Data Governance for AI: Challenges & Best Practices
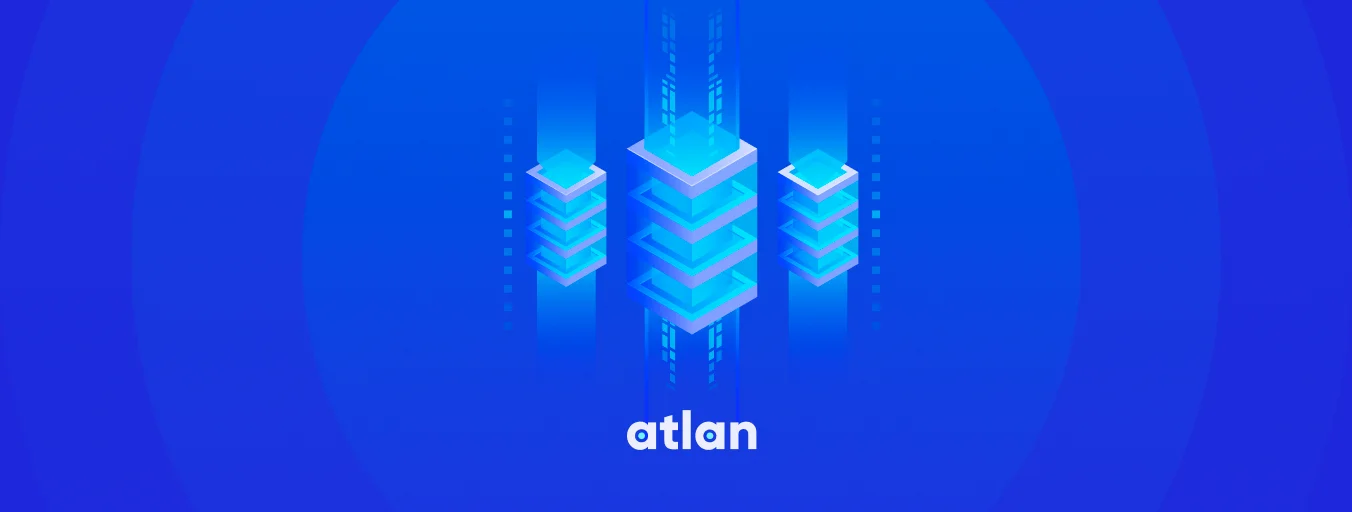
Share this article
AI is a powerful tool for organizations, but AI systems come with unique challenges. The large amount of data used by AI systems raises complicated data governance questions. In this article, we will look at some of the challenges of data governance for AI systems and consider possible solutions.
Want to make data governance a business priority? We can help you craft a plan that’s too good to ignore! 👉 Talk to us
Table of contents #
Challenges of AI governance #
AI tools are rapidly impacting the business landscape. According to a 2022 survey by McKinsey, AI adoption has more than doubled in the last five years.
The large amount of data used to train AI systems raises complex questions of data lineage, trust, and privacy. Sensitive, private information needs to be handled properly, especially as the regulations in Europe and America are shifting. AI systems need to be carefully designed so that their data usage aligns with user safety and the law.
This means that AI needs its own data governance. Let’s look in more detail at exactly what issues an AI data governance system needs to handle.
What makes data governance for AI challenging? #
A few factors make data governance for AI a particularly complicated problem.
- Hidden security risks: When a system is being trained on hundreds of terabytes of data, it’s easy for some entries containing sensitive information to find their way in. That information will be trained into the AI’s neural network. That makes it potentially accessible to users without anyone even recognizing the vulnerability.
- Irregular user interfaces: With AI, users don’t select from menus - they prompt using natural language. This flexibility means unexpected inputs are possible, such as a user mistakenly inputting private or confidential information. Any records of this input are a severe risk! Added flexibility means that UI requires extra safeguards and audits.
- Unexplainability: The algorithms produced by AI training aren’t explicitly designed. The mechanics of an AI system are not easy to unpack. The opacity of AI systems can make them difficult to trust. Efforts towards explainability help build trust in AI systems.
- Expensive testing: Since inputs are flexible, AI system outputs can be chaotic. Imagine the chatbot you trained gives incorrect answers for certain phrasings of a common question. Testing for these failures is prohibitively expensive, so AI systems require consistent monitoring and auditing to stay reliable.
The data governance issues around AI are challenging, but there are potential solutions. Let’s look at some of the core ideas in detail.
Data Governance for AI #
AI requires data governance, which handles the security of its data, the safety of user interfaces, and testing standards to maintain trust. Let’s look at some ideas for addressing each of these issues.
Data Security #
AI systems are driven by the data they are trained on. Thus, having a solid hold on the security and access permissions for training data is paramount. If any sensitive data ends up as part of an AI system, there is a potential for leaks. This means step one of AI governance is baseline strong data governance.
Strong data governance means committing to an organizational idea of data stewardship. Data stewardship means everyone who works with data is responsible for its security and accuracy. An established stewardship framework allows data to be shared with trust.
Trust is the key that allows data to be shared, for example, with an AI framework. The idea of data stewardship is the first step to building a trusted data-sharing framework. Such a framework requires an organizational commitment and technical investment.
For example, payment processing company North American Bancard used Atlan’s metadata layers to flag and identify sensitive data. Such metadata labeling may soon be an industry standard. This labeling allows an organization to be transparent about the data it uses for model training, thereby building the trust necessary for security.
Interface safety #
The power of AI systems is their ability to handle and answer a wide variety of questions. But that flexibility opens up new risks.
Users can mistakenly reveal sensitive information to the model, which can end up in logs. Or worse, users can perform malicious prompt injection to get the model to disclose private information.
If you build an AI system, you need to be sure that the data coming in and out is as safe as the data used to train it. Maintaining security means scrubbing sensitive data from input logs and rejecting inputs that can compromise security. From a design perspective, it also means minimizing the use cases that may bring sensitive information into the system.
Tracking these risks means building a management system that can help you discover problems with your data. For example, the search platform Elastic used Atlan to help flag breakdowns in their data pipeline. Reducing discovery time for potential issues helps ensure that AI systems are safe at all times.
Testing standards #
According to Google’s whitepaper on AI governance, the following are important factors in the transparency and accountability of AI systems: Flagging capabilities, output contesting, and auditing.
Flagging capabilities are a way for users to indicate that an output of the AI system is somehow concerning, like flagging a post on social media. For example, if a prompt produces a response containing sensitive information, the user can flag that prompt and respond. Such flagging systems are critical for AI, where case-by-case behavior may be unexpected.
Output contesting is a system for overriding the output an AI gives. For example, if a coding assistant suggests some broken code, output contesting is when the developer replaces it with working code. Output contesting is critical to prevent an error the AI system produces from proliferating unhindered.
All of this testing and maintenance comes down to consistent audits of AI systems, which requires building a governance framework to track the structure and lineage of the data used. For example, API platform Postman uses Atlan to track their transformation pipelines, ensuring that they clearly understand the connections between data and dashboards.
Conclusion #
While AI is powerful, we have seen that it comes with unique data governance issues. Atlan’s metadata tracking, enhanced data discoverability, and automated data lineage can help you handle the data governance of your AI system. Request a personalized Atlan demo today and see if it is right for you.
Data Governance for AI: Related Reads #
- AI Data Catalog: Its Everything You Hoped For & More
- 8 AI-Powered Data Catalog Workflows For Power Users
- AI Data Governance: Why Is It A Compelling Possibility?
- What is Data Governance? It’s Importance, Principles & How to Get Started?
- Enterprise Data Governance — Basics, Strategy, Key Challenges, Benefits & Best Practices
- Automated Data Governance: How Does It Help You Manage Access, Security & More at Scale?
- 6 Commonly Referenced Data Governance Frameworks in 2024
- 8 Best Practices for a Robust Data Governance Program
- Data Governance Roles and Responsibilities: The complete list
- 5 Popular Data Governance Certification & Training in 2024
- 8 Best Data Governance Books Every Data Practitioner Should Read in 2024
- Snowflake Data Governance — Features, Frameworks & Best Practices
- What is Active Metadata? — Definition, Characteristics, Example & Use Cases
Share this article