The Top 12 Data Governance Trends in 2024
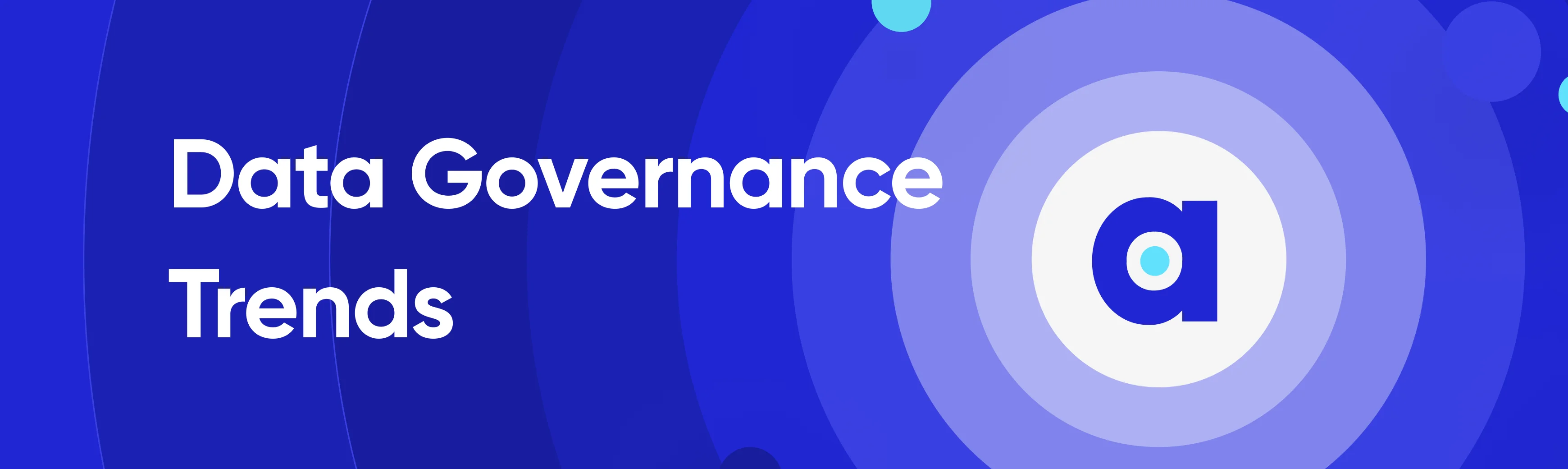
Share this article
In the ever-evolving digital landscape of 2024, data governance has emerged as a powerhouse, setting the stage for a data-driven revolution like never before. Data governance is a set of procedures and guidelines that detail how data is to be properly managed, accessed, and used.
Good data governance helps ensure the quality, integrity, and security of organizational data. Data governance grew out of data stewardship, which is about managing the flow of, and access to, data in order to protect an organization from risk.
It is the invisible backbone that sustains the integrity, quality, and usability of an organization’s data assets, turning them into valuable tools for decision-making, innovation, and growth.
Historically, data governance was seen as a technical or compliance-focused task. But with the explosion of data volumes, the diversity of data sources, and the complexity of regulations, it has transcended its initial boundaries. Today, data governance is ruling the roost, emerging as a strategic function that aligns with core business goals, customer trust, and competitive advantage.
So, let’s dive into the trends of data governance quickly.
Table of contents #
- Data governance trends in 2024
- Increased adoption of AI and machine learning in data management
- Data privacy and compliance with emerging regulations
- Data quality management
- Data lineage and metadata management
- Integration of cloud-based solutions
- Decentralized data governance models
- Real-time data governance
- Data as a Service (DaaS)
- Increased focus on data ethics
- Collaboration and community engagement in data governance
- Emergence of data lakes and data catalogs
- Emergence of data democratization
- Related reads
The top 12 trends of data governance in 2024 #
In this section, let’s dive deep into the top twelve trends in data governance. By embracing these trends, organizations can enhance their data management capabilities, align with regulatory requirements, and leverage data as a strategic asset to drive business success.
- Increased adoption of AI and machine learning in data management
- Data privacy and compliance with emerging regulations
- Data quality management
- Data lineage and metadata management
- Integration of cloud-based solutions
- Decentralized data governance models
- Real-time data governance
- Data as a Service (DaaS)
- Increased focus on data ethics
- Collaboration and community engagement in data governance
- Emergence of data lakes and data catalogs
- Emergence of data democratization
Let’s take a closer look at each one of them.
1. Increased adoption of AI and machine learning in data management #
The integration of artificial intelligence (AI) and machine learning (ML) technologies into data management marks a groundbreaking advancement in how organizations handle their data and govern their data-related processes.
AI and ML are subsets of artificial intelligence that enable machines to learn from data, identify patterns, and make decisions without explicit programming.
In the context of data governance, the application of AI and ML introduces a paradigm shift in automating and enhancing various data-related procedures. Here’s how:
1.1 Automated data processing #
AI and ML can automate data processing tasks, such as data cleansing and preparation. These technologies have the capability to identify inconsistencies, duplicates, and errors within large datasets, significantly reducing the manual effort required for data cleanup. By automating these tasks, organizations can ensure that their data is accurate, reliable, and consistent.
1.2 Predictive analytics #
With the help of ML models, data governance can go beyond mere data management and enable predictive analytics. By analyzing historical data patterns, ML models can make predictions about future trends or potential issues.
This predictive capability empowers organizations to be more proactive in their decision-making, allowing them to identify opportunities or potential risks well in advance.
1.3 Personalized user experience #
AI algorithms can provide customized insights based on individual user needs and behaviors, thus enhancing the user experience.
1.4 Scalability #
With machine learning, the data management process can be scaled to handle large data sets, allowing for real-time analysis and insights.
2. Data privacy and compliance with emerging regulations #
Ensuring compliance with various data privacy laws is a complex and multifaceted challenge that extends its influence over every aspect of data governance.
Data privacy laws and regulations, such as the General Data Protection Regulation (GDPR), California Consumer Privacy Act (CCPA), Health Insurance Portability and Accountability Act (HIPAA), and many others, have been established to protect individuals’ rights and regulate the handling of personal and sensitive data.
2.1 Regulatory landscape #
Understanding and complying with a myriad of regulations like GDPR, CCPA, HIPAA, etc., requires constant vigilance and the updating of policies.
2.2 Data classification #
Identifying and categorizing personal and sensitive data helps in applying appropriate protection measures.
2.3 Risk management #
Regular risk assessments and audits are essential to ensure continuous compliance and to identify potential vulnerabilities.
2.4 Consumer rights #
Regulations often grant consumers specific rights over their data, such as the right to access, correct, or delete their information. Compliance with these rights requires robust data management practices.
3. Data quality management #
Ensuring the quality of data is a critical aspect of data governance because it directly impacts the reliability and usefulness of the insights derived from that data. When data is of high quality, it is accurate, complete, consistent, and reliable.
This means that the information extracted from the data is trustworthy and reflects the true state of affairs within the organization or the market it operates in.
3.1 Validation and standardization #
Implementing validation rules and standardizing data formats helps maintain consistency across different data sources.
3.2 Enrichment and transformation #
Enhancing data by filling gaps and transforming it into usable formats increases its value and applicability.
3.3 Monitoring and reporting #
Continual monitoring of data quality and regular reporting allow for timely detection and rectification of issues.
3.4 Collaboration between business and IT #
Close collaboration between business users and IT ensures that data quality meets specific business requirements.
4. Data lineage and metadata management #
Managing data lineage and metadata plays a crucial role in improving the transparency, traceability, and usability of data assets within an organization.
4.1 Data lineage visualization #
Tools that provide a visual representation of data flow help in understanding how data moves and transforms within the organization.
4.2 Impact analysis #
Understanding data lineage enables an analysis of how changes in data can impact various processes and systems.
4.3 Metadata standardization #
Creating standardized metadata definitions helps in achieving a shared understanding and consistency across different data assets.
4.4 Automated metadata collection #
Automation in collecting and managing metadata ensures up-to-date information and reduces manual efforts.
5. Integration of cloud-based solutions #
The adoption of cloud computing in data governance has revolutionized how organizations manage and leverage their data assets. Cloud computing provides a range of benefits that significantly enhance data governance practices as follows.
5.1 Scalability and flexibility #
Cloud solutions allow organizations to scale their data governance efforts according to business needs without significant capital expenditure.
5.2 Collaboration and accessibility #
Cloud platforms facilitate collaboration across teams and geographical locations, providing access to data and tools anywhere with internet connectivity.
5.3 Security and compliance #
Many cloud providers offer built-in security features and certifications that help in complying with various regulations.
5.4 Cost-effectiveness #
Pay-as-you-go models in cloud computing can result in cost savings, as organizations only pay for the resources they actually use.
6. Decentralized data governance models #
Shifting from a centralized to a decentralized model of data governance represents a fundamental change in how an organization manages and controls its data assets.
6.1 Empowerment of business units #
By decentralizing governance, individual departments have more control and responsibility, promoting a sense of ownership.
6.2 Alignment with business objectives #
Tailoring governance practices to specific business units ensures alignment with their unique goals and requirements.
6.3 Cross-functional collaboration #
Decentralized models encourage collaboration across different functions, fostering innovation and efficiency.
6.4 Challenges in Standardization #
While decentralization offers many benefits, it can also pose challenges in maintaining standardization and consistency across the organization.
7. Real-time data governance #
Real-time data governance enables organizations to respond immediately to changes, enhancing agility and competitiveness.
7.1 Continuous monitoring #
Implementing real-time monitoring of data quality, security, and compliance helps in the immediate detection of issues.
7.2 Real-time analytics #
Providing real-time insights allows for immediate decision-making in response to market changes or operational challenges.
7.3 Integration with operational systems #
Linking governance tools with operational systems ensures that governance policies are applied in real-time as data is generated and used.
7.4 Technology considerations #
Implementing real-time governance requires careful consideration of technologies and infrastructure to support timely data processing and analysis.
8. Data as a Service (DaaS) #
DaaS represents a shift in how data is managed and distributed, centralizing control while enhancing accessibility.
8.1 Centralized data management #
By managing data centrally, DaaS ensures consistency and quality across various applications and processes.
8.2 Customized access #
DaaS allows for tailored access to data for different users, departments, or partners, facilitating collaboration and efficiency.
8.4 Security and compliance #
Centralizing data management through DaaS enables more robust security controls and easier compliance with regulations.
8.5 Cost control #
By providing data as a service, organizations can optimize costs through efficient resource utilization and management.
9. Increased focus on data ethics #
Ethical considerations in data usage extend beyond legal compliance, reflecting societal values and expectations.
9.1 Ethical guidelines and policies #
Developing and adhering to ethical guidelines helps in building trust and alignment with societal norms.
9.2 Transparency and accountability #
Organizations must be transparent about how they collect, use, and share data and be accountable for any adverse impacts.
9.3 Stakeholder engagement #
Engaging with various stakeholders, including customers, employees, and communities, ensures a diverse and inclusive approach to data ethics.
9.4 Consideration of unintended consequences #
Ethical data governance involves assessing potential unintended or harmful consequences of data usage and taking measures to mitigate them.
10. Collaboration and community engagement in data governance #
Collaboration within the organization and engagement with the broader community enriches data governance practices.
10.1 Cross-functional collaboration #
Collaboration between IT, business units, and external partners fosters alignment with organizational objectives and values.
10.2 Community engagement #
Engaging with industry groups, standard-setting bodies, and other communities offers opportunities for learning, benchmarking, and leveraging collective wisdom.
10.3 Shared best practices #
Collaboration and community engagement facilitate the sharing of best practices, tools, and insights, enhancing the overall effectiveness of data governance efforts.
10.4 Building a data-driven culture #
Encouraging collaboration and engagement helps in fostering a data-driven culture where data is recognized as a valuable asset and leveraged accordingly.
11. Emergence of data lakes and data catalogs #
The advent of data lakes and data catalogs has revolutionized how organizations store, organize, and access their vast data resources.
11.1 Data lakes #
Data lakes are storage repositories that can hold vast amounts of raw data in their native format until it’s needed. Unlike traditional databases, data lakes are capable of storing structured, semi-structured, and unstructured data, providing immense flexibility.
1. Scalability #
Data lakes can easily scale to accommodate growing data volumes.
2. Cost-efficiency #
They often provide a more cost-effective solution for storing large data sets.
3. Integration challenges #
Proper integration, governance, and security must be considered to avoid turning the data lake into a so-called “data swamp” where data becomes unmanageable.
11.2 Data catalogs #
A data catalog is a workspace that serves as a context, control, collaboration, and action plane integrating your entire data estate, diverse data users, and divergent data use cases.
1. Discoverability #
Catalogs enable users to quickly find the data they need, reducing time spent searching.
2. Metadata management #
They store and manage metadata, providing context and understanding of the data.
3. Collaboration #
Data catalogs promote collaboration by allowing users to share insights, comments, and ratings about different data assets.
11.3 Synergy between data lakes and catalogs #
Combining data lakes with catalogs provides a robust solution for managing and utilizing large and diverse data sets.
12. Emergence of data democratization #
Data democratization is the process of making data accessible to non-technical users, empowering them to make data-driven decisions without relying on data specialists.
12.1 Accessibility #
Making data available to all employees, regardless of their technical expertise, fosters a more data-driven culture.
12.2 Self-service tools #
Providing self-service tools enables non-technical users to access, analyze, and visualize data without needing specialized skills.
12.3 Enhanced decision making #
By empowering more people to use data, organizations can derive insights and make decisions more quickly and collaboratively.
12.4 Governance considerations #
While democratization provides many benefits, it also requires careful governance to ensure that data is used responsibly and in compliance with regulations.
12.5 Security and privacy #
Ensuring that proper security measures are in place is essential to prevent unauthorized access or misuse of data.
12.6 Skills development #
Along with technology, organizations may need to invest in training and support to ensure that all employees can effectively utilize the available data.
As businesses navigate the multifaceted landscape of global markets, technological advancements, and societal expectations, understanding and embracing these trends in data governance becomes imperative. They are not merely fleeting shifts in the industry but powerful catalysts that are reshaping the way organizations leverage data. By recognizing the importance of data governance and the trends that are shaping its future, businesses position themselves to thrive in a data-driven world, turning information into insights, and insights into impact.
The top 12 trends of data governance in 2024: Related reads #
- What is Data Governance? Its Importance & Principles
- What is Data Stewardship?
- Data Catalog: The Must-Have Tool for Data Leaders in 2024
- Metadata Management: Benefits, Automation & Use Cases
- What Is Data Democratization: Definition, Benefits & Strategy
Share this article