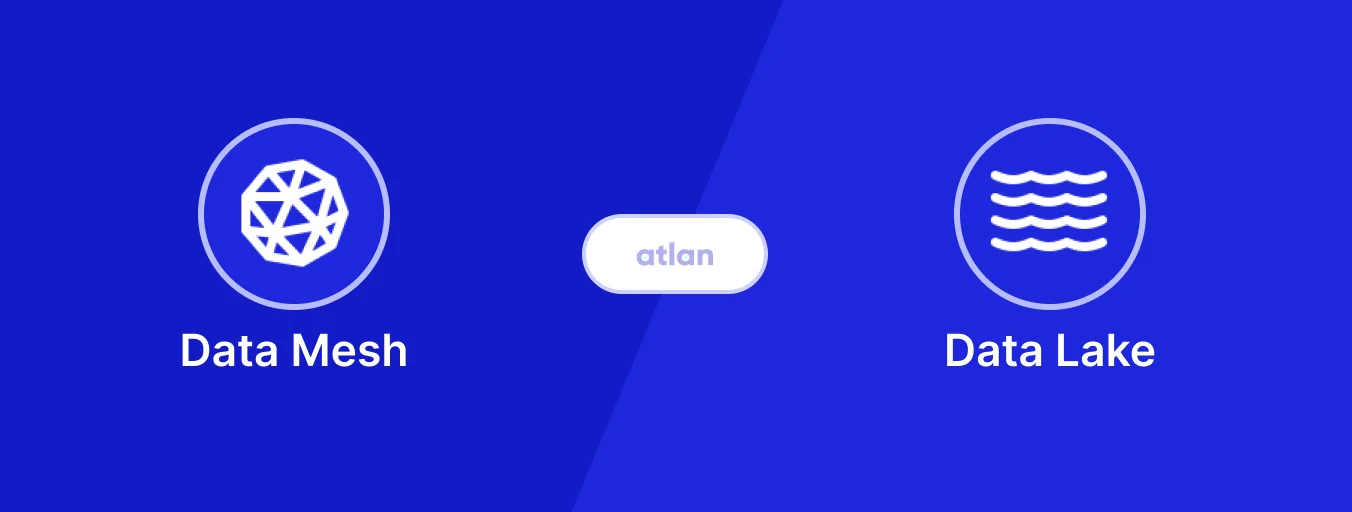
Share this article
Data Mesh and Data Lake are distinct approaches to managing and organizing data within an organization.
- A data lake centralizes raw, unstructured data in a single repository for flexible storage and analysis.
- A data mesh decentralizes data ownership, aligning it with domain teams for better scalability and governance.
See How Atlan Simplifies Data Governance – Start Product Tour
While data lakes are ideal for storing massive datasets, data meshes optimize data for real-time analytics and decision-making.
Both architectures support modern data strategies but serve different organizational needs.
Understanding their differences helps businesses choose the right solution for scalable and efficient data management.
Table of contents #
- Data mesh Vs Data Lake
- Data mesh and data lake: The fundamentals
- Data mesh vs. data lake: Why you shouldn’t be comparing them
- Data mesh and data lake: When do you choose to roll out either or both of them?
- How Atlan Supports Data Mesh Concepts
- Bottom line
- FAQs about Data Mesh vs Data Lake
- Data mesh vs. Data lake: Related reads
Data mesh Vs Data Lake #
The main difference between a data mesh and a data lake is that a data mesh is a design strategy for enterprise data platform architecture. Meanwhile, a data lake is a central repository that stores data — structured and unstructured — in a raw format.
The Ultimate Guide to Data Mesh - Learn all about scoping, planning, and building a data mesh 👉 Download now
Here, we will further explore the concepts of data mesh and data lake by understanding their specific use cases and maturity stages at which organizations may choose to deploy them into their data platform.
Data mesh and data lake: The fundamentals #
Let’s begin by quickly reviewing the fundamentals of data mesh and data lake.
What is a data mesh? #
Data mesh is a decentralized sociotechnical approach to share, access, and manage analytical data in complex and large-scale environments—within or across organizations, as defined in Data Mesh: Delivering Data-Driven Value at Scale.
It combines the techniques of distributed domain-driven architecture, self-serve platform design, and product thinking with data to develop an enterprise data platform architecture.
The term was coined by Zhamak Dehghani, a former principal technology consultant at ThoughtWorks, in 2019.
Read more → Everything you need to know about data mesh
Key principles of a data mesh architecture. Source: Data Mesh by Zhamak Dehghani, O'Reilly Media, Inc
Example use cases: Data mesh for sales and marketing #
Both sales and marketing departments are domains and, as such, have domain owners.
These teams are responsible for collecting, storing, and managing their data, rather than a central data team. So, if someone from the marketing team wants to access sales data, they work with the sales team directly.
As a result, business teams have complete control and ownership of their campaigns. They don’t have to wait for the IT department to help them find historical data on customers or campaign results.
What is a data lake? #
A data lake is a central location for storing all kinds of data in raw and processed forms, collected from various sources.
Data lakes are simple, scalable, and cost-effective for storing large volumes of data. So, many organizations have invested in a central data lake and a data team responsible for managing it to get rid of data silos, capture all kinds of data, and drive business decision-making.
The term was popularized by James Dixon — founder and CTO of business intelligence software company Pentaho — in 2010.
Read more → Everything you need to know about data lakes
Example use cases: Data lake for big data storage #
From oil and gas to life sciences, all data-heavy industries can use data lakes to store and process big data at scale.
For instance, an average oil and gas platform generates at least 2 TB daily. When that data is stored at a central, accessible location, data scientists and geologists can use it to optimize directional drilling, minimize unplanned downtime, improve safety, and more.
Data mesh vs. data lake: Why you shouldn’t be comparing them #
Since a data lake is a centralized approach to managing data, and the data mesh is a decentralized design for enterprise data architecture, people tend to compare the two concepts.
However, a better way is to think about how they synergize. Let’s explore this possibility by looking at the following:
- The shortcomings of a centralized approach to data architecture design
- The promise of a decentralized data architecture
- The merits of a design where the data mesh and data lake fit together
The shortcomings of a centralized data architecture #
Currently, most data-driven organizations have set up a centralized, domain-agnostic data platform overseen by a central team of engineers.
The resulting data repository — usually a data lake — houses all organizational data and is treated as the single source of truth for the organization. For instance, Adidas used to rely on such an architecture where:
- The data lake (AWS S3) centralized the storage of all kinds of data
- A central data team was responsible for all the heavy lifting involved in ingesting data into the lake
Such an approach isn’t scalable, especially for large organizations like Adidas with several business domains and analytics use cases.
Here’s how Zhamak Dehghani describes this conundrum:
“The hyper-specialized data platform engineers need to provide data for a diverse set of needs, operational or analytical, without a clear understanding of the application of the data and access to the consuming domain’s experts.”
Data mesh: Key differentiating characteristics. Source: Data Mesh by Zhamak Dehghani, O'Reilly Media, Inc
The promise of a decentralized data infrastructure approach #
The data mesh reimagines how you design your data architecture to be domain-oriented decentralization for analytical and operational data.
Each data domain is owned end-to-end by the domain team, rather than a centralized data team. According to Dehghani:
“For a distributed data platform to be successful, domain data teams must apply product thinking with similar rigor to the datasets that they provide; considering their data assets as their products and the rest of the organization’s data scientists, ML, and data engineers as their customers.”
So, the domains are responsible for owning their data and implementing data pipelines that clean, prepare, aggregate, and send data to various applications.
The overall data mesh design would have several data products, just like a microservice architecture. These data products are interconnected and interoperable, which ensures that there aren’t any data silos.
Data mesh: Key principles and their benefits. Source: Data Mesh by Zhamak Dehghani, O'Reilly Media, Inc
Introduction to Data Mesh with Zhamak Dehghani
Why does the data mesh architecture need a data lake? #
Let’s start by understanding the three building blocks of the data mesh architecture:
- Distributed data products oriented around domains
- Independent cross-functional teams, with data engineers and data product owners, responsible for managing these products
- A common data infrastructure as a platform to host, prep, and serve all data assets
The first point talks about how each domain is responsible for its own data products. To be able to do that, the individual domains still need to house their data in a cost-effective, cloud-based, scalable platform like a data lake.
For instance, when JP Morgan Chase implemented the data mesh design for its data ecosystem, each data product had a product-specific data lake. The data lakes were interconnected and exchanged data using a suite of AWS cloud services for cataloging, querying, and data sharing.
You can use the various data lake tools from AWS, Oracle, Databricks, or Azure to set up the overall data mesh infrastructure.
Data mesh and data lake: When do you choose to roll out either or both of them? #
Choosing the data lake architecture #
A centralized, monolithic architecture is good enough if you:
- Are a small organization without numerous business domains
- Don’t have several engineering teams
- Can manage your analytical and operational use cases smoothly with a monolithic system
Choosing the data mesh architecture #
The data mesh isn’t for everyone. You must have the following:
- Data-oriented strategy: You consider AI, ML, and analytics to be your “strategic differentiator”
- Data technology: You already have the ability and desire to build data-driven technology infrastructure and use it as a competitive advantage
- Modern engineering: You already inculcate modern engineering principles of CI/CD, DevOps, and DataOps, and have a modern data stack in place
- Domain-oriented organization: Your organization design is based on your business domains
- Organizational complexity: Existing data warehouse or lake solutions have become blockers in your quest to extract value from data at scale
- Data-mature teams: Each domain has technical teams capable of maintaining the necessary data infrastructure and domain experts well-versed in data-driven decision-making
How Atlan Supports Data Mesh Concepts #
Atlan helps organizations implement data mesh principles by enabling domain teams to create and manage data products that can be easily discovered and consumed by other teams.
Data products in Atlan are scored based on data mesh principles such as discoverability, interoperability, and trust, providing organizations with insights into their data mesh maturity.
Atlan’s automated lineage tracking and metadata management capabilities further support data mesh implementation by providing a comprehensive understanding of data flows and dependencies across domains.
How Autodesk Activates Their Data Mesh with Snowflake and Atlan #
- Autodesk, a global leader in design and engineering software and services, created a modern data platform to better support their colleagues’ business intelligence needs
- Contending with a massive increase in data to ingest, and demand from consumers, Autodesk’s team began executing a data mesh strategy, allowing any team at Autodesk to build and own data products
- Using Atlan, 60 domain teams now have full visibility into the consumption of their data products, and Autodesk’s data consumers have a self-service interface to discover, understand, and trust these data products
Book your personalized demo today to find out how Atlan supports data mesh concepts and how it can benefit your organization.
Bottom line #
The main difference between a data mesh and a data lake is that a data mesh is a design strategy for enterprise data platform architecture. Meanwhile, a data lake is a central repository that stores data — structured and unstructured — in a raw format.
A key takeaway from this article is:
- The data mesh is a cultural and technological shift in thinking
- The data lake - other than being a fundamental component of data stacks - is a means of implementing the many elements constituting the mesh infrastructure
If you’re considering the data mesh approach, your focus should be redesigning how data flows through your organization. Tooling — data lakes, warehouses, pipelines — should be a secondary concern, i.e., “an implementation detail.”
FAQs about Data Mesh vs Data Lake #
1. What is the difference between a data mesh and a data lake? #
A data mesh is a decentralized data architecture approach focusing on domain-oriented ownership and self-serve infrastructure, while a data lake is a centralized repository that stores structured and unstructured data at scale.
2. How does a data mesh architecture work compared to a data lake? #
Data mesh operates by assigning data ownership to individual domains, ensuring they manage and govern their data as a product. In contrast, data lakes provide a single storage solution, which organizations use for batch and real-time data processing.
3. What are the benefits of a data mesh vs a data lake? #
Data mesh improves scalability, data quality, and governance by decentralizing data management. Data lakes are cost-effective for storing large datasets but can suffer from data governance challenges and “data swamp” risks.
4. Can a data mesh and data lake be used together? #
Yes, a data lake can serve as the foundational storage layer in a data mesh ecosystem, providing scalable storage while enabling decentralized data ownership and governance.
5. How does data quality differ in a data mesh vs a data lake? #
Data mesh promotes high-quality data by treating it as a product, with each domain responsible for its governance and quality. Data lakes, without proper governance, can degrade in quality due to lack of ownership and standards.
6. What are the challenges of implementing a data mesh vs a data lake? #
Implementing a data mesh requires cultural and operational shifts, including clear domain ownership and governance. Data lakes, on the other hand, face challenges in maintaining data quality and avoiding ungoverned growth.
Data mesh vs. Data lake: Related reads #
- What Is a Data Lake? Definition, Architecture, and Solutions
- What is Data Mesh?: Examples, Case Studies, and Use Cases
- Data Catalog: Does Your Business Really Need One?
- Data Mesh Setup and Implementation - An Ultimate Guide
- Data Mesh Principles — 4 Core Pillars & Logical Architecture
- Snowflake Data Mesh: Step-by-Step Setup Guide
- Data Mesh and Data Lake: Understanding Use Cases & Reasons to Deploy
- Data Fabric vs Data Mesh: What are the Key Differences?
- DataOps:: Guide, Framework & 9 Key Principles
- Data Silos 101 Definition, Challenges and Solutions
- Data Mesh Architecture: Why It Matters to You in 2025?
- What Is a Data Warehouse: Concept, Architecture & Example
- Modern Data Team 101: A Roster of Diverse Talent
- Modern Data Stack: Components, Architecture & Tools
- What is Data Driven Decision Making & Why Does It Matter?
Share this article