Dagster vs Airflow: Data Orchestration Capabilities, UX, Setup, and Community Support
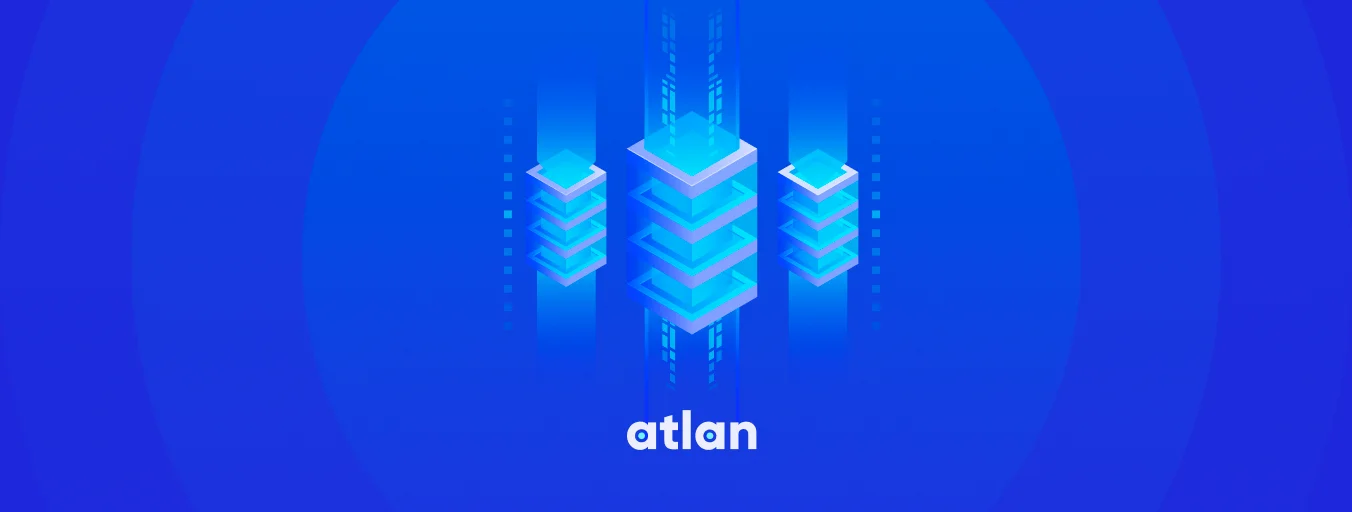
Share this article
Dagster and Airflow are popular open-source data orchestration tools that can be used to optimize a data pipeline.
While Airflow is a battle-tested open-source data orchestration tool that uses DAGs to manage workflows, it is ideal for static and slowly changing workflows. Dagster is relatively new and offers a declarative approach to data orchestration.
In this article, we’ll compare Dagster vs Airflow to understand their orchestration capabilities, ease of use, setup, and more.
Modern data problems require modern solutions - Try Atlan, the data catalog of choice for forward-looking data teams! 👉 Book your demo today
Table of Contents #
- Dagster vs Airflow: Analyzing data orchestration
- UI
- Error detection and messaging
- Setup and community support
- Integrations and plugins
- Final verdict
- Related Reads
Dagster vs Airflow: Analyzing data orchestration #
Airflow and Dagster differ in how they manage workflows.
Apache Airflow is task-based — you define each task and its dependencies separately. Airflow supports dynamic task generation, and as a result, you can create several tasks at runtime using the data that’s available. However, it can be challenging and time-consuming to isolate dependencies and provision infrastructure.
Meanwhile, Dagster is pipeline-based — you define the entire pipeline as a single unit, with tasks nested inside it. Each unit is called a software-defined asset (SDA).
This can make it easier to manage dependencies in complex pipelines. As a result, Dagster can support local development, unit testing, CI, code review, staging, and debugging of data pipelines.
Orchestration is the glue in a data stack and schedule periodic runs of pipelines. An important feature is backfilling in case of an error.
— Rugg (@rd_rugg) November 10, 2022
The main player is Airflow which has some limitations.
Prefect and Dagster are gaining adoption trying to solve those. pic.twitter.com/Tun0wq1D5W
With Airflow leading, Prefect and Dagster rise to address its limitations. - Source: Twitter.
Next, let’s compare Dagster and Airflow in terms of their UI, error detection and messaging, ease of setup, and community support.
Dagster vs Airflow: UI #
The cloud- and container-native Dagster focuses on user experience and comes with strong data validation and error-handling capabilities.
Dagster’s UI focuses on user-friendliness and UX quality - Source: Dagster.
Meanwhile, Apache Airflow provides a web-based UI and built-in operators for common tasks.
Airflow’s web-based UI (Graph View). - Source: Airflow.
Debugging Airflow tasks is only possible either through IDE tools or by manually running a task, with additional support from various logging mechanisms and metric emissions.
Several users flag Airflow’s clunky interface and their struggles with monitoring and troubleshooting DAGs as a challenge.
A discussion on Reddit highlighting the lack of user-friendliness in Airflow - Source: Reddit.
Dagster vs Airflow: Error detection and messaging #
Error detection #
Let’s start by looking at error detection.
Dagster prioritizes pre-runtime error detection via a typing system and a configuration framework to catch errors before a pipeline is run.
Using Dagster’s UI, users can monitor sensors, view their evaluations, and manage errors. - Source: Dagster.
Airflow does not have a built-in mechanism for pre-runtime error detection. However, it supports error detection using an Airflow health check. It also supports real-time error notification via integration with Sentry.
However, these features are more about handling errors once they occur rather than preventing them before runtime.
Error messaging #
Dagster provides error messages that offer context on the nature and location of errors, often with suggestions for fixes for efficient debugging. The structured setup, typing, and configuration system in Dagster further ensure meaningful error messages.
"C-ares status is not ARES_SUCCESS"
— Dagster (@dagster) May 17, 2023
Do you know what that means?
Neither did we.@kubernetesio and @awscloud's ECS error messages can be frustratingly cryptic.
Recent enhancements allow Dagster to surface clear and actionable infrastructure errors. pic.twitter.com/Q9FM4PnaJO
Dagster’s recent updates clarify cryptic error messages from @kubernetesio and @awscloud’s ECS. - Source: Twitter.
Airflow’s error messages may necessitate a deeper understanding owing to its technical intricacy. These messages can occasionally be cryptic or devoid of essential context, posing a potentially steeper troubleshooting learning curve.
apache airflow documentation is like “you can do stuff like this, imagine how it work in detail, or maybe read the source code, and no, our error messages will not give you a clue” lol
— @fireantprincess.bsky.social (@feuer_ameise) December 22, 2022
Apache Airflow’s documentation often leaves users guessing details or diving into source code, with unhelpful error messages. - Source: Twitter.
Dagster vs Airflow: Setup and community support #
Dagster’s documentation is thorough and its getting started guide makes it relatively easy for newcomers to get the hang of it.
Dagster’s documentation and getting started guide provide a user-friendly introduction. - Source: Dagster.
Whereas Airflow’s documentation is extensive, it still might feel overwhelming for the uninitiated.
Airflow’s documentation is potentially challenging for newcomers - Source: Airflow.
However, the community around Airflow is robust and active, whereas Dagster’s community is still growing.
There are 8500+ questions about Airflow on StackOverflow while Dagster and Prefect are ~100.
— Christophe (@_Blef) September 6, 2022
It's gonna be hard to bridge this community gap.
Airflow has 8,500+ StackOverflow queries, far outpacing Dagster and Prefect’s 100. - Source: Twitter.
Integrations and plugins #
Dagster, though newer, is growing its plugin ecosystem focusing on modern data stack tool integrations. It supports general integrations with platforms like Airbyte, Databricks, and Docker for containerization.
Additionally, Dagster integrates with cloud and orchestration platforms like Kubernetes and Azure for deployments and data pipeline management.
i am once again asking you sign up for @dagster's community day
— rex ledesma (@_rexledesma) December 6, 2022
i'll be speaking about all the wonderful new integrations (like for @noteable_io, @SnowflakeDB, @duckdb, @AirbyteHQ, @fivetran, and @getdbt) we've made in the past two months!! https://t.co/ct28XR6tyb
Dagster has announced integrations with platforms noteable_io, SnowflakeDB, and more. - Source: Twitter.
Apache Airflow has a mature ecosystem with core extensions for functionalities with providers like Google and Amazon. It also supports File Transfer Protocol (FTP), Apache Pig, and Amazon Athena.
The dagster-airflow package facilitates interoperability between Dagster and Airflow. It’s useful for migrating existing Airflow DAGs into Dagster Jobs/SDAs, and for triggering Dagster job runs from Airflow.
Final verdict #
Airflow, with its mature community and proven scalability, is a reliable choice for complex, large-scale orchestration needs. Here’s how Pedram Navid, the Head of Data at Hightouch, puts it:
“Without a doubt, Airflow is a project that has been around for over a decade, has the support of the Apache Foundation, is entirely open-source, and used by thousands of companies is a project worth considering. In many ways, going with Airflow is the safest option out there — community support and proven usefulness makes it such a safe choice.”
On the other hand, Dagster offers a developer-friendly, pipeline-based, and UX-centric data orchestration platform.
Airflow vs Dagster pic.twitter.com/PTcfP4meHV
— Neelesh Salian 💻 (@neelesh_salian) December 29, 2022
Airflow vs Dagster: Final verdict. - Source: Twitter.
Each tool has its strengths, and the choice between the two would essentially hinge on the unique requirements of your data orchestration tasks, your technical expertise, and the maturity of your data stack.
Dagster vs Airflow: Related Reads #
- What is data orchestration: Definition, uses, examples, and tools
- Open source ETL tools: 7 popular tools to consider in 2023
- 5 open-source data orchestration tools to consider in 2023
- ETL vs. ELT: Exploring definitions, origins, strengths, and weaknesses
- 10 popular transformation tools in 2023
- Dagster 101: Everything you need to know
- Airflow for data orchestration
- Luigi: Spotify’s open-source data orchestration tool for batch processing
Share this article