What Is Data Discovery: Definition, Purpose, Process, Techniques, and Tools
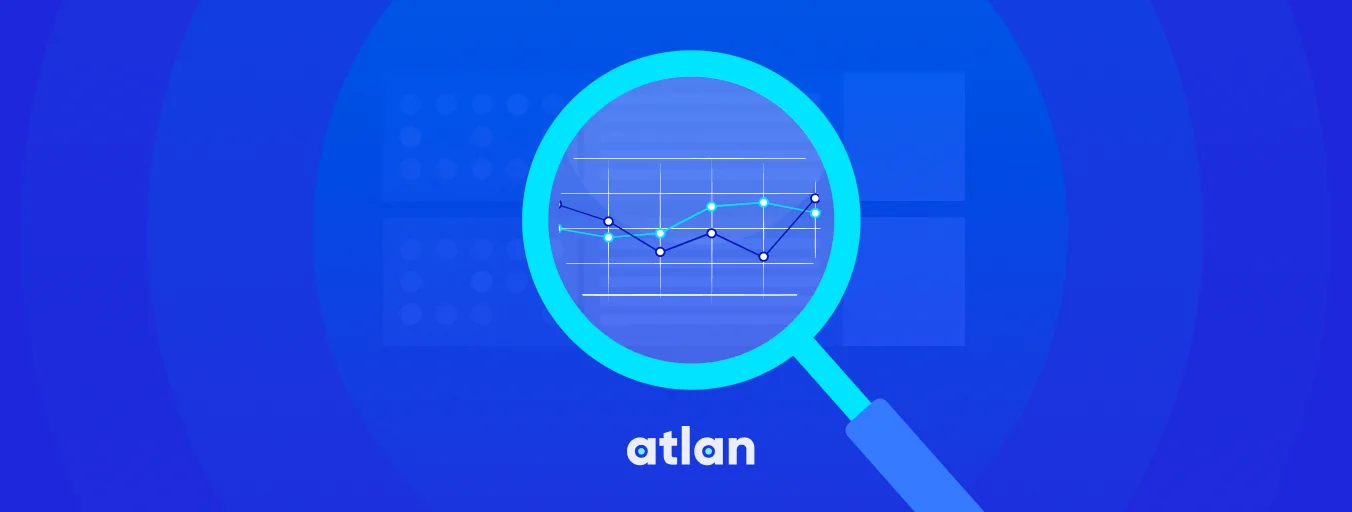
Share this article
What is data discovery? #
Data discovery is the process of analyzing data collected from various sources to spot trends and patterns. Smart data discovery — a term coined by Gartner — enables business users to perform advanced analytics and extract useful insights from data.
Modern data problems require modern solutions - Try Atlan, the data catalog of choice for forward-looking data teams! 👉 Book your demo today
The history of data discovery #
In the 1960s, data discovery had a different name — data mining. Statisticians, economists, and financial researchers would mine data to identify patterns. Data discovery gathered momentum as data mining started becoming essential to enhance processes in the 1990s.
After the First International Conference on Data Mining and Knowledge Discovery (KDD-95) in Montreal in 1995, data discovery — extracting useful knowledge from huge data repositories — became a hot issue for academic research.
Then came the big data boom in the 2000s, starting with Hadoop, real-time processing, and powerful BI tools for data visualization and reporting. According to Gartner, all these advances eventually led to a need for smart data discovery — a next-generation data discovery capability that provides business users or citizen data scientists with insights from advanced analytics.
Before we proceed with smart data discovery and its importance, it’s crucial to know that data discovery isn’t the same as data exploration. Here’s why.
Data discovery vs. data exploration #
Data exploration is the first phase in data analysis, where data scientists use advanced analytics and statistics to understand the data better.
Data exploration allows data scientists to see what they have to work on - within the various data sets. This helps them build a framework to ask the right questions when retrieving data.
Meanwhile, data discovery searches for trends or tendencies in data. In this case, data scientists know exactly what questions they want to answer and where to look for the data to help answer these questions.
Sequentially, data exploration comes first, once data has been prepared for further analysis. Then, you explore data sets to understand which aspects will help you find the answers you need.
Once you’ve identified those data sets, you can dig further to get the specifics. That’s data discovery.
Data discovery: Purpose and importance #
Data discovery helps organizations find the information they need quickly, along with the right context, without having to rely on engineering.
The top data discovery benefits are: #
- Faster and more effective searches
- Easier understanding with adequate context
- Better collaboration across teams
Let’s explore each benefit further.
1. Faster and more effective searches #
A data scientist spends 80% of their time at work sifting through messy data and transforming them into data sets that are ready for analysis.
Data discovery makes it easier to find high-quality, trustworthy data.
For instance, modern data discovery platforms enable semantic search — a Google-like search interface to look for and discover data sets. They also come with filters that let you sort the search results in terms of asset type, classification, owner, and more.
2. Easier understanding with adequate context #
Modern data discovery systems add adequate context to data with capabilities such as a business glossary, description, metadata, and metrics (frequency, missing values, or unique values).
As a result, you understand:
- What data you have and what it means
- Who has access to that data
- What transformations the dataset has undergone
3. Better collaboration across teams #
Effective data discovery tools centralize all data (with context) and eliminate silos. They’re also equipped with in-line chats and discussions to help cross-functional teams collaborate seamlessly.
They can even share data sets with a click to everyone with the right access credentials.
Common data discovery challenges #
Efficient data discovery requires comprehensive, accurate, and credible data. However, there are five common data discovery challenges that you must overcome:
- Processing large volumes of data
- Dealing with data inconsistencies and quality issues
- Causing data chaos without adequate context
- Enforcing access policies for better security
- Maintaining a single source of truth for all data
Let’s explore each data discovery challenge further.
1. Processing large volumes of data #
Organizations collect and store clickstreams, server logs, and other such data types, which involve large volumes of data being produced regularly.
With zettabytes of data being generated every year, the amount of data is undoubtedly an essential factor in enabling proper data discovery.
Processing such volumes requires massive computing infrastructure, repositories, and big tools that automate data discovery, profiling, and transformation.
2. Dealing with data inconsistencies and quality issues #
Data must remain consistent throughout its lifecycle so that it’s reliable and useful. This requires end-to-end visibility from the moment a data set enters your systems and the ability to evaluate the accuracy, completeness, structure, and quality.
For data to be credible, you must also have automated profiling and quality check workflows set up to:
- Spot or flag duplicate or missing data and outliers
- Collect descriptive statistics — mean, median, standard deviation
- Auto-classify data and auto-populate business glossaries
- Generate real-time data quality reports
3. Causing data chaos without adequate context #
Data without context leads to chaos. As a result, questions like “What does X in this data set mean?” or “Why did someone change this value?” become commonplace.
That’s why it’s crucial to add and maintain business metadata with standard attributes such as the last date verified, quality score, last run status, or frequency.
4. Enforcing access policies for better security #
The challenge with enabling data discovery for everyone is managing access. With more business users needing access to data that can help drive decision-making, it’s important to ensure that they can’t access everything.
According to the Ponemon Institute, 71% of employees have access to data they shouldn’t see. Securing confidential or sensitive data with granular access policies is essential to overcome this challenge.
5. Maintaining a single source of truth for all data #
As the number of data sources grows, it’s vital to maintain a centralized repository or catalog — a single source of truth for all data.
Currently, several organizations struggle with problems stemming from siloed data and maintaining multiple versions of the same dataset. It’s not uncommon to have scenarios where the information about data assets is spread across the data tech stack.
Standardizing data definitions, usage rules, formats, processes, and setting up a single source of truth is essential to effective data discovery and solid data management.
Overcoming common data discovery challenges with the modern data stack #
The modern data stack empowers business users and analysts to leverage data assets with self-service, agile data management, and cloud-first and cloud-native design.
The challenges mentioned in the earlier section exist mainly due to a traditional, monolithic data architecture with legacy tools. A modern data stack tackles some of these challenges — processing large volumes of data in real-time or enforcing granular policies to manage access.
However, data discovery for the modern data stack requires more capabilities. Moreover, every organization has a unique set of use cases or workflows, and the data discovery solution must accommodate them. That’s why the biggest tech companies started building their own discovery solutions — Lyft’s Amundsen and Uber’s Databook are two such examples.
However, not every organization has the engineering budget to commission building data discovery tools from scratch.
So, we’ve put together a few core capabilities that you should look for when browsing through data discovery tools for the modern data stack.
Here are some of the characteristics of modern data discovery tools that tackle the data discovery challenges mentioned earlier: #
-
Google-like search for data (and metadata) discovery: Modern data discovery solutions enable the Google-like search and browse experience by allowing you to search using context.
So, anyone can look for columns, SQL queries, glossary terms, BI dashboards, and other such aspects of data assets without needing help from engineering or IT.
More importantly, you can search, explore, and analyze metadata such as query logs.
Despite typos, singular/plural mix-ups, and other keyword errors, you get accurate search results.
-
Auto-profiling: Data profiling helps in determining the accuracy, completeness, structure, and quality of your data. This helps ensure the consistency and quality of data.
Modern discovery tools let you profile for multiple assets in a database or schema at one go — bulk data profiling.
They also auto-generate the key descriptive statistics for data profiles, making it easier for business users to understand and interpret data quality and credibility.
-
Contextual discussions: Modern data discovery platforms have capabilities such as in-line chats that allow you to discuss issues, ask questions about the asset, and resolve issues quickly for each data asset or glossary entry.
You can also make sure that all data-related notifications and alerts are part of your daily workflow — for instance, in the form of Slack messages.
-
Granular access control and governance: Granular access policies help you maintain confidentiality while democratizing data. They grant permissions for viewing or collaborating or deny access to specific data assets.
-
360-degree visibility with embedded collaboration: The modern data discovery solution consolidates everything in one place — a single platform for data discovery, cataloging, and governance.
So, you can search for any data asset, get context, set access-related permissions, track lineage, monitor quality, and more from a single solution — set up a single source of truth.
Such solutions also integrate seamlessly with your daily workflows, enabling embedded collaboration and enhancing the overall user experience.
Check out this curated list of 9 popular data discovery tools in 2023
Conclusion #
Data discovery aids you in understanding your business. It helps you examine data in unique and interesting ways, as well as uncover previously unknown insights. As you modernize your data infrastructure, it’s equally important to ensure that you can easily find and use your data.
Third-generation data discovery and data catalog solutions will be built on the premise of embedded collaboration that is key in today’s modern workplace, borrowing principles from GitHub, Figma, Slack, Notion, Superhuman, and other modern tools that are commonplace today.
If you’re striving to unlock the full potential of your business’s metadata, you must check out all possibilities that Atlan promises to unlock.
Evaluating data discovery tools? Take Atlan for a spin.
Data discovery: Related reads #
- Data discovery tools: 9 popular tools you should consider in 2023
- What is metadata management: Definition, benefits, best practices, and tools
- Data Catalog: Does Your Business Really Need One?
- 5 popular open-source data lineage tools in 2023
- Open-source data catalog software: 5 popular tools to consider in 2023
- Top data catalog use cases intrinsic to data-led enterprises
Photo by Skitterphoto
Share this article