The G2 Grid® Report for Machine Learning Data Catalog: How Can You Use It to Choose the Right Data Catalog for Your Organization?
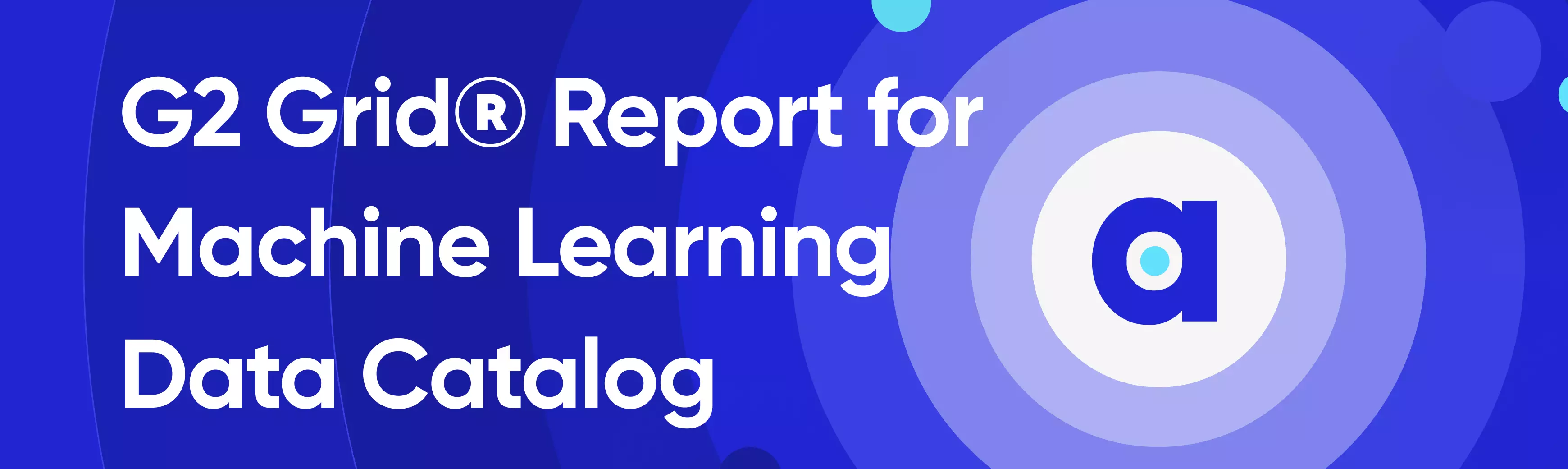
Share this article
The G2 Grid® Report for Machine Learning Data Catalog is a good starting point for you to compare the various machine learning data catalogs available.
The framework considers several data points from G2 user reviews to third-party data sources to evaluate the most popular machine learning data catalogs. Let’s look at the framework and evaluating criteria to know more.
Table of Contents #
- What is the G2 Grid® Report for Machine Learning Data Catalog?
- How is the G2 Grid® Report calculated?
- How to interpret the G2 Grid® Report for Machine Learning Data Catalog
- Most important features to consider when researching machine learning data catalogs
- Wrapping up
- G2 Machine learning data catalog: Related reads
What is the G2 Grid® Report for Machine Learning Data Catalog? #
The G2 Grid® Report for Machine Learning Data Catalog is a framework to evaluate ML data catalogs (i.e., MLDCs). The grid uses several factors, such as market presence, customer satisfaction scores, user adoption percentage, and ROI to assess machine learning data catalogs.
You can use the G2 Grid® to:
- Compare the various machine learning data cataloging products
- Streamline your buying process for the data catalog
- Identify the best products for your organization quickly, based on the experiences of your peers
How is the G2 Grid® Report calculated? #
G2 looks at user reviews, publicly available information, and third-party sources to prepare a comprehensive assessment of each product listed in its grid.
According to G2:
“G2 scores products and vendors based on reviews gathered from our user community, as well as data aggregated from online sources and social networks. Together, these scores are mapped on our proprietary G2 Grid®.”
While the list isn’t exhaustive, here are some of the core factors that G2 category Grid® Reports use to compare products in any given category:
- Satisfaction score aggregates the scores for a product’s category, with core categories receiving greater weight than supplementary categories.
- Market presence score measuring the product’s market share, vendor size, and influence in the machine learning data catalog category
- Feature level data (if available)
- User adoption percentage
- ROI
- Available segmented
- A product’s implementation score by looking at factors, such as:
- Time required to go live
- Implementation method used
- Customer satisfaction with the set-up process
- A product’s results score by considering the customers’ responses about factors, such as:
- Reported time to go live
- Customer satisfaction with the product’s ability to meet their requirements
- The likelihood to recommend a product
- A product’s usability data by measuring factors, such as:
- Ease of use
- Ease of admin
- Customer responses to the “Meets Requirements” question
- A product’s relationship score by looking at customer satisfaction in terms of:
- Ease of doing business
- Quality of support
- The response to the “Likely to Recommend” question
How to interpret the G2 Grid® Report for Machine Learning Data Catalog #
While the G2 category Grid® Reports consider several factors to rank various products, the grid itself is mapped with two axes, namely market presence and customer satisfaction.
The grid is further divided into four quadrants:
- Leaders: Well-established tools with a high market presence and customer satisfaction
- High Performers: Show good potential but have a lower market presence
- Contenders: Traditional tools with a high market presence but low customer satisfaction
- Niche: Less well-known tools with limited functionality
G2 Grid® Report for Machine Learning Data Catalog Source: G2.
Let’s understand each quadrant of the grid further.
G2 Grid® for Machine Learning Data Catalogs: The Leaders quadrant #
Tools in the Leaders quadrant, like Atlan, have high customer satisfaction ratings and a high market presence. These tools are considered to be the most comprehensive and mature for ML data cataloging.
They’re ideal for large enterprises with complex data environments requiring extensive data discovery, metadata management, and data governance capabilities.
G2 Grid® for Machine Learning Data Catalogs: The High Performers quadrant #
The tools in the High Performers quadrant, like Coginiti, have high customer satisfaction ratings but a lower market presence. These tools are relatively new to the market but still offer powerful ML data cataloging capabilities.
They’re ideal for smaller businesses with significantly less complex data environments, as compared to large enterprises. These tools might not offer the same amount of features as the tools in the Leaders quadrant as their use cases might still be evolving.
G2 Grid® for Machine Learning Data Catalogs: The Contenders quadrant #
The tools in the Contenders quadrant, like Collibra, have a high market presence but lower customer satisfaction ratings.
While these tools may offer mature capabilities for ML data cataloging and have an established market presence, they may not be easy to use and don’t offer extensive customer support.
Their popularity comes from legacy customers as these tools have been around for a while. However, they struggle to keep up with modern, intuitive, and cloud-agnostic ML data catalogs like Atlan.
G2 Grid® for Machine Learning Data Catalogs: The Niche quadrant #
Tools in the Niche quadrant, like Datalogz, have both low customer satisfaction ratings and a lower market presence.
These tools are either too new to the market or cater to a specific set of use cases. They’re also limited in their capabilities when compared with the more mature tools in the Leaders or High Performers quadrant.
They may be ideal for small teams requiring compact ML data catalogs to solve very specific problems in data discovery.
The most important features that data practitioners are considering when researching machine learning data catalogs #
As mentioned earlier, the G2 Grid® Report analyzes several capabilities to rank ML data catalogs. These include:
- Metadata ingestion with native connectors: Machine learning data catalogs should offer native connectors to compile data from various data sources, BI platforms, applications, and other data science tools in your tech stack.
- Data discovery: You should be able to look for data assets and discover new insights across your entire data asset universe from a single platform.
- Semantic search: According to G2, the machine learning data catalog should provide you with visual and intuitive searches like search engines to look for databases, SQL queries, BI dashboards, etc.You should also be able to browse for the asset you want and filter your search results with metadata properties, such as certification, owners, run status, and more.
- Auto-classification of data assets: G2 emphasizes that automated labeling of data assets is a prerequisite for machine learning algorithms. Machine learning data catalogs should auto-classify data assets to eliminate manual efforts and reduce the risk of human errors.
- 360-degree data profiles: MLDCs should help you get complete context on the asset’s glossary, schema, relationships, usage popularity, and more at a glance. You can think of it as LinkedIn profiles but for data assets.
- Metadata management: The ML data catalog should offer active metadata management to help you connect all the tools in your data stack and ferry metadata back and forth in a two-way stream.This is essential to set up a living, intelligent, action-oriented data ecosystem that optimizes costs, enhances data discovery, enriches data quality, and supports data security and compliance. Also, read → Active metadata use cases
- Automated data lineage: MLDCs should help you automate the lineage mapping process down to a column level so that you can see how data flows through your organization.This requires capabilities such as automated SQL parsing, out-of-the-box integrations with data warehouses, lakes, and BI tools, and open APIs to connect with any data product you want.
- Compliance capabilities: The machine learning data catalog should help you ensure that sensitive data doesn’t get exposed. It can offer capabilities such as auto-classification of sensitive assets or masking policy propagation via data lineage for upstream and downstream applications.
Wrapping up #
The G2 Grid® for Machine Learning Data Catalog covers several data catalogs that will help you automate various aspects of data discovery, classification, profiling, lineage, and governance.
Besides looking at the factors mentioned above, G2 recommends that you pick a tool that offers the following benefits:
- Ease in data and metadata curation
- Ease of search
- Ease of collaboration
So, it’s best to consider machine learning data catalogs (MLDCs) that meet all of your requirements and offer the above benefits.
Also, read → 15 Essential Data Catalog Features to Look For in 2023
G2 Machine learning data catalog: Related reads #
- Data Catalog: The Must-Have Tool for Data Leaders in 2023
- AI Data Catalog: Exploring the Possibilities That Artificial Intelligence Brings to Your Metadata Applications & Data Interactions
- Machine Learning Data Catalogs: Evolution, Benefits, Business Value and Uses in 2023
- 8 Ways AI-Powered Data Catalogs Save Time Spent on Documentation, Tagging, Querying & More
- 15 Essential Data Catalog Features to Look For in 2023
- Top Data Catalog Use Cases Intrinsic to Data-Led Enterprises
- Business Data Catalog: Users, Differentiating Features, Evolution & More
Share this article