Amundsen Data Catalog: Understanding Architecture, Features, Ways to Install & More
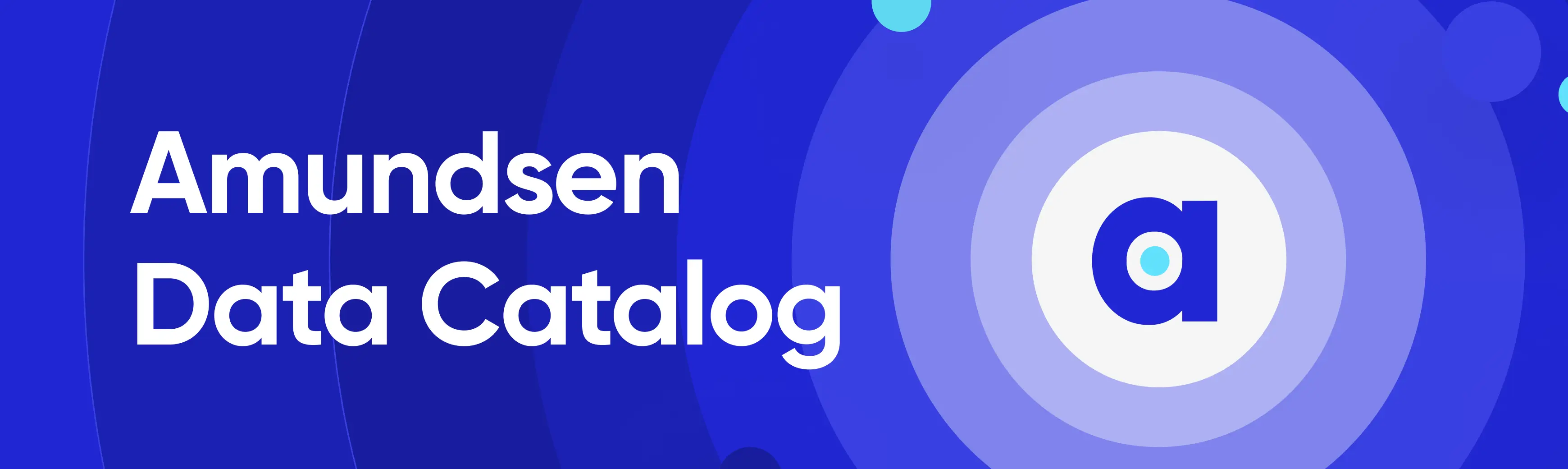
Share this article
Amundsen data catalog: The origin story
Amundsen was born out of a need for solving data discovery and data governance at Lyft. Soon after starting Amundsen’s journey within Lyft, it was open-sourced in October 2019. It has grown in popularity and adoption since then.
This article will take a deep dive into Amundsen’s architecture, features, and use cases. In the end, we’ll also talk about other open-source alternatives to Amundsen. But before that, let’s discuss why and how Amundsen was created.
Table of contents
- Amundsen data catalog: The origin story
- Architecture
- Features
- How to set up Amundsen
- Open-source alternatives to Amundsen
- Conclusion
- Related reads
Is Amundsen open source?
Yes, Amundsen was open-sourced in 2019, but before that, there were several open-source data catalogs such as Apache Atlas, WeWork’s Marquez, and Netflix’s Metacat, among others. Some of these tools were created to suit certain data stacks, and the rest were not very flexible.
Here’s the timeline to give you an idea of where Lyft’s Amundsen came in compared to other open-source data catalogs:
Timeline showing the release of open-source data catalog tools. Image by Atlan.
With Amundsen, the engineering team at Lyft decided to look at the problem of data discovery and governance from a fresh approach using a flexible microservice-based architecture. With this architecture, you could replace many of the components based on your preferences and requirements, which made it enticing for many businesses.
Over the last few years, data catalogs have made life easier for engineering and business teams by enabling data discovery and governance across data sources, targets, business teams, and hierarchies.
With time, data catalogs are building newer features, such as data lineage, profiling, data quality, and more, to enable various businesses to benefit from the tools. Amundsen’s story isn’t much different. Let’s begin by understanding how Amundsen is architected and how it works.
Amundsen Data Catalog Demo
Here’s a hosted demo environment that should give you a fair sense of the Lyft Amundsen data catalog platform.
Amundsen architecture
Amundsen’s architecture comprises four major components.
- Metadata service
- Search Service
- Frontend service
- Databuilder utility
Specific technologies back these components out of the box, but there’s enough flexibility to use drop-in or almost drop-in replacements with some customizations.
As with every application, Amundsen has backend and frontend components.
The backend comprises the metadata and search services — different types of data stored back both. The frontend component has only one service, which is the frontend service.
Amundsen also has a library that helps you connect to different sources and targets for metadata management. This library is collectively called the databuilder utility. Before discussing the services in detail, let’s look at the following diagram depicting Amundsen’s architecture:
Schematic representation of Amundsen architecture. Image by Atlan.
1. Metadata service
The metadata service provides a way of communicating with the database that stores the actual metadata that backs your data catalog. This might be the technical metadata stored in information_schema in most databases, or it might be business-context data or lineage metadata.
As mentioned in the article, Amundsen was created to be more flexible than earlier avatars of data catalogs; the API is designed to support different databases for storing the metadata. The metadata service is exposed via a REST API. Engineers and business users can use this API to interact programmatically or via the front end with Amundsen, respectively.
Like many other data catalogs, Amundsen’s default choice is neo4j, and you can use proprietary graph databases like AWS Neptune or even different data catalogs like Apache Atlas. The databuilder utility, which we will discuss later, provides a way to integrate Amundsen with Atlas.
from apache_atlas.client.base_client import AtlasClient
from databuilder.types.atlas import AtlasEntityInitializer
client = AtlasClient('<http://localhost:21000>', ('admin', 'admin'))
init = AtlasEntityInitializer(client)
init.create_required_entities()
Here’s what the job configuration for loading and publishing a CSV extract from Atlas to Amundsen will look like:
job_config = ConfigFactory.from_dict({
f'loader.filesystem_csv_atlas.{FsAtlasCSVLoader.ENTITY_DIR_PATH}': f'{tmp_folder}/entities',
f'loader.filesystem_csv_atlas.{FsAtlasCSVLoader.RELATIONSHIP_DIR_PATH}': f'{tmp_folder}/relationships',
f'publisher.atlas_csv_publisher.{AtlasCSVPublisher.ATLAS_CLIENT}': AtlasClient('<http://localhost:21000>', ('admin', 'admin')) ,
f'publisher.atlas_csv_publisher.{AtlasCSVPublisher.ENTITY_DIR_PATH}': f'{tmp_folder}/entities',
f'publisher.atlas_csv_publisher.{AtlasCSVPublisher.RELATIONSHIP_DIR_PATH}': f'{tmp_folder}/relationships',
f'publisher.atlas_csv_publisher.{AtlasCSVPublisher.ATLAS_ENTITY_CREATE_BATCH_SIZE}': 10,
f'publisher.atlas_csv_publisher.{AtlasCSVPublisher.REGISTER_ENTITY_TYPES}': True
})
The early documentation of Amundsen suggested that a backend like MySQL could also be used for storing the metadata. With the official documentation entirely outdated, there’s no in-depth tutorial on how to do that.
2. Search Service
The search service is to serve the data search and discovery feature. A full-text search backend helps business users get fast search results from the data catalog. The search service indexes the same data already stored in the persistent storage by the metadata service. Like the metadata service, the search service is also exposed via a search API for querying the technical metadata, business-context metadata, and so on.
Amundsen’s default search backend is Elasticsearch, but you can use other engines like AWS OpenSearch, Algolia, Apache Solr, and so on. This would require a fair bit of customization as you’d need to modify all of the databuilder library components that let you load and publish data into Elasticsearch indexes.
3. Frontend service
The frontend service, built on React, lets business users interact with the Amundsen web application. Both backend services power the front end with REST APIs and search APIs that interact with neo4j and Elasticsearch, respectively. The frontend service is responsible for displaying all the metadata in a readable and understandable fashion.
The frontend isn’t only a read-only search interface; business users can enrich metadata by adding different types of information to the technical metadata. Tagging, classification, and annotation are some examples of metadata enrichment.
Moreover, the frontend is customizable enough to allow you to build other essential features like SSO on top. Amundsen also lets you integrate with different BI tools and query interfaces to enable features like data preview.
A typical integration in the frontend would involve the following steps:
- Ensure the external application is up and running and is accessible by Amundsen
- Modify the frontend code to interact with the new integration
- Modify the frontend configuration file (or directly update the environment variables)
- Build the frontend service for the new integration to take effect to follow this step-by-step, you can go through our in-depth tutorial on setting up Okta OIDC for Amundsen or setting up Auth0 OIDC for Amundsen.
4. Databuilder utility
Rather than asking you to build your own metadata extraction and ingestion scripts, Amundsen provides a standard library of scripts with data samples and working examples. You can automate these scripts using a scheduler like Airflow. This utility eventually ends up populating all the data in your data catalog.
When using a data catalog, you want to make sure that your data catalog represents the data from various data sources, such as data warehouses and lakes. Amundsen provides the tools you need to schedule the extraction and ingestion of metadata in a way that doesn’t inject any fatigue at the source end. Let’s look at a sample extraction script that extracts data from PostgreSQL’s data dictionary:
job_config = ConfigFactory.from_dict({
'extractor.postgres_metadata.{}'.format(PostgresMetadataExtractor.WHERE_CLAUSE_SUFFIX_KEY): where_clause_suffix,
'extractor.postgres_metadata {}'.format(PostgresMetadataExtractor.USE_CATALOG_AS_CLUSTER_NAME): True,
'extractor.postgres_metadata.extractor.sqlalchemy.{}'.format(SQLAlchemyExtractor.CONN_STRING): connection_string()})
job = DefaultJob(
conf=job_config,
task=DefaultTask(
extractor=PostgresMetadataExtractor(),
loader=AnyLoader()))
job.launch()
In this script, you only need to provide the connection information and the WHERE clause to filter the schemas and tables you want. You can selectively bring metadata into your data catalog, avoiding temporary and transient tables.
On top of extraction and ingestion, the databuilder utility provides various ways to transform your data to suit your requirements. This is a big plus if you want to get metadata from non-standard or esoteric data sources. Let’s look at snippets of an Airflow DAG that does the following things:
- Extracts data from a PostgreSQL database
- Loads into the neo4j metadata database
- Publishes the data to the Elasticsearch database
Here’s the job configuration for the job that takes care of steps 1 and 2:
job_config = ConfigFactory.from_dict({
f'extractor.postgres_metadata.{PostgresMetadataExtractor.WHERE_CLAUSE_SUFFIX_KEY}': where_clause_suffix,
f'extractor.postgres_metadata.{PostgresMetadataExtractor.USE_CATALOG_AS_CLUSTER_NAME}': True,
f'extractor.postgres_metadata.extractor.sqlalchemy.{SQLAlchemyExtractor.CONN_STRING}': connection_string(),
f'loader.filesystem_csv_neo4j.{FsNeo4jCSVLoader.NODE_DIR_PATH}': node_files_folder,
f'loader.filesystem_csv_neo4j.{FsNeo4jCSVLoader.RELATION_DIR_PATH}': relationship_files_folder,
f'publisher.neo4j.{neo4j_csv_publisher.NODE_FILES_DIR}': node_files_folder,
f'publisher.neo4j.{neo4j_csv_publisher.RELATION_FILES_DIR}': relationship_files_folder,
f'publisher.neo4j.{neo4j_csv_publisher.NEO4J_END_POINT_KEY}': neo4j_endpoint,
f'publisher.neo4j.{neo4j_csv_publisher.NEO4J_USER}': neo4j_user,
f'publisher.neo4j.{neo4j_csv_publisher.NEO4J_PASSWORD}': neo4j_password,
f'publisher.neo4j.{neo4j_csv_publisher.JOB_PUBLISH_TAG}': 'unique_tag',
})
Here’s the job config for the task that takes care of step 3:
elasticsearch_client = es
elasticsearch_new_index_key = f'tables{uuid.uuid4()}'
elasticsearch_new_index_key_type = 'table'
elasticsearch_index_alias = 'table_search_index'
job_config = ConfigFactory.from_dict({
f'extractor.search_data.extractor.neo4j.{Neo4jExtractor.GRAPH_URL_CONFIG_KEY}': neo4j_endpoint,
f'extractor.search_data.extractor.neo4j.{Neo4jExtractor.MODEL_CLASS_CONFIG_KEY}':
'databuilder.models.table_elasticsearch_document.TableESDocument',
f'extractor.search_data.extractor.neo4j.{Neo4jExtractor.NEO4J_AUTH_USER}': neo4j_user,
f'extractor.search_data.extractor.neo4j.{Neo4jExtractor.NEO4J_AUTH_PW}': neo4j_password,
f'loader.filesystem.elasticsearch.{FSElasticsearchJSONLoader.FILE_PATH_CONFIG_KEY}': extracted_search_data_path,
f'loader.filesystem.elasticsearch.{FSElasticsearchJSONLoader.FILE_MODE_CONFIG_KEY}': 'w',
f'publisher.elasticsearch.{ElasticsearchPublisher.FILE_PATH_CONFIG_KEY}': extracted_search_data_path,
f'publisher.elasticsearch.{ElasticsearchPublisher.FILE_MODE_CONFIG_KEY}': 'r',
f'publisher.elasticsearch.{ElasticsearchPublisher.ELASTICSEARCH_CLIENT_CONFIG_KEY}':
elasticsearch_client,
f'publisher.elasticsearch.{ElasticsearchPublisher.ELASTICSEARCH_NEW_INDEX_CONFIG_KEY}':
elasticsearch_new_index_key,
f'publisher.elasticsearch.{ElasticsearchPublisher.ELASTICSEARCH_DOC_TYPE_CONFIG_KEY}':
elasticsearch_new_index_key_type,
f'publisher.elasticsearch {ElasticsearchPublisher.ELASTICSEARCH_ALIAS_CONFIG_KEY}':
elasticsearch_index_alias
})
If you want to use different backend storage engines instead of neo4j and Elasticsearch, these out-of-the-box scripts from the databuilder utility need updating. But rest assured; you can plug in other backend systems.
Amundsen features
Amundsen’s architecture enables three main features to enhance the experience of your business teams working with data. These features focus on discoverability, visibility, and compliance.
Some tools before Amundsen didn’t enjoy wide adoption partly due to a less intuitive user interface and poor user experience, which is why Amundsen created a usable search and discovery interface. Let’s look at how discovery, governance, and lineage work in Amundsen.
1. Data discovery
Data discovery without a data catalog involves searching and sorting through Confluence documents, Excel spreadsheets, Slack messages, source-specific data dictionaries, ETL scripts, and whatnot.
Amundsen approaches this problem by centralizing the technical data catalog and enriching it with business metadata. This allows business teams to have a better view of the data, how it is currently used, and how it can be used.
The data discovery engine is powered by a full-text search engine that indexes data stored in the persistent backend by the metadata service. Amundsen handles the continuous updates to the search index that give you the most up-to-date view of the data.
The default metadata model stores basic data dictionary metadata, tags, classifications, comments, etc. You can customize the metadata model to add more fields by changing the metadata service APIs and the database schema.
All of this is exposed to the end user with an intuitive and easily usable web interface, where the end user can search the metadata and enrich it.
2. Data governance
The other major problem that Lyft’s engineering team attacked was dealing with security and compliance around data handling. Data governance helps you answer questions like who owns the data, who should have access to the data, and how the data can be shared within the organization and outside. Amundsen uses the concept of owners, maintainers, and frequent users to answer the questions mentioned above.
Amundsen’s job doesn’t stop displaying what you can access and can’t. It can integrate with your authentication and authorization to provide and restrict access to data based on the policies in place.
Moreover, when dealing with PII (personally identifiable information) and PHI (personal health information), you can define mask and hide data and restrict access based on compliance standards like GDPR, CCPA, and HIPAA.
3. Data lineage
Data lineage can be seen branching out from the data discovery and visibility aspect of data catalogs; however, it has data governance aspects too. Data lineage tells the story of the data - where it came from, how it has changed over its journey to its destination, and how reliable it is. This visibility of the flow of data builds trust within the system and helps debug when an issue arises.
Data lineage has always been there. You could always go to the ETL scripts, stored procedures, and your scheduler jobs to infer data lineage manually, but that was just limited to the engineers and mainly used to debug issues and build on top of the existing ETL pipelines.
A visual representation of data lineage opens it up for use by business teams. Also, the business teams can contribute to the lineage and add context and annotations both for themselves and engineers.
Data lineage builds trust by enabling transparency around data within the organization, which was the third key problem that Amundsen was solving. Many of the popular tools in the modern data stack have automated the collection of data lineage. Amundsen can get lineage information directly from these tools, store it in the backend, and index it to be exposed by the web and search interface.
How to set up Amundsen
Setting up Amundsen on any cloud platform is straightforward. Irrespective of the infrastructure you are using, you’ll end up going through the following steps when installing Amundsen:
- Create a virtual machine (VM)
- Configure networking to enable public access to Amundsen
- Log into the VM and install the basic utilities
- Install Docker, and Docker Compose
- Clone the Amundsen GitHub repository
- Deploy Amundsen using Docker compose
- Load sample data using databuilder
Here’s the detailed guide for you to follow: Amundsen Setup Guide
The Amundsen installation process is detailed in this extensive walkthrough. You can check out cloud platform-specific guides listed below:
Other open-source alternatives to Amundsen
At the beginning of the article, we discussed the timeline of open-source data catalogs. Some of the data catalogs mentioned in that timeline are still active and going, while others, like Netflix’s Metacat and WeWork’s Marquez, haven’t seen wide adoption. Since adoption is one of the key metrics while assessing open-source projects, you are left with projects like Apache Atlas and DataHub that might be worth your attention.
Other up-and-coming open-source alternatives like OpenMetadata and OpenDataDiscovery are also worth considering because of their additional features on top of the basic data catalog.
Look out for other open-source data catalogs, as more will keep coming, given it is still a new area in data and analytics engineering.
Conclusion
This article walked you through Amundsen’s architecture, features, technical capabilities, and use cases. It also discussed the possibility of customizing Amundsen to suit your needs and requirements.
For engineering-first teams, using Amundsen might be a good option, considering that it requires a fair bit of customization to build some basic security, privacy, and user experience features on top.
Share this article