What is the Cost of Bad Data? 12 Ways to Tackle Them!
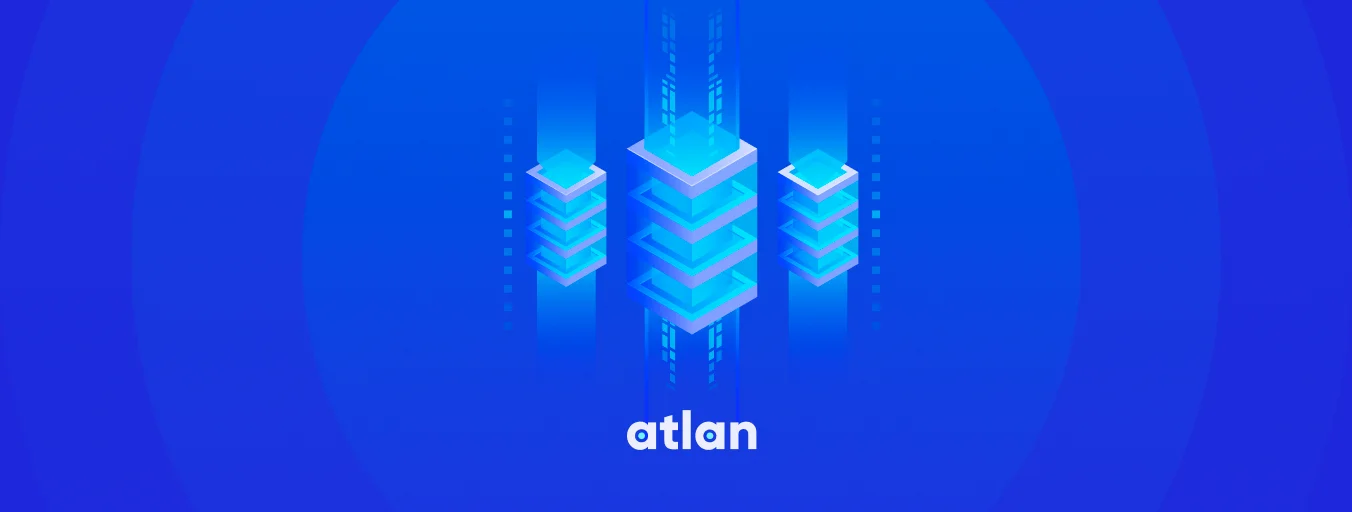
Share this article
As per Gartner’s findings, poor data quality imposes an average annual cost of bad data as $12.9 million on companies across various sectors. As organizations accumulate vast volumes of information to fuel decision-making, operational efficiency, and customer engagement, a critical factor often goes overlooked: the cost of bad data.
Modern data problems require modern solutions - Try Atlan, the data catalog of choice for forward-looking data teams! 👉 Book your demo today
From financial losses to operational setbacks and erosion of trust, the cost of bad data reverberate across every echelon of an organization, demanding vigilant attention and strategic mitigation.
That’s why it’s time to explore the hidden costs of bad data and what organizations can do to minimize the costs of data.
Let’s dive in!
Table of contents #
- What is bad data and what are its types?
- How do you calculate the cost of bad data?
- What is the hidden cost of bad data? (Explained with examples)
- How to minimize the cost of bad data? 12 Strategic ways
- Finally, what are the effects of bad data?
- Bottom line: Summary
- Hidden cost of bad data: Related reads
What is bad data and what are its types? #
Bad data is information in a dataset that is incorrect, incomplete, outdated, or irrelevant. The quality and trustworthiness of data are critical in decision-making processes and in powering various systems, from simple analytics to machine learning models. It can lead to misguided strategies, inaccurate analyses, and operational inefficiencies.
Bad data can be classified into the following types: inaccurate data, outdated data, incomplete data, duplicate data, inconsistent data, irrelevant data, unstructured data, and non-compliant data.
What are the types of bad data? #
“Bad data” refers to information that is inaccurate, misleading, incomplete, or formatted incorrectly, and it can stem from various sources and manifest in different ways. In the context of data quality, “bad data” can often lead to inaccurate analyses, incorrect decision-making, and, in some instances, financial losses or damage to an organization’s reputation.
Here’s a deeper look into various types of bad data:
1. Inaccurate data #
- Erroneous values: Data points that are plainly incorrect or misrepresented.
- Outdated information: Data that is not updated and no longer relevant or accurate.
2. Incomplete data #
- Missing values: Instances where certain data points are not available.
- Truncated data: Situations where only partial data is available due to errors or interruptions during data collection or transfer.
3. Inconsistent data #
- Mismatched data: When data across different systems or datasets is not aligned or synchronized.
- Duplicate data: Repetitive entries that can cause overrepresentation of certain information.
4. Poorly structured data #
- Improper format: Data that does not conform to a specified or expected format.
- Unstandardized data: Data that does not adhere to pre-defined standards, making it difficult to analyze or interpret.
5. Irrelevant data #
- Unnecessary information: Data that doesn’t serve the purpose or context of the analysis.
- Noise: Random or irrelevant information that could distort analyses.
6. Biased or unrepresentative data #
- Sampling bias: When the data collected is not representative of the population or phenomenon being studied.
- Prejudice: Introducing personal or systematic beliefs or attitudes into data collection or analysis, either intentionally or unintentionally.
7. Violated data #
- Integrity issues: Data that has been tampered with or altered inappropriately.
- Security breaches: Instances where data has been accessed or manipulated maliciously.
8. Misleading data #
- Manipulated data: Information that has been intentionally altered to mislead or produce desired results.
- Anomalous data: Data points that deviate significantly from the norm and could potentially misguide interpretations.
9. Unverified or unvalidated data #
- Lack of source credibility: Utilizing data from sources that are not credible or reliable.
- Non-validated data: Utilizing data that has not been checked or validated for accuracy or reliability.
10. Non-compliant data #
- Legal and regulatory Non-compliance: Data that is not in line with legal, ethical, or regulatory standards, such as GDPR, HIPAA, etc.
Recognizing and mitigating bad data is crucial for maintaining data quality and ensuring that analyses and decision-making are based on reliable and accurate information. This process involves a continuous effort to monitor, clean, and validate data throughout its lifecycle.
How do you calculate the cost of bad data? #
Calculating the cost of bad data can be quite complex due to the multifaceted ways in which poor data quality can impact an organization. In general, bad data affects businesses by hindering decision-making, damaging customer relationships, and introducing inefficiencies and additional costs in operations. Here’s a comprehensive approach to calculating the cost of bad data:
1. Direct financial costs #
- Data remediation costs: Calculate the cost of identifying, cleaning, and repairing bad data. Include manpower, technology, and any third-party services used for data cleansing and management.
- Regulatory fines: If applicable, add costs related to non-compliance with data protection laws, which could involve legal fees and fines.
2. Impact on operational efficiency #
- Increased workload: Measure the extra work (time and resources) required to deal with issues caused by bad data, such as resolving customer complaints or correcting errors.
- Process delays: Calculate the cost of delays and disruptions in business processes due to inaccurate or incomplete data.
3. Customer & reputation impact #
- Customer churn: Estimate the financial impact of customers leaving due to frustrations related to data inaccuracies, such as billing errors or mis-targeted communications.
- Brand damage: Although hard to quantify, try to estimate the potential loss of revenue due to damaged reputation, such as lost sales or decreased customer acquisition.
4. Decision-making impact #
- Poor decisions: Evaluate the cost of decisions made based on inaccurate data. This could involve investments, product launches, or strategic shifts that do not yield expected returns due to unreliable data.
- Opportunity costs: Measure what has been lost in terms of opportunities (like missed sales or investments) due to unavailability or inaccuracy of data.
5. Productivity loss #
- Employee time: Calculate the amount of time employees spend handling issues related to bad data, and convert this time into monetary value based on their salaries or wages.
- Redundant efforts: Evaluate the costs associated with duplicated efforts resulting from inconsistent or redundant data.
6. Technology & infrastructure impact #
- System downtime: Quantify the costs of any system downtime related to data issues, including lost sales, employee idle time, and recovery costs.
- Data storage: Account for unnecessary data storage costs for storing redundant, obsolete, or trivial (ROT) data.
7. Supply chain & inventory impact #
- Inventory costs: Identify additional costs incurred due to inaccurate inventory data, such as holding costs for excess inventory or emergency orders for stockouts.
- Vendor relations: If applicable, calculate any financial impact related to vendor relationships due to unreliable data, such as incorrect order placements or payment issues.
Steps to calculate costs #
- Identify impact areas: Determine the areas of the organization most impacted by bad data.
- Measure quantifiable impacts: Wherever possible, directly measure the financial impact.
- Estimate non-quantifiable impacts: For aspects like brand damage or opportunity cost, use estimates or industry benchmarks.
- Collect data: Use surveys, operational data, and financial records to gather relevant data.
- Analyze: Utilize data analysis to understand patterns, frequency, and severity of data issues.
- Aggregate costs: Combine the calculated and estimated costs from all identified areas to determine the total cost of bad data.
Calculating the cost of bad data provides a tangible metric that can demonstrate the return on investment (ROI) for data quality initiatives and help prioritize areas for improvement in data management and governance.
What is the hidden cost of bad data? (Explained with examples) #
Bad data can be costly for businesses, not just in terms of financial losses but also in missed opportunities, damaged reputations, and more. Below are some of the hidden costs associated with bad data:
- Ineffective decision-making
- Increased operational costs
- Reduced customer trust and loyalty
- Compromised business intelligence and insights
- Regulatory penalties and compliance issues
- Wasted resources on data cleanup
- Damage to brand reputation
- Increased risk in mergers and acquisitions
- Reduced employee morale and productivity
- Lost opportunities
Let’s understand each hidden cost in detail.
1. Ineffective decision-making #
When decision-makers rely on inaccurate or unreliable data, the choices they make may not be in the best interest of the organization. Poor decisions can lead to financial losses, missed opportunities, and strategic blunders that can set the company back significantly.
For example, using incorrect sales data could lead a company to invest heavily in a product that isn’t actually in demand, or conversely, underinvest in a potential bestseller.
2. Increased operational cost #
Unreliable data can lead to inefficiencies in operations. For instance, incorrect inventory data may result in overstocking or understocking of items, both of which have associated costs. Similarly, inaccurate customer data can lead to failed deliveries or miscommunication, which can further escalate operational costs.
For instance, an airline, that has incorrect data about its fuel consumption might over-purchase fuel, leading to unnecessary storage costs.
3. Reduced customer trust and loyalty #
When customers encounter errors due to bad data, such as receiving wrong product recommendations, incorrect bills, or misaddressed communications, their trust in the company diminishes. Over time, these negative experiences can erode customer loyalty, leading to decreased sales and revenue.
If an e-commerce site recommends products based on incorrect purchase history data, customers might feel that the company doesn’t understand their needs, pushing them to competitors.
4. Compromised business intelligence and insights #
Analysts depend on accurate data to derive insights and predict trends. Bad data can distort these insights, leading to misguided strategies and investments. The effort and resources put into analyzing bad data are essentially wasted, not to mention the potential for incorrect conclusions.
If an e-retailer misinterprets data due to inaccuracies and stocks up on winter wear in summer, they could suffer major financial setbacks.
5. Regulatory penalties and compliance issues #
Certain industries are bound by regulations that mandate the accuracy and privacy of data. Non-compliance due to inaccurate, outdated, or mishandled data can lead to hefty penalties, legal ramifications, and loss of licenses.
A hospital mismanaging patient records because of bad data can face lawsuits, penalties, and even lose its license to operate.
6. Wasted resources on data cleanup #
A significant amount of time and money is spent on cleaning up and rectifying bad data. This involves not only the immediate cost of the cleanup process but also the opportunity cost of diverting resources away from more strategic initiatives.
If a bank, for example, has multiple incorrect records for customers, they’d need to manually verify and correct each entry, diverting manpower from other critical tasks.
7. Damage to brand reputation #
Repeated issues arising from bad data can tarnish the image of a company. In the age of social media, news of mistakes or poor customer experiences can spread quickly, potentially leading to a broader public relations crisis.
A simple error like sending promotional emails to users who’ve opted out can spark backlash and negative publicity.
8. Increased risk in mergers and acquisitions #
When companies consider mergers or acquisitions, they often conduct due diligence to understand the assets and liabilities of the target company. If a company’s data is found to be unreliable, it may devalue the company or increase the perceived risk of the acquisition, impacting the terms of the deal or even derailing it altogether.
If a tech firm looking to acquire a startup discovers that user engagement data is inflated, it might reconsider the acquisition or substantially lower its offer, impacting the startup’s valuation.
9. Reduced employee morale and productivity #
Constantly dealing with issues arising from bad data can be demoralizing for employees. It can lead to increased frustration, decreased faith in the organization’s systems, and a drop in overall productivity.
If a customer support team is continually dealing with complaints arising from incorrect billings, it can lead to burnout and higher turnover rates.
10. Lost opportunities #
This is perhaps the most intangible yet significant cost. The decisions not made, the markets not entered, and the innovations not pursued – all because of unreliable data – represent potential growth and opportunities lost for the organization.
If a pharmaceutical company, relying on flawed data, halts the development of a potentially groundbreaking drug, the long-term cost could be billions, not to mention the societal cost of withheld medical advancement.
In a nutshell, while the immediate costs of bad data might seem obvious, the hidden costs permeate various facets of an organization and can significantly impact its long-term viability and success. It’s essential for businesses to recognize these potential pitfalls and invest in robust data management practices to mitigate these costs.
How to minimize the cost of bad data? 12 Strategic ways #
Minimizing the costs of bad data requires a proactive approach that involves both technological solutions and organizational strategies. Here are some ways to mitigate the negative impacts of bad data.
- Implement data governance policies
- Prioritize data quality from the start
- Regular data audits
- Use data validation tools
- Employ data cleaning solutions
- Maintain regular backups
- Train and educate staff
- Foster a culture of data responsibility
- Invest in data integration tools
- Stay updated on compliance and regulations
- Continuously monitor data sources
- Seek feedback
Let’s explore each way briefly.
1. Implement data governance policies #
- Just as a city relies on governance to maintain order and efficiency, data requires structure and rules. Without a clear data governance policy, different departments may handle data inconsistently, leading to fragmentation and discrepancies.
- By standardizing processes such as naming conventions, access permissions, and retention policies, organizations can ensure data is unified and coherent.
2. Prioritize data quality from the start #
- Preventing bad data from entering the system in the first place is more efficient than trying to fix it later.
- For instance, if an e-commerce company ensures product prices are input correctly during listing, it can prevent potential revenue loss from pricing errors and the cost of fixing them post-publication.
3. Regular data audits #
- Think of this as a regular health check-up for data. Without periodic assessments, minor inaccuracies can grow into major issues. An audit can reveal patterns of errors, helping identify areas for improvement.
- For instance, a regular audit might show that a particular data entry team consistently enters data incorrectly, pointing to a need for retraining.
4. Use data validation tools #
- Automated tools can check data against predefined criteria. For example, a system could reject any phone number entries that don’t fit the format of valid numbers in a particular country.
- This immediate feedback can prevent erroneous data from entering the system.
5. Employ data-cleaning solutions #
- Over time, even with preventive measures, some bad data might slip through. Data cleaning solutions scan databases to identify anomalies, such as duplicate records or outdated information.
- It’s like spring cleaning, ensuring the environment remains efficient and clutter-free.
6. Maintain regular backups #
- Imagine the costs and repercussions of losing months of customer transaction data due to a technical glitch.
- Regular backups act as a safety net, ensuring data can be restored to its last known good state, minimizing downtime and data loss.
7. Train and educate staff #
- Human error is a leading cause of bad data. Regular training ensures that employees are updated on the best practices of data entry and understand the implications of errors.
- It’s like ensuring every player on a football team knows the game’s rules to prevent avoidable fouls.
8. Foster a culture of data responsibility #
- Beyond just training, fostering a culture where data quality is valued can lead to self-policing and peer accountability.
- In environments where data integrity is seen as everyone’s responsibility, there’s a collective effort to uphold standards.
9. Invest in data integration tools #
- Many organizations source data from multiple channels, be it sales from online and offline stores or user data from various platforms.
- Integration tools ensure that when this data converges, it does so seamlessly, without creating duplicates or inconsistencies.
10. Stay updated on compliance and regulations #
- Regulations aren’t just legal mandates; they often embody industry best practices.
- By staying updated, companies not only avoid legal penalties but also benefit from adhering to standards recognized as effective by industry bodies.
11. Continuously monitor data sources #
- An organization might rely on third-party data feeds for market trends, weather predictions, or news updates.
- If one of these feeds starts delivering inaccurate data, it’s crucial to identify the lapse quickly. Regular monitoring ensures that external data sources remain reliable.
12. Seek feedback #
- Feedback is a valuable tool for improvement. By opening channels for users, clients, or even employees to report data inconsistencies, organizations create an extra layer of verification.
- For example, if a delivery service receives feedback about wrong addresses, it can correct these anomalies, improving service quality.
In short, minimizing the costs of bad data is a multi-faceted effort, combining technology, training, culture, and vigilance. The goal is to create an ecosystem where data accuracy is championed at every level, from the entry point to its application.
Finally, what are the effects of bad data? #
Bad data can have numerous cascading effects across an organization, impacting various facets like operational efficiency, financial performance, customer relationships, and strategic decision-making. Below, let’s delve into some of these effects in detail:
1. Impaired decision-making #
- Inaccurate insights: Poor data leads to misleading analytics and insights, which can misguide strategic and operational decisions.
- Strategic risks: Decisions based on bad data can misalign the organization’s strategy with market realities, leading to financial and competitive risks.
2. Operational inefficiencies #
- Process breakdowns: Inaccurate data can disrupt operational processes, causing delays, errors, and inefficiencies.
- Increased costs: Resources and time spent rectifying errors and dealing with the consequences of bad data increase operational costs.
3. Financial consequences #
- Revenue loss: Incorrect pricing, mismanaged orders, and customer churn due to bad data can erode revenues.
- Unnecessary expenditure: Investing in projects or initiatives based on flawed data can result in wasteful spending.
4. Customer dissatisfaction #
- Poor customer experience: Inaccurate customer data can lead to issues like miscommunication, billing errors, and failed deliveries, impairing the customer experience.
- Loss of trust: Customers may lose trust in an organization if they experience issues arising from inaccurate data, such as receiving irrelevant marketing communications.
5. Compliance and legal issues #
- Regulatory fines: Non-compliance with data protection regulations due to poor data management can result in legal actions and fines.
- Legal risks: Inaccurate data, especially concerning customers and financial transactions, can expose the organization to legal vulnerabilities.
6. Damaged reputation #
- Brand devaluation: Persistent issues arising from bad data can tarnish the brand image and devalue its perception in the marketplace.
- Negative publicity: Public exposure of issues, especially those concerning customer data and privacy, can result in negative publicity.
7. Ineffective marketing #
- Misaligned campaigns: Marketing campaigns based on inaccurate customer data may be poorly targeted and fail to engage the intended audience.
- Wasted marketing budget: Resources spent on misguided campaigns due to bad data represent a direct financial loss.
8. Supply chain disruptions #
- Inaccurate forecasting: Bad data can lead to flawed demand forecasting, resulting in inventory issues, such as stockouts or overstocks.
- Vendor relationship strains: Inaccurate procurement and payment data can strain relationships with suppliers and vendors.
9. Employee morale and productivity #
- Frustration and burnout: Constantly dealing with issues arising from bad data can frustrate employees and lead to burnout.
- Decreased productivity: Time and effort spent rectifying data issues divert resources away from value-generating activities.
10. Stifled innovation #
- Misdirected R&D: Research and development efforts based on inaccurate market and customer data may fail to yield viable products or services.
- Lost opportunities: Inability to recognize and capitalize on opportunities due to unreliable data may hinder innovation and growth.
Addressing the effects of bad data requires a holistic approach, involving technological investments, process enhancements, and fostering a data-centric culture within the organization.
Bottom line: Summary #
- While the immediate implications of bad data may be apparent, the concealed costs infiltrate every facet of an organization, significantly influencing its long-term viability and prosperity.
- Recognizing these latent pitfalls, businesses must invest in robust data management practices to counteract these consequences effectively.
- Through proactive measures such as data governance, quality prioritization, regular audits, automation tools, and fostering a culture of responsibility, organizations can leverage the power of accurate data and safeguard their operations, reputation, and growth trajectory.
Hidden cost of bad data: Related reads #
- What is Data Governance? Its Importance & Principles
- Data Governance Policy — Examples & Templates
- How to Write a Successful Data Governance Policy?
- Data Quality Explained: Causes, Detection, and Fixes
- How to Improve Data Quality in 10 Actionable Steps?
- Data Cleaning, Management, and Tagging: The Best Practices
- 5 Common Mistakes That Lead to Bad Data Visualization
Share this article