How Do Large Organizations Avoid Data Silos? Strategies, Best Practices, and Challenges
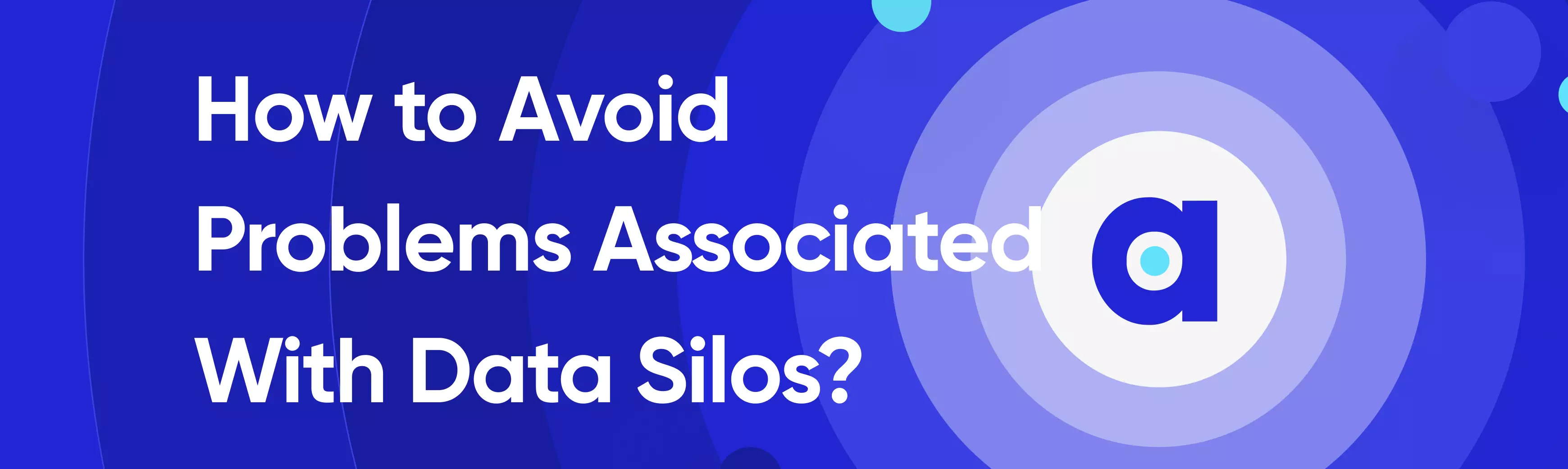
Share this article
Large organizations can avoid the problems associated with data silos by implementing various strategies and practices that promote data accessibility, collaboration, and understanding across teams. Here are some approaches to consider:
- Data governance
- Centralized data storage
- Data cataloging and metadata management
- Cross-functional collaboration
- Data democratization
- Data integration
- Training and education
Let us look into each of the above approaches in brief:
Table of contents #
- Dealing with data silos at large organizations: Taking a closer look
- How do you get rid of data silos in large organizations?
- Why do data silos often occur in large organizations?
- Key factors to consider
- Rounding it all up
- Related reads
1. Data governance #
Establishing a strong data governance framework helps ensure that data is managed and maintained in a consistent and standardized manner. This includes defining roles and responsibilities for data management, creating policies and procedures for data access and usage, and ensuring data quality and integrity.
2. Centralized data storage #
Consolidating data into a central repository, such as a data lake or data warehouse, can help break down data silos by making data accessible to all teams and departments. This allows for easier sharing, collaboration, and analysis of data across the organization.
3. Data cataloging and metadata management #
Implementing a data catalog and managing metadata effectively can help users understand the context of the data they are working with. This includes maintaining a data dictionary, business glossary, and documenting data lineage, changes made, and data sources. This makes it easier for users to find the data they need and trust its accuracy.
4. Cross-functional collaboration #
Encouraging collaboration between teams and departments is crucial for breaking down data silos. This can be done by creating cross-functional teams or data working groups, organizing regular meetings to discuss data-related issues, and promoting a data-driven culture across the organization.
5. Data democratization #
Providing self-service analytics tools and platforms empowers non-technical users to access, analyze, and make decisions based on data. This helps to foster a data-driven mindset across the organization and reduces the reliance on data specialists for basic data tasks. Result? It promotes data democratization across the enterprise.
6. Data integration #
Integrating data from disparate sources and systems can help create a more unified view of the organization’s data. This can be achieved through the use of data integration tools, APIs, and middleware that enable seamless data exchange between systems.
7. Training and education #
Providing training and education on data literacy, data management, and data analytics to employees can help them understand the value and importance of using data effectively. This can also help create a data-driven mindset across the organization.
By implementing these strategies, large organizations can successfully address data silos and create an environment where data is accessible, trusted, and effectively used to drive decision-making and business outcomes.
Dealing with data silos at large organizations: Taking a closer look #
Here’s a quick blueprint and a deeper dive for each approach to help you address data silos in your organization:
1. Data governance #
- Establish a data governance committee with representatives from various departments to oversee data management.
- Define roles and responsibilities for data stewards, data owners, and other data management personnel.
- Develop policies and procedures for data access, usage, privacy, and security.
- Implement data quality management processes to ensure the accuracy, consistency, and completeness of data.
- Regularly review and update data governance policies and practices to keep up with changing business needs and industry regulations.
2. Centralized data storage #
- Assess existing data sources and systems to identify the data that needs to be centralized.
- Select a suitable data storage solution, such as a data warehouse or data lake, based on your organization’s needs and requirements.
- Migrate existing data into the centralized storage and establish processes for future data ingestion.
- Implement access controls and security measures to protect the centralized data storage.
- Monitor and optimize data storage performance and costs regularly.
3. Data cataloging and metadata management #
- Inventory your organization’s data assets and identify the critical data elements that need to be cataloged.
- Select a data catalog tool or platform that supports metadata management, data lineage, and data discovery.
- Populate the data catalog with metadata, including data dictionary, business glossary, data lineage, and data sources.
- Establish processes for maintaining and updating metadata as new data is added or changes are made.
- Train users on how to navigate and use the data catalog effectively.
4. Cross-functional collaboration #
- Create cross-functional teams or data working groups that include members from different departments and roles.
- Schedule regular meetings or workshops to discuss data-related issues, share insights, and collaborate on data projects.
- Encourage open communication and knowledge sharing about data and analytics across the organization.
- Recognize and reward successful cross-functional collaborations and data-driven achievements.
5. Data democratization #
- Evaluate self-service analytics tools and platforms that cater to users with varying levels of technical expertise.
- Implement a self-service analytics solution that provides access to data and enables users to create visualizations, dashboards, and reports.
- Train employees on using self-service analytics tools and interpreting the insights they generate.
- Monitor usage and adoption of self-service analytics tools to identify areas for improvement or additional training.
6. Data integration #
- Identify the data sources and systems that need to be integrated to provide a unified view of your organization’s data.
- Select appropriate data integration tools and technologies, such as ETL (Extract, Transform, Load) tools, APIs, or middleware.
- Develop and implement data integration processes to ensure seamless data exchange between systems.
- Monitor and maintain data integration processes, ensuring they remain up-to-date and efficient.
7. Training and education #
- Assess the current data literacy levels of employees across your organization.
- Develop a comprehensive data training program that includes topics such as data management, data analytics, and data-driven decision-making.
- Offer training through various formats, such as workshops, online courses, or mentorship programs, to cater to different learning preferences.
- Encourage employees to apply their new data skills and knowledge in their day-to-day work.
- Continuously update and expand the training program to address emerging data trends and technologies.
By following these steps, you can systematically address data silos in your organization and promote a culture of data-driven decision-making.
Now that we have understood how to break down data silos at large enterprises, let us look at some common challenges that lie ahead.
But, why do data silos often occur in large organizations? #
Data silos lead to inefficiencies, reduced productivity, and sometimes missed opportunities. In large organizations, the occurrence of data silos is not uncommon, and there are several reasons for their existence, such as:
- Departmental specialization and autonomy
- Rapid organizational growth
- Mergers and acquisitions
- Inconsistent data management strategies
- Different technology platforms and systems
- Resistance to change
- Lack of vision or understanding of integration benefits
- Inadequate IT infrastructure
Let us look at each of the above reasons in brief:
1. Departmental specialization and autonomy #
In large organizations, each department or team may have its own objectives and tools tailored to their specific needs. Over time, these departments may develop or purchase software solutions independently, leading to isolated data sources.
2. Rapid organizational growth #
When organizations grow quickly, either organically or through acquisitions, the integration of systems and data sources might not be the primary focus. This can lead to multiple, disparate systems in use without a unified data strategy.
3. Mergers and acquisitions #
Mergers and acquisitions introduce new systems and data sources into an organization. Integrating these new sources with existing ones is often a challenging process and can lead to the creation of data silos if not done comprehensively.
4. Inconsistent data management strategies #
Without a unified data management strategy, different departments may adopt varied approaches to data storage, processing, and management. This lack of consistency can make integration difficult.
5. Different technology platforms and systems #
Different teams might prefer or be accustomed to different technology solutions, leading to a heterogeneous IT environment. For instance, one department might use a particular CRM system, while another uses a different one. These systems may not be inherently compatible.
6. Resistance to change #
Employees and departments may resist changes to their current systems due to familiarity and comfort with existing processes. This resistance can lead to a persistence of legacy systems that contribute to data silos.
7. Lack of vision or understanding of integration benefits #
Upper management might not recognize the value of integrated data systems, either due to a lack of understanding or because other priorities overshadow the need for integration. Without a top-down mandate, integration initiatives may falter.
8. Inadequate IT infrastructure #
Sometimes, the IT infrastructure itself might not support easy integration of various data sources. This can be due to outdated technologies, lack of interoperability features, or insufficient resources dedicated to data integration.
While data silos can be detrimental to the overall efficiency and decision-making processes of an organization, understanding the reasons behind their existence can help in devising strategies to tackle and prevent them.
How do you get rid of data silos in large organizations? #
Breaking down data silos can be a complex and challenging process, as it often involves addressing deeply rooted organizational, technical, and cultural issues. Here are some general challenges encountered in this process and ways to get rid of them:
- Establish a unified data strategy
- Organizational structure
- Data ownership and privacy concerns
- Engaging leadership and securing executive buy-in
- Adopting cloud-based solutions
- Incompatible systems and formats
- Data quality and consistency
- Limited resources and expertise
- Resistance to change
- Scalability and performance
- Legal and regulatory compliance
Let us look at each of the above challenges in brief:
1. Establish a unified data strategy #
A holistic and organization-wide data strategy defines how data is collected, stored, accessed, and used. This ensures consistency across departments and emphasizes the value of integrated data sources.
2. Organizational structure #
Companies with rigid hierarchical structures or a strong departmental focus may find it difficult to foster cross-functional collaboration and data sharing. In such cases, changing the organizational structure or culture might be necessary to enable better data accessibility.
3. Engaging leadership and securing executive buy-in #
Top-down support is essential for any large-scale organizational change. When leadership understands the risks of data silos and the benefits of integration, they can drive initiatives and allocate resources more effectively.
4. Adopting cloud-based solutions #
Cloud platforms often come with built-in integration capabilities, making it easier to connect different data sources. Moreover, they are scalable and can be accessed from anywhere, which aids in reducing the barriers often associated with traditional on-premises systems.
5. Data ownership and privacy concerns #
Some departments or individuals may be reluctant to share their data due to concerns about data ownership, privacy, or potential misuse. Addressing these concerns requires establishing clear data governance policies, access controls, and security measures to protect sensitive information.
6. Incompatible systems and formats #
Data silos often arise when different departments or teams use incompatible systems, software, or data formats. Integrating these disparate systems and standardizing data formats can be a time-consuming and resource-intensive process.
7. Data quality and consistency #
Ensuring that data is accurate, complete, and consistent across different sources can be challenging, especially when dealing with large volumes of data. Implementing robust data quality management processes is essential for maintaining trust in the data and facilitating its use across the organization.
8. Limited resources and expertise #
Breaking down data silos may require significant investments in new technologies, tools, and personnel. Organizations with limited resources or expertise in data management may struggle to allocate the necessary funding and staffing for these efforts.
9. Resistance to change #
Employees who are used to working within data silos may resist changes to their established workflows and processes. Overcoming this resistance requires clear communication about the benefits of breaking down data silos, as well as providing training and support to help employees adapt to new ways of working with data.
10. Scalability and performance #
As organizations grow and their data needs evolve, maintaining a centralized and accessible data storage solution can become increasingly challenging. Ensuring that data systems can scale to handle increasing volumes and complexities of data is crucial for long-term success.
11. Legal and regulatory compliance #
When breaking down data silos, organizations must consider legal and regulatory compliance requirements, such as data protection laws and industry-specific regulations. Ensuring that data sharing and access practices adhere to these requirements is essential to avoid potential penalties and reputational damage.
By understanding and addressing these challenges, organizations can successfully break down data silos and unlock the full potential of their data to drive better decision-making and business outcomes.
Using tools to break down data silos in large organizations: Key factors to consider #
Selecting the right tools is crucial for effectively breaking down data silos and promoting a data-driven culture within an organization. Here’s a list of must-have tools or features to consider when implementing the approaches mentioned earlier:
- Data governance tools
- Data storage solutions
- Data catalog and metadata management tools
- Data integration tools
- Self-service analytics platforms
- Data quality management tools
- Collaboration and communication tools
Now, let us look into each of these tools in brief:
1. Data governance tools #
Look for tools that help you manage and monitor data governance policies and procedures, assign roles and responsibilities, and ensure data quality and integrity. Some popular data governance tools include Atlan.
2. Data storage solutions #
For centralized data storage, choose a solution that suits your organization’s needs, such as a data warehouse (e.g., Snowflake, Amazon Redshift, or Google BigQuery) or a data lake (e.g., Amazon S3, Azure Data Lake Storage, or Google Cloud Storage). These tools enable you to store, manage, and access large volumes of structured and unstructured data from various sources.
3. Data catalog and metadata management tools #
Implement a data catalog tool that allows you to catalog and manage metadata effectively, such as data lineage, data dictionary, business glossary, and data sources. Some popular data catalog tools like Atlan.
4. Data integration tools #
Select data integration tools or platforms that facilitate seamless data exchange between disparate systems and sources. Some popular data integration tools include Apache NiFi, and Microsoft Azure Data Factory.
5. Self-service analytics platforms #
Implement self-service analytics tools that enable users with varying levels of technical expertise to access, analyze, and visualize data. Popular self-service analytics platforms include Tableau, Microsoft Power BI, Looker, and Qlik Sense.
6. Data quality management tools #
Choose tools that help you maintain data accuracy, consistency, and completeness, such as data profiling, data cleansing, and data validation. Some popular data quality tools include Informatica Data Quality, Talend Data Quality, and IBM InfoSphere Information Analyzer.
7. Collaboration and communication tools #
Encourage cross-functional collaboration and communication by using tools that facilitate knowledge sharing and teamwork, such as Slack, Microsoft Teams, or Google Workspace.
But, when you get down to selecting tools, consider the following factors to ensure they are the right fit for your organization:
- Compatibility: Make sure the tools you choose are compatible with your organization’s existing systems, data formats, and workflows.
- Scalability: Ensure that the tools can scale to accommodate your organization’s growing data needs and complexities.
- Ease of use: Choose tools that are user-friendly and easy to learn, especially for non-technical users.
- Security and compliance: Verify that the tools comply with your organization’s security requirements and relevant data protection regulations.
- Cost and resources: Evaluate the costs of the tools, including licensing fees, implementation, and ongoing maintenance, as well as the resources needed to manage and support them.
By carefully selecting the appropriate tools for your organization, you can effectively break down data silos and enable data-driven decision-making across teams and departments.
Rounding it all up #
Data silos are situations where data exists in isolation, making it difficult for various teams to access and collaborate on data-driven decisions. This problem persists despite significant investments in big data and the growing importance of data-driven decision-making.
Breaking down these data silos requires addressing organizational, technical, and cultural challenges. By implementing the strategies mentioned above and selecting the right tools, organizations can overcome these challenges and create an environment that promotes data accessibility, collaboration, and data-driven decision-making.
Data silos in large organizations: Related reads #
- Data Silos 101: Definition, Challenges, and Solutions
- What Is Data Governance? Its Importance, Principles & How to Get Started?
- What Is Data Lineage & Why Is It Important?
- What Is a Data Catalog? & Why Do You Need One in 2023?
Share this article