8 Data Governance Standards Your Data Assets Need Now
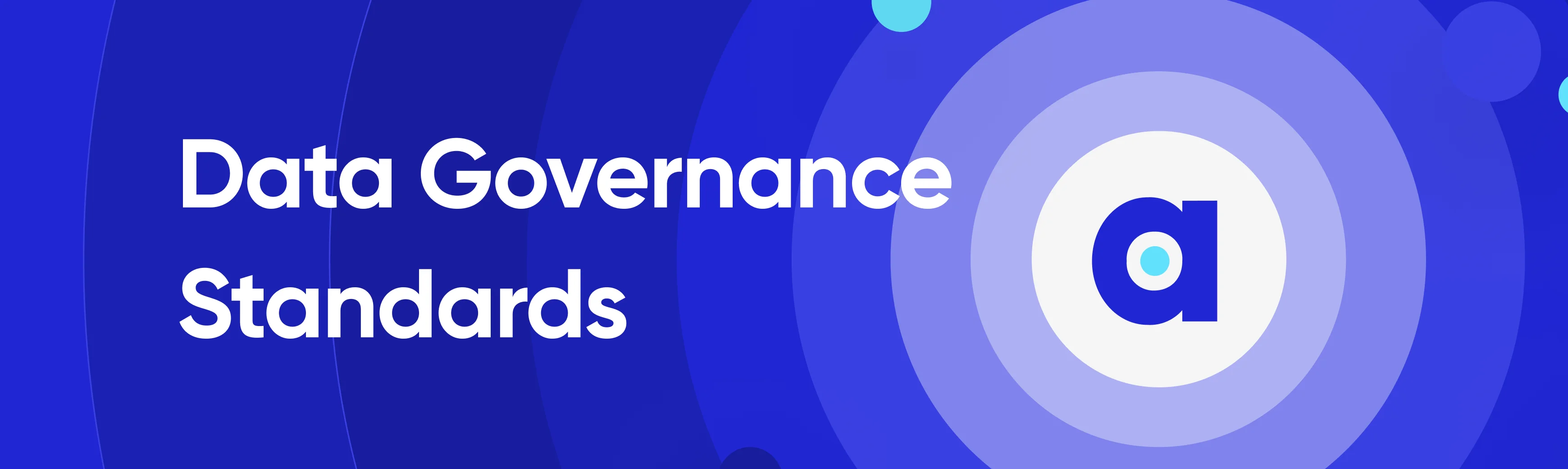
Share this article
Data governance standards are guidelines and procedures that an organization puts in place to manage and ensure the quality, availability, usability, integrity, and security of its data assets.
These standards are a system for defining who within an organization can take action on specific data, when these actions can be taken, and the scope of these actions.
In this article, we will learn the eight fundamental standards that shape effective data governance practices such as data quality, integration, security, metadata and more.
Let’s dive in!
Table of contents #
- What are the key data governance standards?
- Data governance standard examples: Understanding them better
- How to create data governance standards: A step-by-step guide
- Summary
- Data governance standards: Related reads
What are the key data governance standards? #
Data governance standards establish a framework and guidelines for managing and protecting data assets effectively. They promote data quality, compliance, collaboration, and enable organizations to derive maximum value from their data while minimizing risks associated with data management.
In this section, we will learn more about the key standards in data governance:
- Data quality standards
- Data integration standards
- Data security and privacy standards
- Data accessibility standards
- Data lifecycle management standards
- Data compliance standards
- Data ownership and stewardship standards
- Metadata management standards
Let’s dive into each of these data governance standards one by one:
1. Data quality #
The data quality standard says that data must be accurate, complete, timely, and consistent. It should be fit for its intended uses in operations, decision-making, and planning.
2. Data integration #
Data from various silos should be able to be combined and used together. This often involves the use of common data models, APIs, and other tools to integrate disparate data sources.
3. Data security and privacy #
The organization needs to ensure the confidentiality and integrity of data, comply with privacy regulations, and protect against unauthorized access and data breaches.
4. Data accessibility #
Data should be accessible to those who need it, with controls to ensure only authorized individuals can access specific data. Data should also be understandable and usable, with clear definitions, labels, and documentation.
5. Data lifecycle management #
Data should be managed throughout its lifecycle, from creation and collection, through use and archiving, to eventual destruction.
6. Data compliance #
This involves complying with relevant industry and government regulations concerning data. This could include regulations on data privacy, data retention, and data use.
7. Data ownership and stewardship #
Clear responsibilities and accountabilities should be assigned for data management. This typically involves assigning data owners who are responsible for specific data assets, and data stewards who oversee the data’s quality and proper usage.
8. Metadata management #
Metadata should be properly managed. This includes descriptive metadata (information about the content of data), structural metadata (information about how data is organized), and administrative metadata (information about the source and management of the data).
These standards form the basis of a data governance program. The standards should be clearly communicated and enforced across the organization, with regular audits and reviews to ensure compliance. Training should also be provided to ensure all staff understand the importance of data governance and their role in it.
Data governance standards examples: Understanding them better #
Now that we know the eight core data governance standards, let us understand them in more detail with examples:
1. Data quality #
Ensuring data quality requires creating data quality standards that include checks for accuracy, completeness, timeliness, and consistency.
Example:
All customer data might be required to have a verified email address and phone number (completeness). Another requirement might be to ensure data entries are not more than 30 days old (timeliness), are consistent with entries in other systems (consistency), and are checked against external databases for accuracy.
2. Data integration #
Data from various sources (like marketing, sales, and customer service databases) should be easily combined for cross-functional analysis. This could involve creating a common data model across the organization and using ETL (Extract, Transform, Load) tools.
Example:
All teams might agree to use a standard format for recording customer interactions, so that data from different departments can be combined into a single customer profile.
3. Data security and privacy #
Security measures might include encryption, access controls, and regular audits.
Example:
Personal customer data might be encrypted both at rest and in transit, and access might be controlled with a role-based access control system. Additionally, privacy policies would be put in place in accordance with regulations such as GDPR or LGPD in specific countries.
4. Data accessibility #
Accessibility standards might dictate that all data be stored in a central location that is accessible to all relevant parties, with appropriate access controls.
Example:
All data might be stored in a cloud-based data warehouse that can be accessed by any team member with the necessary permissions.
5. Data lifecycle management #
Data lifecycle management might involve policies for how long data is stored when it is archived, and when it is destroyed.
Example:
Customer data might be retained for 7 years after the last interaction, then archived for a further 3 years, and then destroyed.
6. Data compliance #
Compliance standards would be based on relevant laws and regulations.
Example:
There might be a standard for obtaining customer consent before collecting personal data, in accordance with privacy laws.
7. Data ownership and stewardship #
Ownership and stewardship policies might assign specific individuals or teams with responsibility for managing specific data assets.
Example:
The marketing team might be assigned as the owner of the customer demographic data, responsible for ensuring its quality. On the other hand, a data steward might be responsible for overseeing the use and protection of the data across the organization.
8. Metadata management #
Metadata management standards might require that all data be accompanied by descriptive metadata (like data source, date of collection, and the individual who collected it), structural metadata (like the data format and schema), and administrative metadata (like the data ownership and usage restrictions).
Example:
A dataset containing customer survey responses might include metadata on the survey date, the survey creator, the format of the survey responses, and the team responsible for the survey data.
These examples illustrate some of the ways in which data governance standards could be applied. The specific standards will, of course, depend on the nature of your organization and its data.
How to create data governance standards: A step-by-step guide #
Setting data governance standards is an extensive process that involves understanding the organization’s data landscape and defining clear policies, procedures, roles, and responsibilities.
Here is a general step-by-step guide:
- Assess the current state
- Define the vision
- Establish a data governance council
- Define roles and responsibilities
- Define data governance standards
- Create policies and procedures
- Implement data governance tools
- Communicate and train
Let’s discuss these in detail:
Step 1: Assess the current state #
Understand the existing data landscape. This includes an audit of the data assets, how data is used, and existing data management practices. Also, understand the business strategy and goals, compliance requirements, and the data challenges that the organization is currently facing.
Step 2: Define the vision #
Clearly define what you hope to achieve with your data governance program. This could be improving data quality, meeting regulatory requirements, improving data security, or enabling better decision-making.
Step 3: Establish a data governance council #
This should be a cross-functional team that will drive the data governance initiative. This team should include stakeholders from various parts of the organization who have a vested interest in effective data governance. The council will be responsible for creating the data governance strategy, setting standards, and overseeing implementation.
Step 4: Define roles and responsibilities #
This includes defining roles like data owners, data stewards, data custodians, and data users, and their responsibilities. Data owners are typically responsible for the quality and use of specific data assets, while data stewards ensure that data governance policies are adhered to.
Step 5: Define data governance standards #
Based on your understanding of the data landscape and business goals, define clear standards for data quality, data security, data privacy, data lifecycle management, etc. The standards should be practical, achievable, and aligned with the business goals.
Step 6: Create policies and procedures #
Translate the standards into clear policies and procedures. This includes procedures for data access, data quality checks, data classification, data retention, and so on.
Step 7: Implement data governance tools #
Consider implementing tools that can help with data governance. This can include data catalogs, data quality tools, data lineage tools, etc. These tools can help automate many aspects of data governance and ensure compliance with standards.
Step 8: Communicate and train #
Once the standards, policies, and procedures are defined, communicate them to all relevant parties. Provide training to ensure everyone understands their roles and responsibilities
Summary #
Data governance standards are crucial for managing the quality, availability, usability, integrity, and security of the organization’s data assets. These standards guide who can take action on specific data, when, and the extent of the actions.
By implementing robust data governance standards, organizations can optimize their data management practices, mitigate risks, enhance decision-making capabilities, and gain a competitive edge in the data-driven landscape.
If you are evaluating and looking to deploy best-in-class data access governance for your data ecosystem without compromising on data democratization, do give Atlan a spin.
Data governance standards: Related reads #
- What is Data Governance? Its Importance, Principles & How to Get Started?
- Key Objectives of Data Governance: How Should You Think About Them?
- Data Governance Framework Examples, Templates, Standards, Best Practices & How to Create One?
- Data Governance and Compliance: Act of Checks & Balances
- How to implement data governance? Steps, Prerequisites, Essential Factors & Business Case
- How to Improve Data Governance? Steps, Tips & Template
- 7 Steps to Simplify Data Governance for Your Entire Organization
- Snowflake Data Governance Features, Frameworks & Best Practices
- Automated Data Governance: How Does It Help You Manage Access, Security & More at Scale?
- Enterprise Data Governance Basics, Strategy, Key Challenges, Benefits & Best Practices
- Data Governance in Manufacturing: Steps, Challenges, and Practical Examples
- Data Governance in Retail: Best Practices, Challenges, and Viable Solutions
- Data Governance in Insurance: Why is it Important and How it Drives Positive Business Outcomes
Share this article