A Deep Dive into the Data Value Curve: Stages and Templates
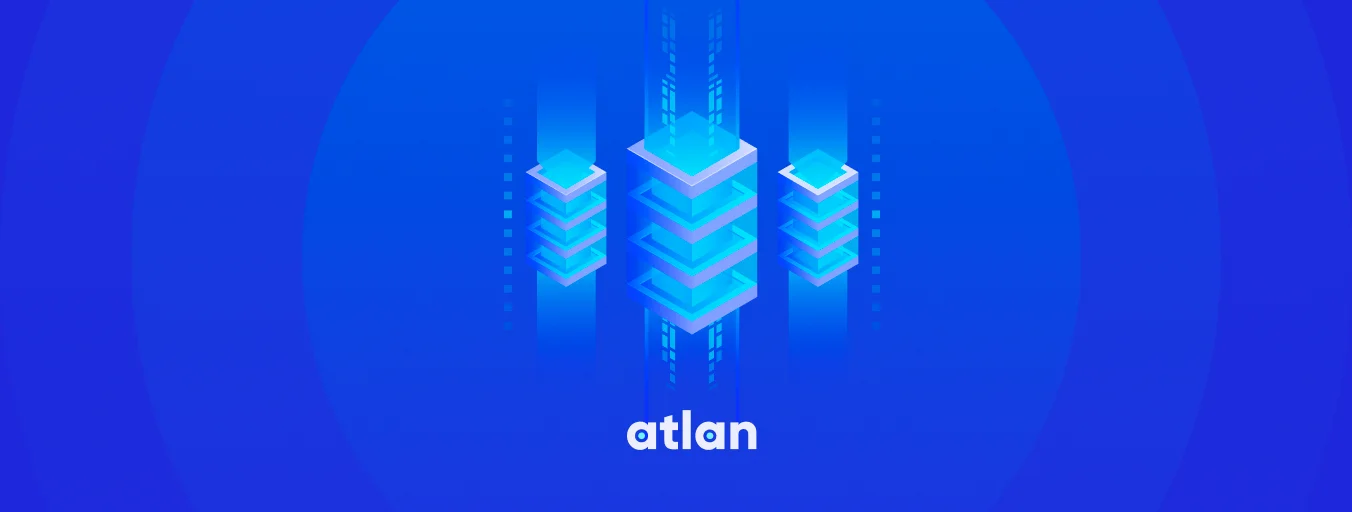
Share this article
"The Data Value Curve is a strategic framework used for evaluating and optimizing the utility of data within an organization. "
Data is often considered the new oil—a resource that, when refined and analyzed correctly, can unlock immense value. Yet, not all data is created equal. Companies collect terabytes of data, but only a fraction of it turns into actionable insights or strategic assets. This is where understanding the data value curve can make a significant difference.
By comprehending the intricacies of this curve, you can unlock the latent potential in your data, transforming it from mere raw information into a cornerstone of your business strategy.
In this comprehensive guide, we’ll take a deep dive into the six key stages of the data value curve, explore its advantages and disadvantages, and provide a step-by-step template to help you create your own data value roadmap.
Let us dive in!
Table of contents #
- What is the data value curve?
- 6 Key stages of the data value curve
- Data value curve: Advantages and disadvantages
- Data value curve template: How to generate one?
- 4 Tools to generate data value curve
- Summary
- Related reads
What is the data value curve? #
The value curve is a graphic depiction of a company’s relative performance across its industry’s factors of competition. The goal is to create a unique curve that offers unparalleled value to customers.
A data value curve provides a visual representation of the increasing value an organization can extract from its data by advancing its analytical capabilities. As an organization progresses from basic data collection to sophisticated predictive analytics and proactive decision-making, the value derived from the data tends to increase.
Key characteristics of the data value curve:
- Factors of competition
- Level of offering
- Red oceans vs. Blue oceans
- Four actions framework
Let us understand each of them in detail:
1. Factors of competition #
- The horizontal axis of the value curve represents the key factors of competition within the industry or market. These factors can be anything that an industry competes on or what customers value, such as price, quality, speed of service, features, etc.
- For your product, these could be features, benefits, or other attributes that customers find important.
- Your market research and competitor analysis would have already given you insights into these factors.
2. Level of offering #
- The vertical axis depicts the level of offering that a company delivers for each factor, ranging from high to low.
- By plotting the level of offering for each competitive factor, you visualize how your product differentiates from the competitors.
3. Red oceans vs. Blue oceans #
- Red oceans: Represent existing market space where industry boundaries are defined, and companies compete fiercely, often on similar factors of competition. This makes the waters bloody, hence the term “red.”
- Blue oceans: Denote new market spaces, untapped and uncontested, where you can create unique value propositions and make competition irrelevant. This is what you’re aiming for with your new product. If your Value Curve is significantly different from competitors, you might be on to a “blue ocean” of opportunity.
4. Four actions framework #
This is a tool associated with the value curve to help you disrupt the market:
- Eliminate: Which of the factors that the industry takes for granted can be eliminated? This can reduce costs and differentiate your offering.
- Reduce: Which factors can be reduced below the industry standard? This can further reduce costs and avoid unnecessary competition.
- Raise: Which factors can be raised above the industry’s standard? This helps in adding unique value.
- Create: What factors can be created that the industry has never offered? This can lead to a breakthrough in value.
By understanding these aspects and plotting your product against competitors on the Value Curve, you can validate whether you’re indeed offering something unique and filling a gap in the market.
6 key stages of the data value curve #
The journey from raw data to impactful business decisions isn’t linear or immediate; it’s a structured process that can best be described through the data value curve.
In this section, we will delve into the six key stages of the data value curve, each representing a significant milestone in your data transformation journey.
These stages help you navigate from the point of initial data collection, through various layers of processing and analysis, to finally utilizing the data in a way that generates true business value.
- Data collection
- Descriptive analytics
- Diagnostic analytics
- Predictive analytics
- Prescriptive analytics
- Proactive decision making
Let us understand each of them in detail:
1. Data collection #
- Before any analysis can begin, raw data must be collected. This stage is foundational to all the subsequent stages and is about capturing as much relevant data as possible from various sources, which might include sensors, user interactions, databases, external sources, and more.
- The initial stage, where the focus is on gathering data. The value at this stage is relatively low since the data is raw and unprocessed.
- Significance: Even though the data is raw and hasn’t been processed or analyzed, it’s the bedrock of the data journey. Without an efficient and comprehensive data collection strategy, the following stages would be compromised.
2. Descriptive analytics #
- Descriptive analytics summarizes historical data to identify patterns and trends. It essentially answers the question: “What has happened?”. Common outputs in this phase include summaries, charts, graphs, and tables.
- At this stage, data is used to understand past events. Tools like dashboards and reports that provide insights into what has already happened fall under this category.
- Significance: This form of analytics allows businesses to learn from past behaviors and understand how they might influence potential future outcomes. It’s the first step in data-driven decision making.
3. Diagnostic analytics #
- Diagnostic analytics delves deeper into data to understand the root causes of observed events. It answers the question: “Why did something happen?”. Techniques such as data discovery, data mining, and correlations are often used.
- Here, data is analyzed to understand the reasons behind past events. This could involve more advanced techniques to find correlations or patterns in historical data.
- Significance: By understanding the causes behind events or behaviors, organizations can make informed decisions to rectify issues or harness positive triggers.
4. Predictive analytics #
- Predictive analytics focuses on forecasting future trends or outcomes based on historical data. It employs statistical algorithms and machine learning techniques, answering the question: “What is likely to happen in the future?”.
- Moving up the value curve, organizations start to use data to forecast future trends. Techniques like machine learning can be employed to predict future events based on historical data.
- Significance: Armed with predictive insights, businesses can be better prepared for future events, anticipate user needs, or identify potential risks.
5. Prescriptive analytics #
- Prescriptive analytics goes beyond predicting future outcomes by suggesting various courses of action and the potential outcomes of each decision. It’s about answering the question: “What should we do?”. Optimization and simulation algorithms play a crucial role here.
- At the peak stage, not only does the organization predict future events, but it also uses data to prescribe specific actions to achieve desired outcomes. It involves complex algorithms and simulations to recommend the best course of action.
- Significance: By understanding the possible outcomes of different decisions, organizations can choose the course of action that aligns best with their objectives, mitigating risks or leveraging opportunities more effectively.
6. Proactive decision making #
- This is the most advanced stage where decisions are made in real-time based on data, often without human intervention. Through technologies like artificial intelligence (AI) and real-time analytics, systems can instantly respond to changing data inputs.
- Some models of the data value curve include this as the pinnacle, where organizations use data in real-time to make immediate decisions, often automated, that can adapt to changing situations seamlessly. explain each point in detail
- Significance: Proactive decision-making allows for a level of agility and responsiveness that can be a significant competitive advantage. In environments where data changes rapidly, such as financial trading or certain types of manufacturing, the ability to make real-time decisions can be critical.
The data value curve isn’t just a progression of technical capabilities; it’s a roadmap for businesses to extract increasing value from their data. As organizations move along this curve, their decision-making processes become more informed, nuanced, and agile, giving them a competitive edge in today’s data-driven landscape.
Data value curve: Advantages and disadvantages #
The data value curve serves as a roadmap for organizations to progress through different stages of data maturity, leveraging increasing value from their data assets.
However, like any strategic tool or framework, it has its advantages and disadvantages.
Advantages of the data value curve #
- Roadmap for progression
- The data value curve provides a clear sequence of stages, guiding organizations on their data analytics journey. It serves as a blueprint for increasing data maturity and sophistication.
- Organizations can easily identify where they currently stand and what the next steps are in their data analytics journey.
- Informed investments
- By understanding where they are on the curve, organizations can prioritize investments in data analytics tools, skills, and technologies accordingly.
- This ensures resources are allocated efficiently and yields a better return on investment.
- Better decision making
- As organizations move up the curve, the quality and depth of insights derived from data analytics improve, leading to more informed decisions.
- This translates to operational efficiencies, improved customer experiences, and innovative strategies.
- Competitive advantage
- Organizations that progress faster and further along the Data Value Curve can achieve a competitive advantage by leveraging insights that others can’t.
- This can result in capturing new markets, better customer retention, and increased profitability.
Disadvantages of the data value curve #
- Overemphasis on progression
- There might be undue pressure to keep moving up the curve, leading organizations to make investments they’re not ready for.
- This can lead to wastage of resources, misguided strategies, and failed initiatives.
- Not One-Size-Fits-All
- While the curve provides a generalized path, it may not cater to the specific needs, challenges, and contexts of all organizations.
- Adhering too strictly to the curve might result in missed opportunities or challenges unique to an organization’s specific industry or market.
- Risk of complacence
- Organizations might feel that reaching a particular stage on the curve is ‘good enough’, leading to complacency.
- This can stifle innovation and growth, making the organization vulnerable to competitors who continue to innovate.
- Resource intensive
- Moving up the curve requires significant investments, not only in technology but also in skills, training, and cultural shifts.
- Smaller organizations or those with limited resources might find it challenging to progress, which can be demotivating.
The data value curve provides a valuable framework for understanding the progression of data analytics maturity. While it offers a range of benefits in terms of guiding strategy and investments, organizations should use it judiciously, adapting it to their unique needs and contexts.
Data value curve template: How to generate one? #
A data value curve template is a visual representation tool that helps organizations chart their progression in leveraging data. It provides a framework to plot the increasing value an organization derives as it improves its data handling and analytics capabilities.
Creating a data value curve involves identifying and plotting the stages of data maturity against the value derived. The curve generally slopes upward, indicating that as you progress in data maturity, the value you derive from your data should increase.
Steps to generate a data value curve template:
- Define the axes
- Identify stages of data maturity
- Assess current stage
- Plot the stages on the template
- Highlight key investments & initiatives
- Review & iterate
- Engage stakeholders
Let us understand each of them in detail:
1. Define the axes #
- Horizontal axis (X-axis): Represents the progression in data maturity. This can range from basic stages like ‘Data Collection’ to advanced stages such as ‘Proactive Decision Making’.
- Vertical axis (Y-axis): Represents the value derived from the data. This can be subjective based on the organization’s goals and can represent metrics like revenue, operational efficiency, or customer satisfaction.
2. Identify stages of data maturity #
- List down the stages of data usage and analytics your organization recognizes. As discussed before, these might include stages like Data collection, Descriptive analytics, Diagnostic analytics, Predictive analytics, Prescriptive analytics, and Proactive decision making.
- Customize as needed: While there are standard stages commonly recognized, you might find that your organization has specific intermediate stages or a different sequence.
3. Assess current stage #
- Conduct an internal assessment to determine where your organization currently stands on this curve.
- Use tools like questionnaires, stakeholder interviews, or third-party assessments to gain an accurate picture.
4. Plot the stages on the template #
- Mark each stage of data maturity on the X-axis.
- Draw a curve that represents the increasing value as you move from one stage to the next on the Y-axis. Remember, this is a general representation; the actual value jumps between stages might vary based on specific organizational experiences.
5. Highlight key investments & initiatives #
- For each stage, note down key investments or initiatives required to move to the next stage. This can include technology investments, training programs, hiring expertise, etc.
- This provides clarity on the steps and resources required for progression.
6. Review & iterate #
- Regularly revisit the data value curve template, especially after major initiatives or at periodic intervals.
- Assess any shifts in your organization’s position on the curve and make necessary adjustments to the roadmap.
7. Engage stakeholders #
- Ensure that relevant stakeholders, including senior leadership, IT teams, and business units, are engaged and aligned with the curve’s insights and roadmap.
- This ensures organizational buy-in and a cohesive approach to progressing along the curve.
The data value curve template serves as both a diagnostic tool and a roadmap. While it provides insight into the current state of data maturity, it also offers a strategic vision for the future. By regularly updating and referencing the template, organizations can stay aligned in their efforts to harness the full potential of their data.
4 Tools to generate data value curve #
Generating a data value curve often requires a mix of visualization tools, assessment tools, and platforms that can collate and interpret data from various sources.
Below are some of the tools that can be instrumental in creating and maintaining a data value curve:
- Data visualization tools
- Data assessment tools
- Data analytics platforms
- Collaboration tools
Let us understand each of them in detail:
1. Data visualization tools #
These tools allow you to create a visual representation of the Data Value Curve, plot progress, and make it shareable and understandable for stakeholders.
- Microsoft excel or google sheets - Both of these spreadsheet tools offer charting and plotting capabilities. You can easily create a data value curve using the graphing functions.For organizations just starting out or those that require basic visual representations, Excel or Google Sheets is often sufficient. They offer flexibility and are widely understood across industries.
- Tableau - Tableau is a more advanced data visualization tool that can not only plot the data value curve but can also integrate real-time data analytics.
For organizations looking to frequently update their curve based on real-time data or seeking more interactive visualizations, Tableau is a strong choice. It also supports advanced visual analytics.
2. Data assessment tools #
Before plotting the curve, you need to assess where your organization stands in terms of data maturity
- Data maturity assessment frameworks - Several consulting firms and industry groups offer data maturity assessment frameworks. These usually come in the form of questionnaires or guided assessments.
By going through these assessments, organizations can gain an understanding of their current data maturity level. The results can then be plotted on the data value curve.
3. Data analytics platforms #
To derive value and insights from your data, you’ll need platforms that can process and analyze vast amounts of information.
-
Python or R: Both are powerful programming languages commonly used in data analytics. With the right libraries (like pandas for Python), they can process, analyze, and even visualize data.While these require more technical expertise, they offer unparalleled flexibility. Custom analytics can be developed to feed into your data value curve assessments.
-
Power BI: Microsoft’s Power BI is a business analytics tool that offers data visualization alongside robust analytics capabilities.It’s integrated with other Microsoft products, making it a seamless choice for many businesses. The visualizations are interactive, and the platform can directly feed into the Data Value Curve for real-time updates.
4. Collaboration tools #
As the data value curve is a strategic tool, collaboration across departments is crucial.
- Slack or Microsoft teams: Both are collaboration platforms that allow teams to communicate, share files, and integrate other tools.When generating and updating the data value curve, regular communication and collaboration between IT, business units, and leadership are essential. Platforms like Slack or Teams enable this seamless communication.
The selection of tools for generating a data value curve should be based on an organization’s unique needs, technical capabilities, and strategic objectives. While the aforementioned tools can be extremely useful, the key lies in how they are used in conjunction with a clear understanding of the organization’s data maturity journey.
Summarizing it all together #
The data value curve is more crucial than ever. This curve provides a roadmap for how businesses can elevate the value of their data from mere information to actionable insights and ultimately, to strategic assets.
Data value curve is not just a theoretical concept; it’s a practical tool that can guide your organization in its journey towards becoming truly data-driven. By understanding each stage of the curve and recognizing its potential advantages and pitfalls, you can make more informed choices about data collection, analysis, and utilization.
As data continues to become an invaluable resource in the digital age, harnessing its full potential is not just an advantage—it’s a necessity.
Data value curve: Related reads #
- 11 Benefits of Data Visualization You Can’t Ignore in 2023
- 4 Data Collection Techniques: Which One’s Right for You?
- The Do’s and Don’ts of Data Visualization
- Why Visualize Data?
- What is Data Governance? Its Importance & Principles
- Data Governance 101: Principles, Examples, Strategy & Programs
- What is Metadata? - Examples, Benefits, and Use Cases
- Metadata Management: Benefits, Automation & Use Cases
- Data Catalog: Does Your Business Really Need One?
Share this article