The Evolution of Data Quality: From the Archives to the New Age
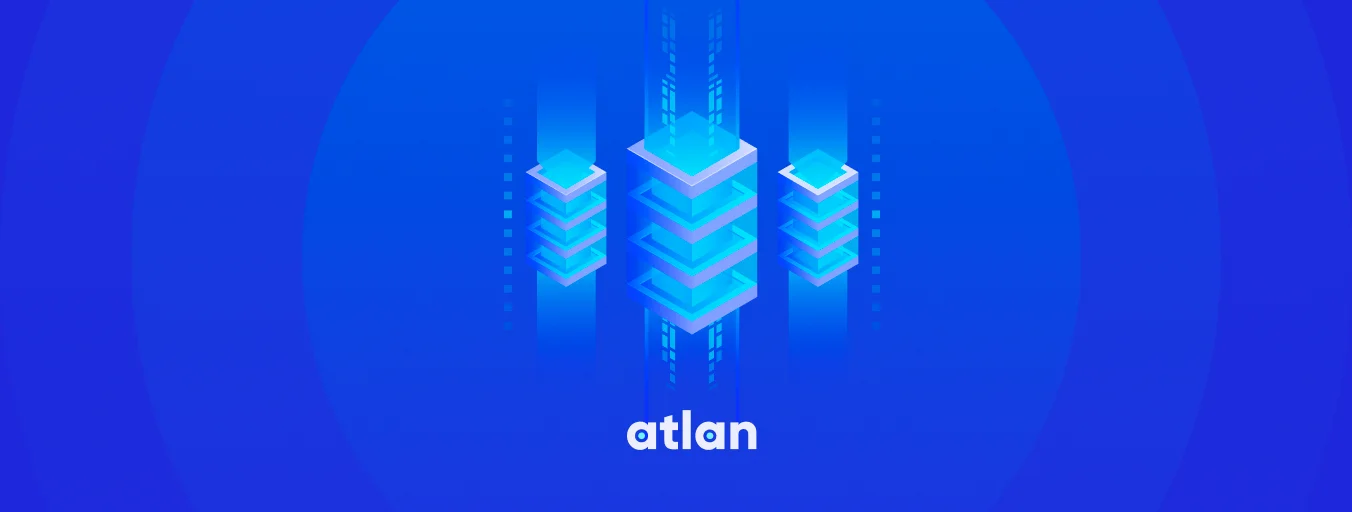
Share this article
Data quality refers to the degree to which data is accurate, consistent, timely, complete, and usable for its intended purpose.
As we transition from analog archives to digital platforms and into an era replete with big data, machine learning, and artificial intelligence, the parameters of what constitutes ‘quality’ data have transformed dramatically.
In this article we will learn the transformative journey that data quality has undertaken—from being an afterthought in the archives to becoming a dynamic, evolving entity in the new age.
Let us dive in!
Table of contents #
- What is modern data quality?
- Evolution of data quality
- Enhancing data quality with MDM, RDM & data catalog integration
- Future of data quality
- Summary
- Related reads
What is modern data quality? #
Modern data quality is no longer just a technical issue relegated to IT departments; it’s a holistic business discipline that plays a crucial role across an organization’s functions.
The quality of data impacts everything from decision-making and strategy formulation to customer relationship management and marketing. By adopting a company-wide approach to data quality, organizations can ensure that the data they rely on is accurate, consistent, and actionable.
Poor data can significantly undermine these technologies, wasting resources and leading to incorrect conclusions. On the other hand, high-quality data can exponentially increase the effectiveness of these tools, thereby amplifying competitive advantage, enhancing customer satisfaction, and driving innovation.
How has the data quality evolved over time? #
The evolution of data quality has been a remarkable journey, adapting and growing alongside advancements in technology and changes in business needs.
Below is a detailed look at how data quality has evolved over time:
- Early days: Tabulation and records
- Pre-digital Era: Mechanical and electrical Systems
- Dawn of computing: Basic databases
- Enterprise software and relational databases
- Internet and E-commerce
- Big data and advanced analytics
- Cloud computing and SaaS
- Modern day: Holistic business discipline
- The future: Automated and adaptive systems
Let us understand each of them in detail:
1. Early days: Tabulation and records #
In the very early days, data was recorded manually on paper, in ledgers and logbooks. The quality of data was solely dependent on the individual responsible for recording it, and verification methods were crude or non-existent.
Impact: Errors were frequent and could be catastrophic. There was no formal “data quality management,” as the concept of data itself was rudimentary.
2. Pre-digital Era: Mechanical and electrical Systems #
With the introduction of mechanical and then electrical systems like punch cards, data could be processed faster. However, this also meant that errors in data entry had more significant consequences.
Impact: Data quality began to receive more attention, with basic error-checking methods being developed, albeit still mostly manual.
3. Dawn of computing: Basic databases #
The introduction of digital databases allowed for more significant data storage and complex processing. However, this also brought about new challenges, such as data duplication and inconsistency.
Impact: Organizations began to realize the importance of data quality for effective computing. Data cleansing operations and de-duplication became early efforts in managing data quality.
4. Enterprise software and relational databases #
The 1990s saw the rise of enterprise software and robust relational databases. Data quality now had a direct impact on business performance.
Impact: Tools started to emerge for data quality management, focusing on standardization, validation, and matching. Companies began establishing data stewardship roles.
5. Internet and E-commerce #
The explosion of the internet and e-commerce platforms generated unprecedented amounts of data, requiring real-time processing and analysis.
Impact: Real-time data quality checks became a necessity. Businesses began employing algorithms for automatic data validation and quality assurance.
6. Big data and advanced analytics #
With Big Data, the volume, variety, and velocity of data skyrocketed. Traditional data quality tools were insufficient to handle this complexity.
Impact: Machine learning algorithms and AI started being used for predictive data quality measures, capable of managing and improving the quality of enormous datasets.
7. Cloud computing and SaaS #
Cloud-based services and Software as a Service (SaaS) offerings have decentralized data storage and processing, making data quality even more complex to manage.
Impact: Centralized data quality governance models started to evolve into more flexible, distributed models to adapt to the cloud environment.
8. Modern day: Holistic business discipline #
Today, data quality is considered a holistic business discipline, essential for analytics, machine learning, and AI. It affects almost every aspect of a business, from operational efficiency to customer satisfaction.
Impact: Organizations now have Chief Data Officers and dedicated data governance teams keeping data quality a core business imperative.
9. The future: Automated and adaptive systems #
The ongoing evolution suggests a future where data quality systems will be largely automated, using AI and machine learning to continuously adapt to changing data landscapes.
Impact: The convergence of AI, Big Data, and IoT will make proactive and predictive data quality management the norm, ensuring high-quality data is available for real-time decision-making and automated systems.
The evolution of data quality has paralleled broader developments in technology and business practices, from manual records to sophisticated, automated systems today. This has made it not just an IT concern but a business-critical function that underpins the digital age.
Enhancing data quality with MDM, RDM & data catalog integration #
The scope of data quality encompasses several dimensions including accuracy, completeness, consistency, reliability, and timeliness.
It involves not just correcting or cleansing data, but also enriching it and maintaining its integrity across various applications, databases, and operational processes.
The scope of data quality is greatly enhanced by its integration with (MDM), (RDM) and data catalogs. Each of these integrations contributes to a more comprehensive, nuanced, and actionable understanding of an organization’s data assets.
In this section, we will understand how these integration contributes to the scope of data quality:
Integration with Master data management (MDM) #
- Master data management is the process of creating and managing a single, consistent, and accurate version of master data—like customer, product, or supplier information—across the organization. Integration with data quality ensures that this master data is accurate, consistent, and actionable.
- Improvement in scope: When data quality is tightly integrated with MDM, businesses have the potential to unlock the true value of their data by ensuring that the ‘single source of truth’ is actually true. It enhances the effectiveness of MDM by making sure that the master data used for decision-making, reporting, and analytics is of the highest quality.
Integration with Reference data management (RDM) #
- Reference data management involves managing the sets of permissible values that are used by other data fields, such as country codes, product categories, etc. It standardizes the values to be used across different datasets.
- Improvement in scope: By integrating data quality with RDM, organizations can ensure that reference data is consistent and up-to-date. This results in cleaner, more reliable data, as well as improved analytics and reporting. The scope of data quality broadens to include not just the data itself but the contextual standards by which data is measured and categorized.
Integration with data catalogs #
- Data catalogs serve as an organized inventory of data assets in an organization, providing metadata, data lineage, and other important information. They make it easier to find, access, and manage data.
- Improvement in scope: When data quality is integrated with data catalogs, users can readily see the quality of datasets they’re considering for analytics or other applications.This helps in avoiding low-quality data and thereby improves the overall quality of business decisions. In addition, it allows data quality to be assessed and improved at every stage of the data lifecycle.
MDM ensures that the data at the core of business processes is sound; RDM maintains the integrity of data by standardizing values; and Data Catalogs make sure that users are aware of the quality of the data they are utilizing. Together, they work in concert to elevate the scope of data quality from a siloed function to a pervasive, organization-wide discipline.
Future of data quality: 6 Things that will shape the future #
The future of data quality management appears poised for a significant transformation, impacted by advancements in technology, evolving business needs, and an increasingly data-driven world.
Let us understand the future trends of data quality:
- Data engineers
- AI-based data quality
- Systems as data consumers
- Real-time data quality management
- Data quality in decentralized systems
- Governance and compliance
Here’s a detailed look at some focal points that are expected to shape the future:
1. Data engineers #
Data engineers will increasingly act as the stewards of data quality, playing a key role in its planning, implementation, and maintenance. They will focus on building robust data pipelines that include real-time data validation, standardization, and transformation capabilities.
Future scope: As the guardians of data architecture, data engineers will work closely with data scientists and analysts to embed data quality right from the data ingestion stage. Automated monitoring tools will be developed to alert engineers about any anomalies or inconsistencies in real time, making data quality a more proactive, rather than reactive, exercise.
2. AI-based data quality #
The integration of Artificial Intelligence (AI) with data quality tools will lead to self-healing and self-managing systems. These AI systems will not just identify but also correct data quality issues automatically, learning continuously from these processes.
Future scope: With the help of machine learning algorithms, AI-based data quality tools will become more intelligent over time. They will not only flag errors but will also predict potential future issues based on historical data. This will make data quality management less resource-intensive and more effective, allowing for predictive rather than just prescriptive or reactive approaches.
3. Systems as data consumers #
In the future, it won’t just be human decision-makers who consume data; automated systems, IoT devices, and even AI algorithms will be significant consumers of data.
Future scope: This will necessitate a change in how data quality is managed. Inconsistent or poor-quality data can disrupt automated workflows and lead to incorrect decision-making by AI algorithms. Therefore, data quality tools will evolve to serve these non-human data consumers by ensuring that data is accurate, consistent, and in a consumable format at all times.
4. Real-time data quality management #
As business decisions become more real-time, there will be a growing need for real-time data quality management.
Future scope: Systems will be developed to validate and clean data as it streams into the organization, enabling immediate insights and decision-making without the latency of batch processing.
5. Data quality in decentralized systems #
With the advent of decentralized technologies like blockchain, there will be new challenges and opportunities for data quality.
Future scope: Decentralized systems can potentially offer immutable, transparent, and verifiable data. However, ensuring that only high-quality data gets recorded on such platforms will be a new avenue for data quality management.
6. Governance and compliance #
As regulations around data privacy and ethics become more stringent, the importance of data quality in meeting compliance requirements will increase.
Future Scope: Data quality tools will integrate compliance checks to ensure that the data not only meets internal quality standards but also complies with external regulations.
In summary, the future of data quality is intricately tied to advancements in technology and shifts in how both humans and systems interact with data. With roles like data engineers becoming more pivotal, and technologies like AI offering new paradigms for quality control, data quality management is set to become more dynamic, efficient, and integral to organizational success.
Summarizing it all together #
Data quality refers to the condition of a set of values of qualitative or quantitative variables. It encompasses various dimensions including accuracy, completeness, reliability, timeliness, and interpretability.
In the era of paper records, data quality was a relatively simple matter of keeping accurate and complete ledgers, where legibility and physical safety (e.g., from water and fire damage) were major concerns.
In conclusion, the evolution of data quality has been a remarkable voyage from the complete records in physical archives to the complexity of managing accuracy.
As we continue to venture into an increasingly data-centric future, the demand for high-quality data will only intensify.
Evolution of data quality: Related reads #
- Data Quality Explained: Causes, Detection, and Fixes
- Data Stewardship: The Key to Implementing a Robust Data Governance Program
- 18 Data Validations That Will Help You Collect Accurate Data
- Difference between Master Data Management(MDM) and Metadata Management
- Why It’s Important to Standardize Your Data
- Data Transformation: Definition, Processes, and Use Cases
Share this article