Top 10 Priorities of Data Teams Explained With Examples in 2024
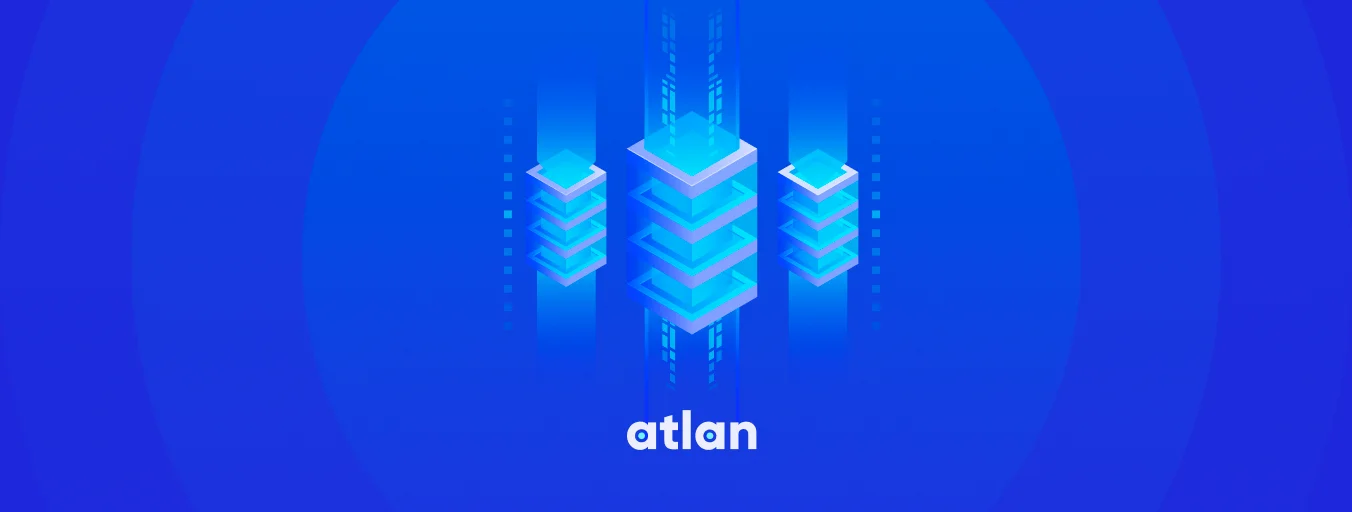
Share this article
In today’s digital age, data stands as the new gold, driving insights, transformations, and innovations across industries. The heart of this data-driven revolution is the data team – a fusion of strategists, engineers, scientists, and analysts who turn raw information into actionable intelligence.
Understanding the goals and priorities of a data team is pivotal to leveraging the power of data to its fullest potential. The year 2024 has brought with it a unique set of challenges and opportunities for data teams worldwide.
In this article, we will closely examine the top 10 priorities for data teams in 2024 along with real-world examples. Let’s dive in!
Table of contents #
- Data ethics and responsibility
- Augmented data management
- Real-time data processing
- DataOps methodology adoption
- Ensuring data privacy and compliance
- Cloud-native data infrastructure
- Expanding data literacy
- Graph analytics and databases
- Quantum computing for data
- Enhancing data visualization
- The top 10 real world examples of data teams priorities
- Bottom line
- The top 10 priorities of data teams: Related reads
With the rapid advancements in technology, an ever-expanding data ecosystem, and heightened global awareness of data rights and ethics, data teams must recalibrate their priorities.
Here are the top ten priorities for data teams this year:
- Data ethics and responsibility
- Augmented data management
- Real-time data processing
- DataOps methodology adoption
- Ensuring data privacy and compliance
- Cloud-native data infrastructure
- Expanding data literacy
- Graph analytics and databases
- Quantum computing for data
- Enhancing data visualization
Let’s understand each priority of the data teams in detail.
1. Data ethics and responsibility #
The age of information brings with it not just possibilities but also ethical challenges. Data teams are the guardians of vast amounts of personal and proprietary data.
- Ethical Implications: As algorithms influence major decisions from healthcare to finance, biases or errors in data can have profound societal impacts. Addressing these biases is paramount.
- Guidelines: Data teams are tasked with crafting strong ethical guidelines that govern data collection, processing, and usage, ensuring that personal data isn’t exploited.
- Training: Ongoing workshops and sessions to sensitize team members to the moral dimensions of their work, emphasizing transparent and fair data practices.
2. Augmented data management #
Incorporating AI into data management can significantly enhance productivity and accuracy.
- Automation: Routine tasks, such as data cleansing and integration, are being automated, freeing up data scientists and engineers for more complex tasks.
- Anomaly Detection: AI can spot inconsistencies or aberrations in data that might escape manual scrutiny, ensuring higher data quality.
- Recommendations: Predictive analytics can guide users in making more informed decisions by offering proactive insights.
3. Real-time data processing #
The modern business landscape demands immediate insights for swift decision-making.
- Shift from Batch Processing: Moving away from periodic batch processing, data streams are now processed instantly for more timely insights.
- Instantaneous Tools: Technologies like Apache Kafka or Flink allow for real-time data ingestion and processing.
- Alert Systems: Instant notifications for stakeholders when specific data thresholds are reached or anomalies detected.
4. DataOps methodology adoption #
DataOps integrates Agile, DevOps, and statistical process controls to improve the quality and speed of data analytics.
- Streamlined Lifecycle: A seamless flow from data collection, processing, analytics to visualization.
- Rapid Delivery: Emphasizing on quick iterations and feedback loops, ensuring that data products and insights are delivered more swiftly.
- Collaboration: Bridging silos between teams, fostering cooperation and shared responsibility.
5. Ensuring data privacy and compliance #
Data breaches or mismanagement can result in heavy fines and loss of trust.
- Monitoring Practices: Continuous audits to ensure adherence to data protection standards.
- Governance Frameworks: Structured policies that determine data access, storage, and usage protocols, ensuring compliance.
- Education: Regular updates about evolving data protection laws and practices.
6. Cloud-native data infrastructure #
The benefits of cloud platforms extend beyond mere storage.
- Cloud-native Solutions: Designing tools and applications to leverage the full capability of cloud infrastructure, including scalability and redundancy.
- Multi-cloud Strategies: Using multiple cloud providers to prevent data loss, ensure availability, and avoid vendor lock-ins.
7. Expanding data literacy #
A holistic data-driven approach requires an organization-wide understanding.
- Promote Understanding: Not limited to data teams; other departments should grasp the basics of data interpretation.
- Training: Workshops to introduce tools, methodologies, and best practices to non-data personnel.
8. Graph analytics and databases #
Graph technology is unlocking new potentials in data understanding.
- Complex Relationships: Understanding intricate data relationships, useful in fields from social networks to supply chains.
- Interconnectivity: Graph databases like Neo4j enable more relational data storage, highlighting connections between data points.
9. Quantum computing for data #
The quantum realm is offering unprecedented computational power.
- Complex Problems: Quantum computers can potentially solve problems in seconds that would take classical computers millennia.
- Encryption: Leveraging quantum principles for enhanced data security.
10. Enhancing data visualization #
With increasing data democratization, visualization is key.
- User-friendly Tools: Visualization tools like Tableau or PowerBI are becoming more intuitive, allowing even non-tech users to derive insights.
- Interactive Elements: Dynamic graphs and charts that update in real-time and allow user interaction for deeper exploration.
As data becomes central to organizational strategy and operations in 2024, the responsibilities of data teams expand and evolve. By deep diving into these priorities, they are better equipped to harness the potential of data, ensuring ethical, efficient, and innovative use.
The top 10 real world examples of data teams priorities #
The world is increasingly oriented around data, and with that, data teams have their tasks cut out for them. While their priorities can be listed on paper, they truly come to life when we examine real-world scenarios. Let’s delve into practical examples that encapsulate these priorities:
- Data ethics and responsibility
- Augmented data management
- Real-time data processing
- DataOps methodology adoption
- Ensuring data privacy and compliance
- Cloud-native data infrastructure
- Expanding data literacy
- Graph analytics and databases
- Quantum computing for data
- Enhancing data visualization
Let’s explore each example in detail.
1. Data ethics and responsibility #
Cambridge Analytica Scandal: The misuse of Facebook user data for political advertising showcased the paramount importance of using data ethically and responsibly. This incident underscores the need for stringent ethical standards in data collection and utilization.
2. Augmented data management #
Netflix’s Content Recommendation: Netflix leverages AI-driven algorithms to automate the curation and recommendation of content for its users. This requires managing vast amounts of user and content data, augmented by AI, to ensure viewers see shows and movies tailored to their tastes.
3. Real-time data processing #
Uber’s Dynamic Pricing: Uber’s surge pricing is determined through real-time analysis of demand and supply in an area. As conditions change, data needs to be processed instantly to adjust prices accordingly.
4. DataOps methodology adoption #
DevOps Integration at Capital One: The banking firm Capital One integrated DevOps into their data operations to streamline workflows, improve collaboration, and increase the speed and reliability of data analytics.
5. Ensuring data privacy and compliance #
GDPR and Tech Companies: The introduction of the General Data Protection Regulation (GDPR) in the EU required companies like Google and Apple to revamp their data collection and storage practices. These companies had to ensure transparency, obtain user consent, and provide data portability and deletion options.
6. Cloud-native data infrastructure #
Snapchat’s Multi-cloud Strategy: Snapchat uses a multi-cloud strategy with Google Cloud and AWS to handle its massive data storage and processing needs, ensuring both scalability and reliability.
7. Expanding data literacy #
Airbnb’s Data University: Recognizing the importance of data literacy across the organization, Airbnb created “Data University”, an internal initiative offering various courses to educate their staff about data and analytics.
8. Graph analytics and databases #
LinkedIn’s People You May Know: LinkedIn’s feature, “People You May Know,” uses graph databases to sift through connections and suggest potential contacts, understanding and leveraging the intricate relationships between users.
9. Quantum computing for data #
IBM’s Quantum Experience: IBM offers cloud-based quantum computing services that allow researchers and businesses to experiment with quantum algorithms, anticipating a future where quantum computing will revolutionize data processing.
10. Enhancing data visualization #
Johns Hopkins University’s COVID-19 Dashboard: In response to the pandemic, Johns Hopkins University developed a real-time interactive dashboard that visualizes COVID-19 cases globally. The dashboard enabled public health officials, researchers, and the general public to track the spread and impact of the virus efficiently.
In examining these real-world instances, it’s evident that the priorities of data teams aren’t just theoretical constructs. They play a vital role in shaping how businesses operate, how consumers interact with services, and even how global events are understood and managed. The alignment of data practices with these priorities can have profound implications in the real world.
Bottom line? #
Understanding data teams’ 2024 priorities empowers leveraging data ethically, embracing AI-augmented management, real-time insights, and a DataOps approach, while ensuring privacy, cloud-native infrastructure, and fostering data literacy, all while exploring the quantum frontier and enhancing visualization.
These priorities materialize in real-world scenarios, from Netflix’s personalized content recommendations to LinkedIn’s graph-powered connections and IBM’s quantum advancements, underscoring data teams’ role in shaping ethics, efficiency, and understanding across industries.
By navigating these priorities, data teams navigate a landscape where data’s power is harnessed responsibly and innovatively.
The top 10 priorities of data teams: Related reads #
- Modern data team 101: A roster of diverse talent
- Modern data culture: The open secret to great data teams
- What is modern data stack: History, components, platforms, and the future
- What is a modern data platform: Components, capabilities, and tools
- Modern data catalogs: What They Are, How They’ve Changed, Where They’re Going
- Data Governance Committee 101: When Do You Need One?
- How to Become a Data Governance Expert: 2024 Roadmap
Share this article